Help Wanted
An Examination of Hiring Algorithms, Equity, and Bias
Aaron Rieke and Miranda Bogen
ReportExecutive Summary
The hiring process is a critical gateway to economic opportunity, determining who can access consistent work to support themselves and their families. Employers have long used digital technology to manage their hiring decisions, and now many are turning to new predictive hiring tools to inform each step of their hiring process.
This report explores how predictive tools affect equity throughout the entire hiring process. We explore popular tools that many employers currently use, and offer recommendations for further scrutiny and reflection. We conclude that without active measures to mitigate them, bias will arise in predictive hiring tools by default.
Key Reflections:
Hiring is rarely a single decision point, but rather a cumulative series of small decisions. Predictive technologies can play very different roles throughout the hiring funnel, from determining who sees job advertisements, to estimating an applicant’s performance, to forecasting a candidate’s salary requirements.
While new hiring tools rarely make affirmative hiring decisions, they often automate rejections. Much of this activity happens early in the hiring process, when job opportunities are automatically surfaced to some people and withheld from others, or when candidates are deemed by a predictive system not to meet the minimum or desired qualifications needed to move further in the application process.
Predictive hiring tools can reflect institutional and systemic biases, and removing sensitive characteristics is not a solution. Predictions based on past hiring decisions and evaluations can both reveal and reproduce patterns of inequity at all stages of the hiring process, even when tools explicitly ignore race, gender, age, and other protected attributes.
Nevertheless, vendors’ claim that technology can reduce interpersonal bias should not be ignored. Bias against people of color, women, and other underrepresented groups has long plagued hiring, but with more deliberation, transparency, and oversight, some new hiring technologies might be poised to help improve on this poor baseline.
Even before people apply for jobs, predictive technology plays a powerful role in determining who learns of open positions. Employers and vendors are using sourcing tools, like digital advertising and personalized job boards, to proactively shape their applicant pools. These technologies are outpacing regulatory guidance, and are exceedingly difficult to study from the outside.
Hiring tools that assess, score, and rank jobseekers can overstate marginal or unimportant distinctions between similarly qualified candidates. In particular, rank-ordered lists and numerical scores may influence recruiters more than we realize, and not enough is known about how human recruiters act on predictive tools’ guidance.
Recommendations:
Vendors and employers must be dramatically more transparent about the predictive tools they build and use, and must allow independent auditing of those tools. Employers should disclose information about the vendors and predictive features that play a role in their hiring processes. Vendors should take active steps to detect and remove bias in their tools. They should also provide detailed explanations about these steps, and allow for independent evaluation.
The EEOC should begin to consider new regulations that interpret Title VII in light of predictive hiring tools. At a bare minimum, the agency should issue a report that further explores these issues, including a candid reflection on the capacity of current regulatory guidance to account for modern hiring technologies.
Regulators, researchers, and industrial-organizational psychologists should revisit the meaning of “validation” in light of predictive hiring tools. In particular, the value of correlation as a signal of “validity” for antidiscrimination purposes should be vigorously debated.
Digital sourcing platforms must recognize their growing influence on the hiring process and actively seek to mitigate bias. Ad platforms and job boards that rely on dynamic, automated systems should be further scrutinized–both by the companies themselves, and by outside stakeholders.
Introduction
The hiring process is a critical gateway to economic opportunity, determining who can access consistent work to support themselves and their families. Employers have long used digital technology to manage their hiring decisions, and now many are turning to new predictive hiring tools to inform each step of their hiring process.
Today, employers like Target, Hilton, Cisco, PepsiCo, Amazon, and Ikea, along with major staffing agencies, are testing and adopting data-driven, predictive tools. With increasing public attention on “artificial intelligence” and emerging popularity of the technology in the employment context, these tools are simultaneously touted for their potential to reduce bias in hiring and vigorously derided for their capacity to exacerbate it. As predictive technologies continue to proliferate throughout the hiring process—for both low-wage, low-skilled jobs and higher wage, white collar positions—it is critical to understand what types of tools are currently being used and how they work, as well as how they may advance or reduce equity.
Hiring is rarely a single decision—it is a series of smaller, sequential decisions.
Hiring is rarely a single decision, but rather a series of smaller, sequential decisions that culminate in a job offer—or a rejection. Hiring technologies can play very different roles throughout this process. For example, in the early stages of recruiting, automated predictions can steer job advertisements and personalized job recommendations to jobseekers from particular demographic groups. Once candidates have applied, algorithms help recruiters assess and quickly disqualify candidates, or prioritize them for further review. Some tools engage candidates with chatbots and virtual interviews, and others use game-based assessments to reduce reliance on traditional (and often structurally biased) factors like university attendance, GPA, and test scores. At each stage, predictive technologies can have a powerful effect on who ultimately succeeds in the hiring process.
“In the case of systems meant to automate candidate search and hiring, we need to ask ourselves: What assumptions about worth, ability and potential do these systems reflect and reproduce? Who was at the table when these assumptions were encoded?”
Meredith Whittaker, Executive Director, AI Now Institute
This report explores how predictive tools are integrated throughout the hiring process. These tools are commonly referred to as “hiring algorithms,” or “artificial intelligence,” but we have chosen to use the frame of “prediction” to remove needless complexity and mystique. Simply put, predictive tools aim to forecast outcomes and behavior by analyzing existing data.
In preparing this report, we attended industry conferences to learn how hiring professionals understand their own work, and how talent acquisition technology vendors frame their offerings. We reviewed technical and interdisciplinary research to situate modern hiring tools within the evolving landscape of both the hiring industry and artificial intelligence technologies. We studied the features, technical specifics, and interfaces of key predictive hiring products. Finally, we closely analyzed vendors’ marketing and research materials, public statements and presentations, and product documentation.
In the first part of this report, we summarize some important background and key concepts: the history of hiring technologies since the 1990s, incentives driving employers to adopt hiring technologies, a conceptual framework for assessing equity (especially those beyond interpersonal biases), and basic U.S. legal and regulatory context. Next, we outline the four stages of the classic hiring process: sourcing, screening, interviewing, and selection. We explore popular predictive technologies used at each stage, analyzing their promises and pitfalls. In closing, we offer reflections and recommendations.
Key Concepts
This section offers background and concepts needed to fully engage with the remainder of this report. First, we outline the evolution of hiring technologies since the advent of the internet, describe how the machine learning techniques used by many of today’s predictive tools work, and identify the primary reasons employers adopt new technologies. Next, we articulate several different kinds of social bias, and explain common ways that predictive tools can absorb and compound them. Finally, we briefly summarize relevant U.S. law and policy, highlighting areas of ambiguity.
Technology: From Monster.com to Machine Learning
A History of Hiring Technology
Hiring technology has evolved rapidly alongside the internet. As early as the 1990s, online job boards like Monster.com capitalized on the new medium by offering employers digital job listings at rates well below those of newspaper classified ads. Search engines for these online job postings emerged soon after, and pay-per-click advertising helped recruiters compete for attention in a newly crowded online market.
Next came new ways to apply for jobs over the internet, triggering a jump in the volume of applications for open positions as it became easier to apply for multiple jobs. The resulting deluge of applicants–many of whom lacked employers’ desired qualifications–prompted employers to adopt applicant tracking systems to help both organize and evaluate rapidly growing pools of candidates.
Meanwhile, recruiters began using digital technology to proactively seek out desirable applicants. By scouring new, public sources of information (like professional profiles and work samples on emerging platforms like LinkedIn), recruiters were able to broaden their focus from “active” candidates–those proactively exploring or applying to open roles–to “passive” ones, who had desirable qualifications but no apparent intention to switch jobs.
As the quantity of potential job candidates ballooned further to include both higher volumes of active applicants as well as millions of passive ones, some employers began turning to new screening tools to keep up. While employers had long relied on tests and assessments to screen jobseekers, the development of new techniques to collect and analyze data prompted the introduction of more advanced assessments.
In response to the growing push for diversity and inclusion (D&I) in the workplace, some technology vendors have more recently introduced tools to facilitate diversity recruiting and reduce various biases endemic to the hiring process. Some vendors offer entire products geared primarily or exclusively for diversity recruiting, while others incorporate features catering to those goals.
Today, hiring technology vendors increasingly build predictive features into tools that are used throughout the hiring process. They rely on machine learning techniques, where computers detect patterns in existing data (called training data) to build models that forecast future outcomes in the form of different kinds of scores and rankings. This new wave of hiring technology resembles popular consumer services like Google’s search engine, Netflix’s personalized movie recommendations, and Amazon’s Alexa assistant, as well as advanced marketing and sales tools like Salesforce.
Why Employers Adopt Predictive Tools
Employers turn to hiring technology to increase efficiency, and in hopes that they will find more successful–and sometimes, more diverse–employees. For many employers, such tools are a basic part of doing business in the digital age. Understanding employers’ motivations to adopt these tools is helpful to make sense of the context in which they are used.
Most employers want to reduce time to hire, the amount of time it takes to fill an open position. It takes a typical U.S. employer six weeks to fill a role, and the longer it takes to find a suitable candidate, the more time and resources are diverted from other priorities. A slow hiring process might lead to a poor applicant experience and increase the likelihood that candidates will drop out of the hiring process or share their bad experience with friends. Employers also fear losing candidates to their competitors–a particularly acute concern in a tight job market. Moreover, some companies have seasonal staffing needs that make it critical to hire new employees within a particular time frame.
Employers also want to reduce cost per hire, or the marginal cost of adding a new worker, which is roughly $4,000 in the U.S. According to research from LinkedIn, 35 percent of companies feel significantly constrained by limited recruitment budgets, and most don’t expect an improvement in the coming year, even as many anticipate an increase in hiring volume.
Employers also try to maximize quality of hire, which is judged based on metrics related to performance evaluations, the quantity or quality of worker output, or whether the hire was eventually promoted or disciplined. Inversely, employers might also aim to avoid hiring “toxic” employees, to prevent theft, or even to forestall labor organizing activities. Many employers also look to maximize the tenure of their workers, presuming that “successful” hires will stay longer than less successful ones. Long tenure is seen as a simple, quantifiable signal of a high-quality hire, while brief tenure can be interpreted as the sign of a “bad fit.” Turnover is costly, requiring an employer to hire and train new workers.
Finally, some employers have goals for workplace diversity, based on gender, race, age, religion, disability, or veteran or socioeconomic status. They may be drawn toward hiring tools that purport to help avoid discriminating against applicants in protected categories, or that appear poised to proactively diversify their workforce. Hiring vendors of all stripes claim they can help employers achieve these goals.
Equity: Beyond Interpersonal Bias
Hiring tool vendors often tout technology’s potential to remove bias from the hiring process. They argue that by making hiring more consistent and efficient, recruiters will be empowered to make fairer and more holistic hiring decisions, or that their tools will naturally reduce bias by obscuring applicants’ sensitive characteristics. But, as we explain below, vendors are usually referring to interpersonal human prejudice, which is only one source of bias. Institutional, structural, and other forms of bias are just as important, if not more important, aspects of any equity analysis when it comes to employment.
Different Dimensions of Bias
In common parlance, the term “bias” is often used to refer to interpersonal bias–prejudices held by individual people, whether implicitly or explicitly. Interpersonal bias against people of color, women, and other marginalized groups has long plagued the hiring process. To this day, many hiring managers evaluate candidates in ways that contribute to disparate hiring outcomes, leading to underrepresentation and pay disparities in roles across industries. But other, more structural kinds of bias also act as barriers to opportunity for jobseekers, especially when predictive tools are involved.
Institutional, structural, and other forms of bias are critical aspects of any equity analysis, especially when it comes to employment.
Bias arises at the institutional level when policies and workplace cultures serve to benefit certain workers and disadvantage others. For example, a business that rewards men for acting ambitiously but punishes women for the same behavior will lead to situations where men are seen as more successful employees. Likewise, a company that tends to hire from a privileged and homogeneous community and then uses “culture fit” as a factor in hiring decisions could end up methodically rejecting otherwise qualified candidates who come from more diverse backgrounds.
Hiring practices can also perpetuate systemic (or “structural”) biases: patterns of disadvantage stemming from contemporary and historical legacies such as racism, unequal economic opportunity, and segregation. For example, many white collar employers place a high value on elite university attendance, but despite changing admissions policies, such a credential is still disproportionately attained by privileged individuals, and often out of reach for those who lack access to quality primary and secondary education. Without proactive steps to account for these realities, even seemingly objective hiring criteria like one’s alma mater or test performance can end up reflecting systemic biases.
Biases can also be internalized by jobseekers themselves, influencing their own behaviors, such as whether or not to apply for a given job. Moreover, within and across all of these categories, the intersection of multiple identities can compound disadvantage in ways that are often overlooked. For instance, a black woman jobseeker may be judged more harshly than other women because of her race, while at the same time find it harder to access opportunities than black men because of gender-based discrimination. The treatment of intersectionality in employment law is far from settled, and their manifestation in the digital realm is only beginning to be studied.
How Predictive Tools Can Perpetuate Biases
The types of bias described above can exist and emerge in predictive hiring tools in several distinct ways.
First, when the training data for a model is itself inaccurate, unrepresentative, or otherwise biased, the resulting model and the predictions it makes could reflect these flaws in a way that drives inequitable outcomes. For example, an employer, with the help of a third-party vendor, might select a group of employees who meet some definition of success–for instance, those who “outperformed” their peers on the job. If the employer’s performance evaluations were themselves biased, favoring men, then the resulting model might predict that men are more likely to be high performers than women, or make more errors when evaluating women. This is not theoretical: One resume screening company found that its model had identified having the name “Jared” and playing high school lacrosse as strong signals of success, even though those features clearly had no causal link to job performance.
Predictive models can reflect biases in other subtle and powerful ways, which can be difficult to detect and correct. For example, in one well-known case, an employer who wanted to maximize worker tenure found that distance from work was the single most important variable that determined how long workers stayed with the employer–but it was also a factor that strongly correlated with race. Since many social patterns related to education and work reflect troubled legacies of racism, sexism, and other forms of socioeconomic disadvantage, blindly replicating those patterns via software will only perpetuate and exacerbate historical disparities. These patterns can also emerge as tools are used, particularly when models are built to learn and adapt to the preferences of its users over time. Importantly, removing or obscuring sensitive factors like gender and race will not prevent predictive models from reflecting patterns of bias.
Removing or obscuring sensitive factors like gender and race will not prevent predictive models from reflecting patterns of bias.
Second, people can be unduly influenced by computerized recommendations. Separate from the mechanics of prediction itself, predictive hiring tools can create new opportunities for cognitive bias as they display information to human recruiters. A phenomenon known as automation bias occurs when people “give undue weight to the information coming through their monitors.” When predictions, numerical scores, or rankings are presented as precise and objective, recruiters may give them more weight than they truly warrant, or more deference than a vendor intended. Moreover, when tools reveal job candidates’ pictures or other demographic features, these interfaces could also subconsciously affect recruiters’ decisions.
A variety of other equity concerns can also be implicated by the technical design and interface of hiring software. For one, candidates with limited internet access or skills, or those with disabilities, may face distinct challenges using online job platforms, which can in turn influence a system’s judgement of their suitability and lead to further exclusion. Additionally, the collection, structure, and labeling of underlying data can impose rigid or exclusionary definitions of identity. For instance, tools that classify applicants into “male” and “female” categories–even for the affirmative purpose of monitoring for gender equality–could end up marginalizing queer, transgender, and non-binary people, while tools that classify people by race reify political categories that “by their very nature mark a status inequality.”
Without active measures to mitigate them, biases will arise in predictive hiring tools by default.
Without active measures to mitigate them, biases will arise in predictive hiring tools by default. But predictive tools could also be turned in the other direction, offering employers the opportunity to look inward and adjust their own past behavior and assumptions. This insight could also help inform data and design choices for digital hiring tools that ensure they promote diversity and equity goals, rather than detract from them. Armed with a deeper understanding of the forces that may have shaped prior hiring decisions, new technologies, coupled with affirmative techniques to break entrenched patterns, could make employers more effective allies in promoting equity at scale.
Law and Policy: Antidiscrimination and Ambiguities
This section offers a brief overview of key U.S. laws and regulations related to discrimination in hiring. The most pertinent law, Title VII of the Civil Rights Act of 1964, broadly prohibits hiring discrimination by employers and employment agencies on the basis of certain protected characteristics. But there are ambiguities about how this law applies to predictive hiring technology. A range of other state and federal laws and rules are also relevant to assessing and overseeing predictive hiring tools.
Key U.S. Statutes and Regulations
Title VII of the Civil Rights Act of 1964 forbids employers from discriminating on the basis of race, color, religion, sex, and national origin. The law seeks to “achieve equality of employment opportunities and remove barriers that have operated in the past to favor … white employees over other employees.” Its provisions extend broadly to advertising, hiring, compensation, terms, conditions, and privileges of employment. Other federal legislation has extended similar protections to older people and people with disabilities.
More specifically, Title VII makes it unlawful for employers and employment agencies to “limit, segregate, or classify … employees or applicants for employment in any way which would deprive or tend to deprive any individual of employment opportunities or otherwise adversely affect [^them]” because of their protected class status. Title VII is conventionally understood to prohibit two kinds of discrimination: disparate treatment and disparate impact. Disparate treatment cases involve overt discrimination, whereas disparate impact covers employment practices that are facially neutral but have a discriminatory effect.
Because disparate impact is often the theory invoked to address harms brought about by predictive tools, the mechanics of a disparate impact case deserve further explanation. To prevail in a disparate impact case, a complainant must first make some showing that an employment practice has a disparate impact on the basis of a protected characteristic. Next, an employer can counter by showing a valid “business necessity”–for example, some amount of evidence that the practice was “job-related,” or that it accurately measured an applicant’s ability to perform on the job. If the employer is successful in making its case, the complainant then must show the existence of a “less discriminatory alternative,” such as another kind of test or procedure that would serve the employer’s legitimate interest while having less of a harmful effect on protected groups.
The Equal Employment Opportunity Commission (EEOC) is the federal agency charged with enforcing federal laws related to employment discrimination. In practice, the EEOC does not typically investigate discrimination except when an individual makes a specific complaint. After such a complaint has been filed, the EEOC can open an investigation, and has a broad right to access relevant evidence. The EEOC also periodically issues guidance and regulations, incorporating input from public meetings, discussion, and comments.
Additional legal and regulatory requirements apply to federal contractors, companies and organizations that provide services or products to a government agency, including healthcare providers, universities, technology companies, hotels, and airlines. Such contractors employ a significant portion of the U.S. workforce. These requirements are overseen by the Office of Federal Contract Compliance Programs (OFCCP).For example, Executive Order 11246 requires that most government contractors take “affirmative action” to ensure that equal opportunity is provided in all aspects of their employment, including recruiting–a requirement that goes beyond the basic requirements of Title VII. Contractors are also required to solicit the race, gender, and ethnicity of job applicants, including “internet applicants,” to enable regulatory research and enforcement.
Finally, a range of other federal, state and local laws are relevant to predictive hiring tools. Laws like the Genetic Information Nondiscrimination Act of 2008 anticipated the risk of employers turning to newly available–and highly sensitive–sources of data to inform hiring decisions. Some cities and states have expanded protections to characteristics not explicitly covered by Title VII, like gender identity, sexual orientation, citizenship status, and political affiliation. Equal pay and salary history laws promote equitable compensation.In other countries, particularly in Europe, data protection laws like the General Data Protection Regulation (GDPR) play a significant role in determining what information and data processing techniques employers can use during the course of their recruitment activities.
Gaps and Ambiguities
The laws and regulations described above may not always apply to predictive technologies. First, it is not obvious that hiring technology vendors are themselves covered by Title VII. The statute does cover employment agencies–entities that “procure employees for an employer”–but many vendors would argue they merely provide products and services to employers and ought not be liable for employers’ ultimate use. Second, while Title VII covers employment advertising and applicant sourcing, the EEOC has offered “only minimal guidance in this area,” and only a handful of legal cases have considered these statutory provisions. However, courts have found that advertising campaigns can trigger disparate impact liability, and have been willing to analyze the broader context of an employer’s recruitment ad campaign, not just an ad’s content.
Current interpretations of the disparate impact doctrine are ill-suited to address bias that arises in machine learning models.
Importantly, current interpretations of the disparate impact doctrine are ill- suited to address bias that arises in machine learning models. For example, the EEOC’s Uniform Guidelines on Employee Selection Procedures, which have not been updated since their enactment in 1978, interpret Title VII to provide a “framework for determining the proper use of tests and other selection procedures.” The framework relies heavily on the notion of “validity studies” to demonstrate that a procedure is sufficiently related to or “significantly correlated with important elements of job performance.” Unfortunately, showing correlation does little to help assess whether a machine learning model is surfacing biases or not. Critics have called this kind of validity analysis “largely ill equipped” and “simply irrelevant” to assessing discrimination in the modern world of data mining.
For those discrimination claims that do end up in court, technology vendors may succeed in shielding themselves from close scrutiny.
Finally, investigation and enforcement under existing legal frameworks require complainants and regulators to be able to notice and bring about claims of machine-enabled discrimination, and to have the resources and ability to investigate and contest them. At present, many jobseekers may not realize they have been judged by a predictive technology, and even if they do, may not have sufficient access to the tool to describe its impact (or the resources to retain expert witnesses to do so), let alone propose a less discriminatory alternative. The EEOC is under-resourced, yet saddled with a long backlog of complaints, and so has little capacity to take on more complex investigations. For discrimination claims that do end up in court, technology vendors may succeed in shielding themselves from close scrutiny through trade secrecy and intermediary immunity claims, which have so far proven difficult to pierce even in cases where key rights and due process appear to have been undermined.
Predictive Tools Across the Hiring Funnel
Hiring is rarely a single decision, but rather a funnel: a series of decisions that culminate in a job offer or a rejection. The hiring process starts well before anyone submits an actual job application, and jobseekers can be disadvantaged or rejected at any stage. Importantly, while new hiring tools rarely make affirmative hiring decisions, they often automate rejections.
While predictive hiring tools rarely make affirmative hiring decisions, they often automate rejections.
Employers start by sourcing candidates, attracting potential candidates to apply for open positions through advertisements, job postings, and individual outreach. Next, during the screening stage, employers assess candidates—both before and after those candidates apply—by analyzing their experience, skills, and characteristics. Through interviewing applicants, employers continue their assessment in a more direct, individualized fashion. During the selection step, employers make final hiring and compensation determinations.
The Hiring Funnel
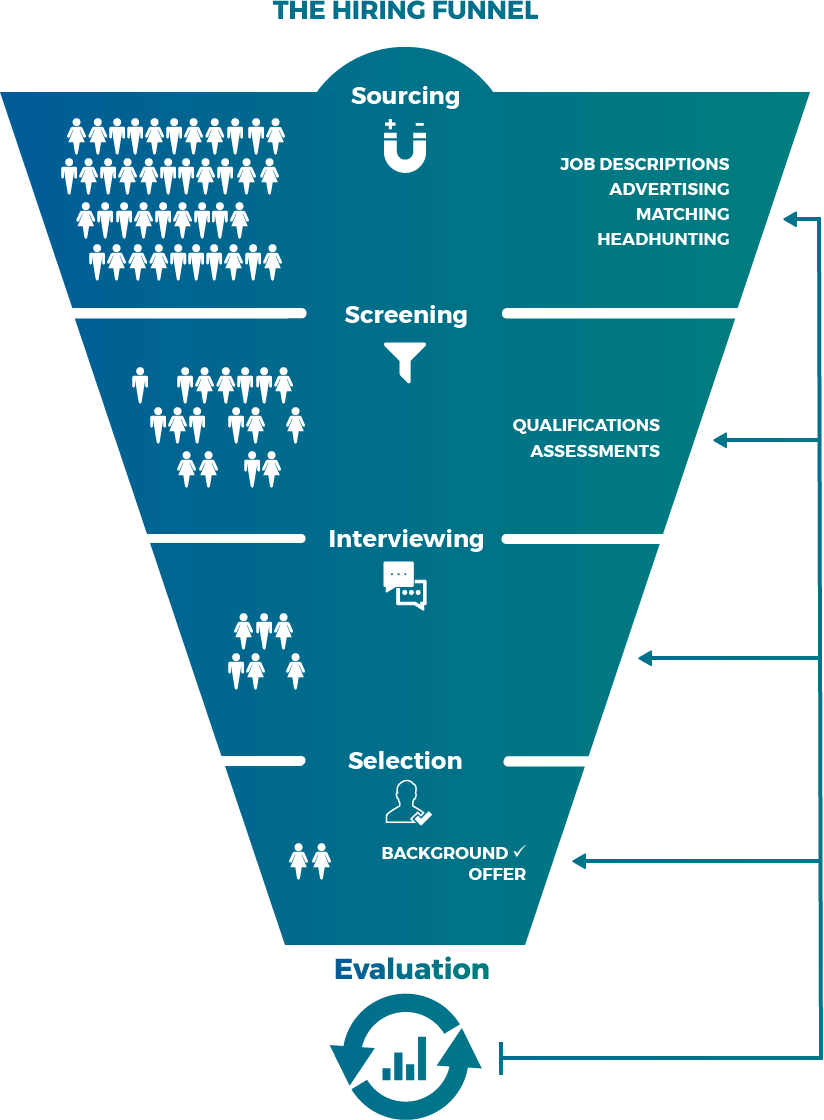
Below, we explore how new predictive hiring tools are being used in each stage, describing and analyzing illustrative products on the market today. Not all products fit cleanly within one stage—some perform multiple roles behind a single interface, or blur the lines between previously distinct stages. After each description, we offer a brief equity analysis.
We do not attempt to map which employers are using which products. This is because employers can use multiple recruitment tools, often from third party vendors, to manage their hiring activities. Many of these tools can integrate with each other, making it easy for employers to mix and match products behind the scenes. In practice, while it is often obvious what primary applicant tracking system an employer uses (because it is usually visible when exploring a company’s job application portal), it can be nearly impossible to tell from the outside what additional tools—or customizations of those tools—an employer may be using to manage and assess applicants. Employers can even use different tools to assess applicants for different positions within the same firm, which would not be obvious unless someone applied to a variety of roles.
For these reasons, we can’t definitively say which tools are more commonly used to recruit for low-income jobs or service sector jobs, as compared to white collar positions. However, generally speaking, employers’ technology choices seem influenced at least as much by an employer’s size as by differences in job function or industry.
It is important to also note that the marketplace for hiring tools is extremely dynamic. Startups and emerging companies frequently launch new products, acquire one another, or are subsumed into enterprise human resource software companies. As a result, details about particular tools can quickly become outdated.
Recognizing this, we encourage the reader to treat the examples below as archetypes to help inform future investigation and analysis. These products were selected primarily for their capacity to exemplify notable and relevant features.
A Landscape of Predictive Hiring Tools
Sourcing
In the sourcing stage, employers seek out candidates to apply for their job opportunities. Predictive technologies help place and optimize job advertisements, notify jobseekers about potentially appealing positions, and identify candidates who may be poachable from a competitor or who may be enticed to rejoin the job market. Sourcing technologies can shape a candidate pool—for better or for worse—before applications ever change hands.
Job Descriptions
Almost every job opening starts with a job description—the title, framing, requirements, and specific wording used to describe a job opportunity. Job descriptions can powerfully influence who chooses to apply for a position. For example, research has found that job descriptions that rely on stereotypically male words tend to result in fewer female applicants.
One vendor called Textio offers tools to help employers adjust the text of their job descriptions to attract more applicants, and to promote more diverse applicant pools, particularly along gender lines.
Textio works by comparing linguistic patterns in the text of a job posting with historical applicant behavior and hiring outcomes, in order to predict the approximate size and demographics of the expected candidate pool. The tool assigns each job posting an overall score between 0 and 100, reflecting a prediction of how quickly a listing will fill compared to jobs in the same industry and location.
A separate “gender tone meter” claims to measure the extent to which language in the job description risks alienating applicants of either gender. This measure predicts the gender balance of applicants, given the proposed text.
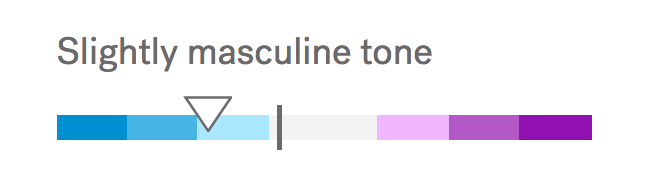
Textio’s gender tone meter
Textio also assesses specific strengths and weaknesses of the job description (like length, complexity, and word choice) and suggests wording changes that would raise its score or improve its gender tone. As employers follow Textio’s suggestions, they can see how those changes could influence both the overall and gender tone scores in real time.
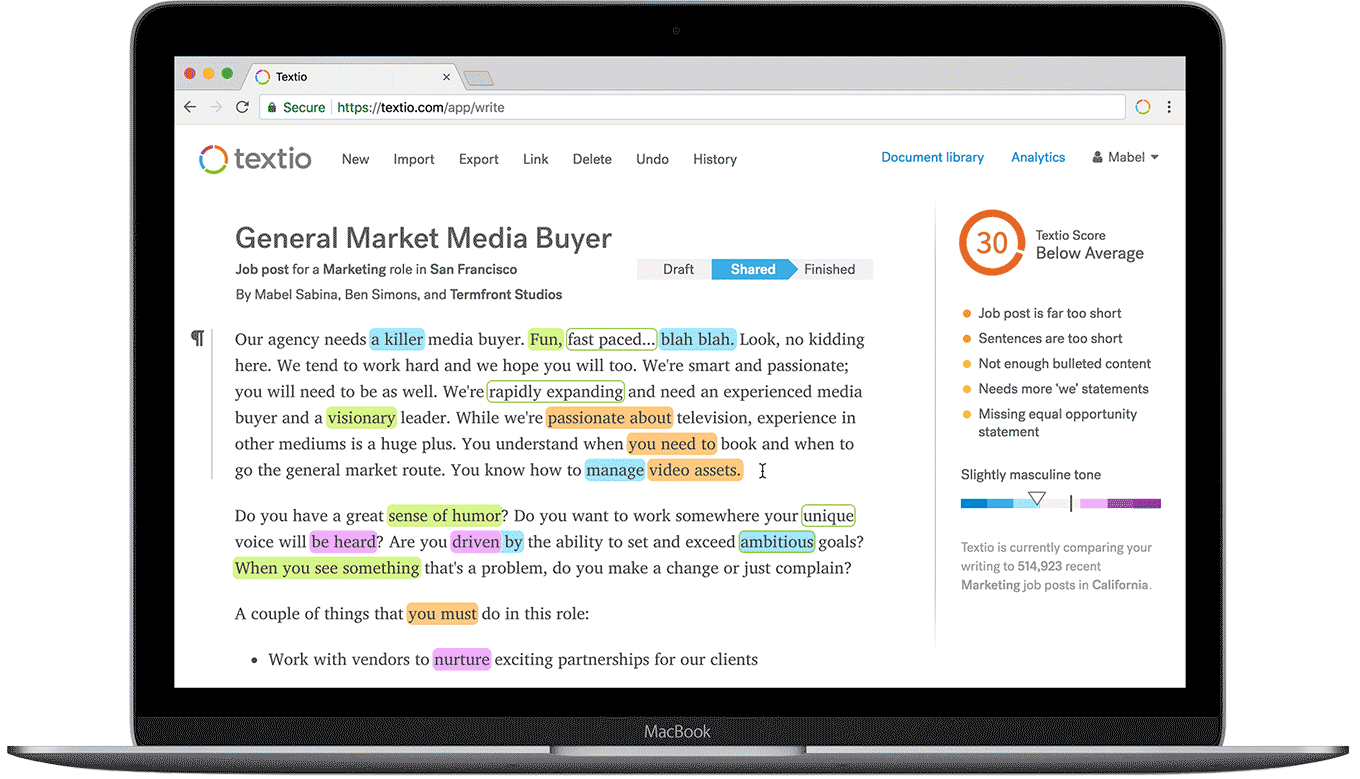
Textio’s real-time recommendations
Textio creates models that take into account the industry and location of the job, as well as in some cases, models that are unique to particular employers who use the service. It updates these models with new job descriptions and demographics of new applicants once a month.
. . .
Because job descriptions are usually a candidate’s first substantive touchpoint with a potential job opportunity, tools like Textio appear poised to help ameliorate gender biases within job descriptions. Textio is somewhat distinct among hiring technologies we observed, because it attempts to promote equity without making judgements about specific people. Even if the predictions they offer are imperfect, such tools still prompt employers to spend time trying to make their descriptions more inclusive.
Moreover, since a number of other predictive hiring products—from job ads to screening tools—rely on the words and phrases from job descriptions to inform their predictions about candidates’ suitability, more inclusive language in job postings can influence everything from who ends up seeing job ads to who is invited to interview.
Advertising
Many employers use paid digital advertising to put job opportunities in front a greater number of potential applicants. Today, employers have access to the same microtargeting, behavioral targeting, and performance-driven advertising tools as the broader e-commerce sector. How and where employers choose to use these tools plays an important role in determining the overall demographics of who learns about job postings and who ultimately applies.
How and where employers choose to advertise their jobs plays an important role in determining the overall demographics of an applicant pool.
Different kinds of online ad platforms let employers target potential applicants in very different ways. Job board platforms offer employers the ability to promote their job postings to particular types of jobseekers. General purpose search engines allow employers to place their ads next to search queries, targeting users based on their search terms and geographic locations, among other factors. Social media sites allow employers to show ads that blend in with other social content, targeting based on a wide array of personal characteristics, including demographic data and inferred interests. And millions of individual websites and mobile apps let employers place ads alongside other web content, and can be targeted to users who share common features or interests using a wide range of data sources. This “display” ad space is available to employers en masse through centralized ad networks.
Many ad networks use data that is both provided by users and inferred from their online activity. The data is used to automatically generate groups of users with certain shared attributes that recruiters can then use to target (or exclude people from seeing) ads. In selecting targeting options, employers define which users are eligible—though not guaranteed—to see a given job opportunity.
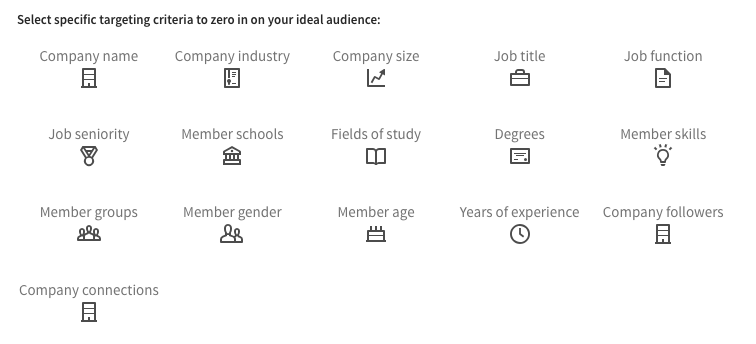
LinkedIn’s ad targeting options
Some platforms also offer employers the ability to target specific people, like people who previously visited an employer’s career website, or who began but did not complete an application.
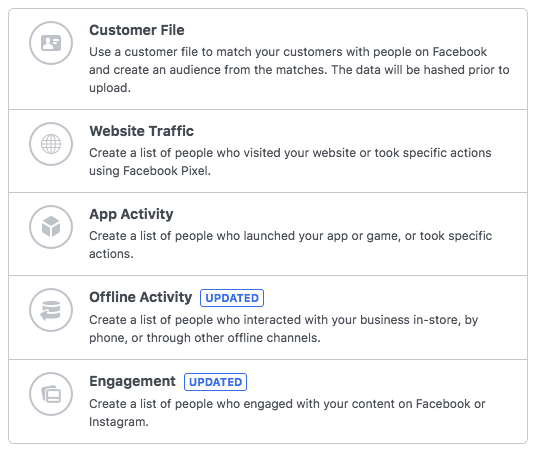
Facebook Custom Audience targeting options
And many platforms, including Facebook, Google, and LinkedIn, offer advertisers the ability to serve ads to users who are predicted to be similar to those the employer initially wanted to reach.
Beyond advertisers’ own targeting choices, ad platforms themselves play a significant role in determining who within a target audience will actually see each ad. While employers may set initial targeting parameters, it is typically the case that advertising space is limited, and not everyone who is eligible to see an advertisement will ultimately have it presented to them. Platforms like Facebook and Google decide which ads are ultimately shown to whom, not only based on advertisers’ willingness to pay, but on the platforms’ own prediction of how likely a user is to engage with the ad (e.g., clicking on it) or to take another desired action (e.g., applying to the employer’s job on the company’s career website).
. . .
As legal scholar Pauline Kim has argued, “not informing people of a job opportunity is a highly effective barrier” to applying for that position. How employers advertise can sharply limit, or greatly expand, the types of people who even learn a job opportunity exists. The targeting and delivery techniques described above are powerful, commonplace tools of the recruitment trade. However, we worry that employers, ad platforms, and regulators do not yet fully appreciate their impact.
In particular, sourcing platforms that deliver ads based on optimizations derived from user behavior, such as the number of clicks or job applications, risk directing ads and notices away from demographics that are historically less likely to take those actions. This could narrow the universe of underrepresented groups who are even presented with opportunities.
The complexity and opacity of digital advertising tools make it difficult—if not impossible—for aggrieved jobseekers to spot discriminatory patterns of advertising in the first place.
The complexity and opacity of digital advertising tools make it difficult, if not impossible, for aggrieved jobseekers to spot discriminatory patterns of advertising in the first place. Even if they could, it is not always clear who can or should bear legal responsibility for advertising practices with discriminatory effect. In the offline world, advertisers have been held liable for unintentional advertising practices that “serve to freeze the effects of past discrimination." However, it is unclear whether advertisers would be aware of these effects, or whether ad platforms themselves can or will be held liable for various discriminatory advertising practices. This is a fast-evolving area ripe for both empirical research and legal interpretation.
Digital advertising can also play a clear role in promoting equity. For example, federal contractors, who are obligated to “take affirmative action to ensure equal employment opportunity," and other employers committed to diversity and inclusion, may want to proactively target underrepresented groups for their job ads and may legitimately need access to seemingly sensitive targeting categories or predictive targeting tools. Even so, U.S. legal guidelines about acceptable job advertising practices have yet to be updated to account for evolving digital tools.
Matching
Matching is the process of comparing job opportunities with prospective applicants, typically culminating in a ranked list of recommendations. For instance, jobseekers might see personalized job recommendations, while recruiters might receive a ranked list of potential candidates. Matching tools promise to connect the right applicants with the right job, but by the same token, they can silently hide certain opportunities from some candidates and suppress others from being seen by recruiters. Personalized job boards and other predictive matching technologies are popular among both employers and jobseekers, in some cases supplanting employment and staffing agencies.
ZipRecruiter is one prominent matching product. It is essentially an online job board with a range of personalized features for both employers and jobseekers. ZipRecruiter is a quintessential example of a recommender system, a tool that, like Netflix and Amazon, predicts user preferences in order to rank and filter information—in this case, jobs and job candidates. Such systems commonly rely on two methods to shape their personalized recommendations: content-based filtering and collaborative filtering. Content-based filtering examines what users seem interested in, based on clicks and other actions, and then shows them similar things. Collaborative filtering, meanwhile, aims to predict what someone is interested in by looking at what people like her appear to be interested in.
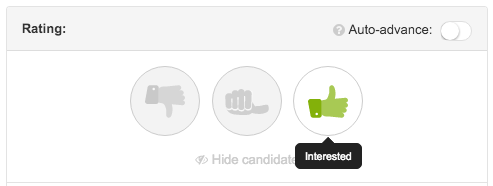
ZipRecruiter’s applicant rating interface
For example, on ZipRecruiter, employers can opt to give incoming applicants a “thumbs up.” As ZipRecruiter collects these positive signals, it uses a machine learning algorithm to identify other jobseekers in its system with similar characteristics to those who have already been given a “thumbs up”—who have not yet applied for that role—and automatically prompts them to apply. The details of the matching process make up ZipRecruiter’s special sauce, which considers not only basic demographic and skills information from resumes and other information added by jobseekers, but also insights gleaned from their behavior on the website.
For example, if two jobseekers have applied to many of the same jobs, that will strengthen ZipRecruiter’s assessment of their similarity. When one of them applies for a new job, and that employer gives that applicant a “thumbs up,” the other is more likely to be nudged to apply for that same job. If the second jobseeker does apply, that person’s application is marked for the employer with a “great match” badge, essentially reinforcing the employer’s initial screening decisions.
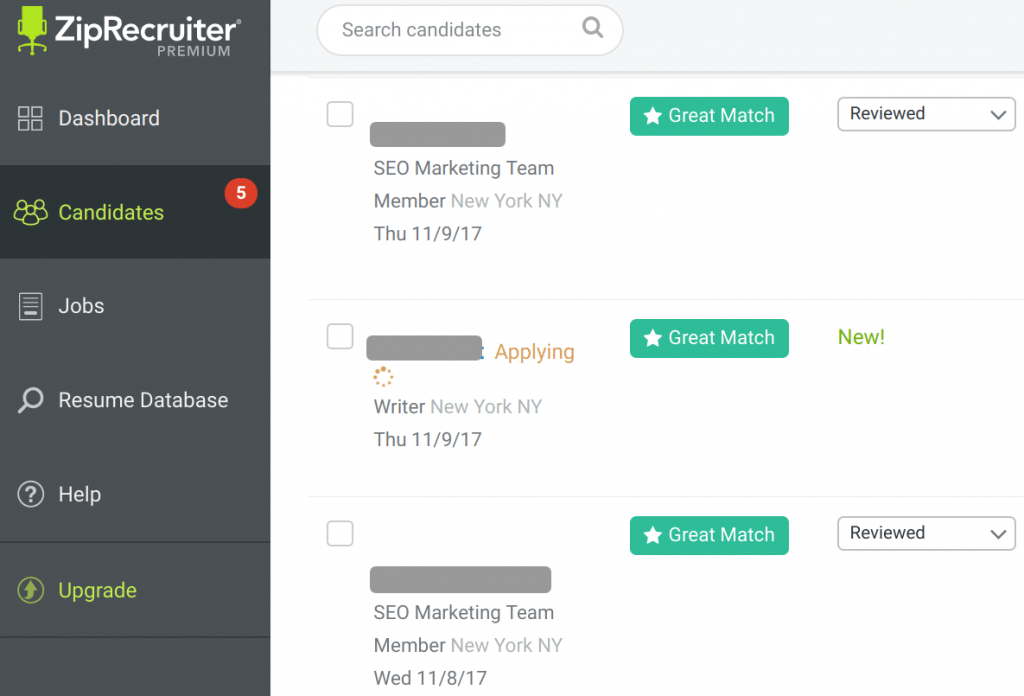
ZipRecruiter’s “Great Match” badges
According to the platform, its matching algorithm dramatically increases the fraction of preferable candidates in an applicant pool—at least in the eyes of a hiring manager. ZipRecruiter claims that without its algorithm, one in six applicants tends to get a thumbs up from an employer. But when its algorithm nudges “similar” candidates toward certain jobs, that rate increases to one in three applicants. One likely reason is that, as ZipRecruiter surfaces a job posting to jobseekers who are more likely to garner a thumbs up, it correspondingly suppresses the posting from others it deems less compatible.
ZipRecruiter uses similar algorithmic methods to filter jobs it displays to jobseekers, elevating certain openings based on their previous applications and other on-site activity and demoting others.
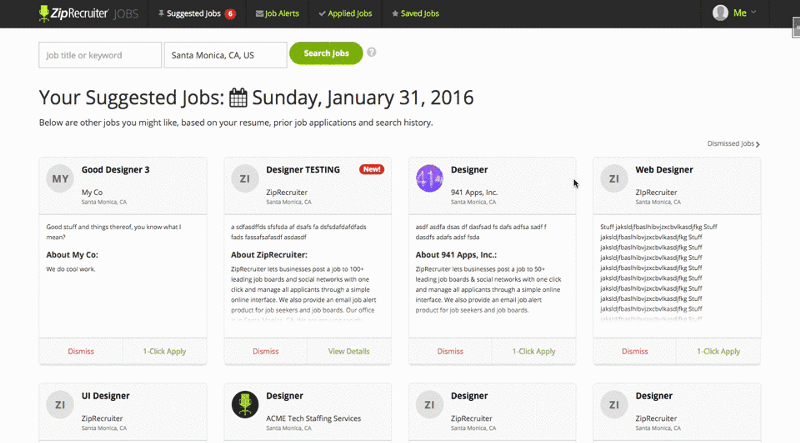
ZipRecruiter learns from user behavior
. . .
Job matching platforms like ZipRecruiter, and recommender systems more generally, present unique equity challenges. For one, tools that rely on attenuated proxies for “relevance” and “interest” could end up replicating the very cognitive biases they claim to remove. Content-based filtering can reinforce users’ own priors and cognitive biases. For example, if a woman with several years of experience tends to click on lower-level jobs because she doubts she is qualified for more senior positions, over time she may be shown fewer higher paying jobs than she would otherwise be qualified for. Collaborative filtering, on the other hand, risks stereotyping users because of the actions of others like them. For example, even if a woman frequently clicks on management positions herself, the system might learn that other, similar women tend to click on more junior positions, and might show her fewer management jobs than a similarly situated man—not due to her own preference, but because of the behavior of people the system deems to resemble her. Technical researchers are still trying to conceive of the right ways to benchmark and measure these systems, even outside of the hiring context.
Tools that rely on attenuated proxies for “relevance” and “interest” could end up replicating the very cognitive biases they claim to remove.
These effects can arise even when a recommender system does not explicitly consider protected characteristics, like race or sex. For example, when Netflix users noticed they were being shown content that appeared to be personalized by race, it was not because Netflix was collecting or explicitly inferring users’ race, but merely predicting users’ preferences using those users’ own behavior, and the behavior of others who appeared to have similar preferences. The same phenomenon can occur with hiring recommender systems, albeit less visibly.
Job matching platforms like ZipRecruiter and LinkedIn might fall between the cracks of existing legal protections. Here again, the role of technological platforms is ambiguous. On one hand, job postings on these platforms are clearly “notices or advertisements” under Title VII. However, platforms currently enjoy significant immunity from the conduct of other entities, such as employers, so it is not clear what legal obligations apply. The ACLU and others have argued that platforms can themselves be employment agencies and ought to be liable as such, but platforms contest this characterization. It is not even clear whether or when jobseekers using these tools would count as “applicants” under federal recordkeeping requirements, which were designed to help regulators monitor for disparate impact, even though some matching tools are making meaningful assessments about jobseekers’ qualifications before they explicitly apply for a particular role.
Headhunting
Headhunting is the practice of proactively reaching out to specific, qualified candidates. It is especially common when employers require specialized experience or are recruiting in competitive environments, often for higher-skill positions. Here, employers typically seek out “passive” candidates—that is, jobseekers who are either not aware of a particular job opening, or those who aren’t actively looking to leave their existing job or rejoin the workforce.
Entelo, a popular tool among Silicon Valley and technology sector employers, searches dozens of sources like LinkedIn, resume databases, and public social media and work portfolio profiles to surface potential candidates who may be receptive to individual outreach. In addition to visually displaying information about prospects’ skills and work history, Entelo makes several predictions about each potential candidate.
First, Entelo predicts whether someone is likely to move jobs, using data like whether she has recently updated her skills on LinkedIn, aggregate data about career trajectories in her field (for instance, how long employees tend to stay at the company where she currently works), and her current employer’s “health” (e.g., recent layoffs, mergers, and stock fluctuations).
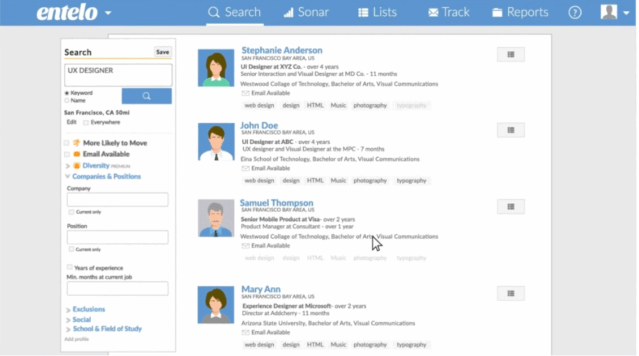
Entelo flags certain people as being “More Likely to Move”
Entelo also scores candidates on “company fit,” a measure based on whether a candidate has worked in companies of a similar size or industry as the recruiter’s company, and whether others have defected from the candidate’s current employer to the company interested in recruiting her.
Notably, Entelo uses data analysis and prediction as a means to actively further employers’ diversity goals in several ways. First, the company predicts whether someone is a “diversity” candidate—for instance, a person of color, woman, or veteran—based on candidates’ public affiliations with sororities, clubs, historically Black colleges, or special interest honor societies. Employers actively looking to recruit diverse candidates can use these predicted labels to search for them within Entelo’s database of passive candidates. And importantly, employers cannot use those categories to exclude candidates from a search. Employers can also opt to use “Unbiased Sourcing Mode,” which obscures personal, sensitive, and protected characteristics from the interface as they review candidates.
Recognizing that women and minority candidates may not use the same language or list the same skills on their resumes and online profiles as other candidates, Entelo offers a feature called “peer-based skills” that uses machine learning to compare profiles and predict skills a candidate is likely to have but may not have explicitly listed. Finally, Entelo offers employers reports that provide basic race and gender breakdowns for the candidates whom that employer has searched for and engaged on the platform.
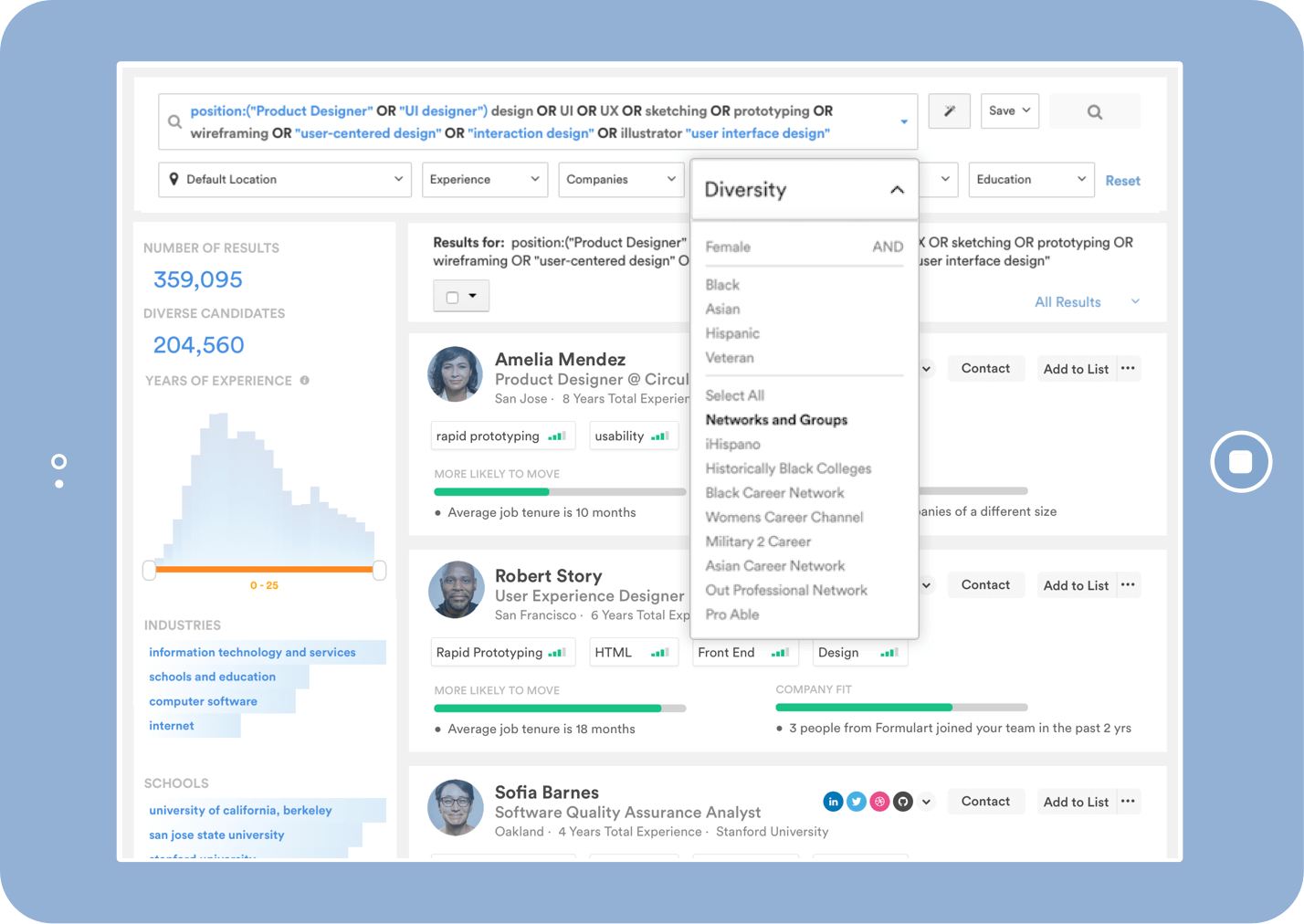
Entelo’s diverse candidate search function
LinkedIn also offers employers headhunting tools that rely on predictive indicators. Once recruiters select filters for candidates who have specific skills, LinkedIn returns a list of candidate profiles ranked by their “likelihood of being hired”—a measure the platform calculates using signals like whether a user is open to moving jobs, whether she follows the employer’s LinkedIn profile, and whether she is likely to respond to a message from a recruiter. The ranking also takes into account whether the candidate is from a region, industry, or company that the recruiter tends to prefer.
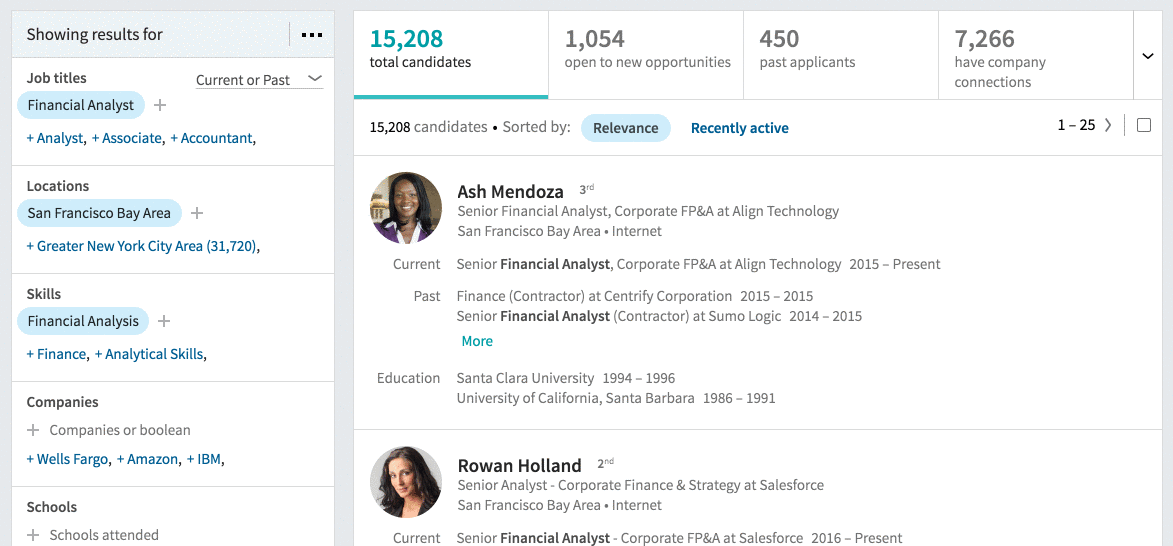
LinkedIn search results, sorted by “relevance”
Recently, LinkedIn updated its recruiter tools to balance the gender distribution in candidate search results, rather than sorting candidates purely by “relevance.” With this update, if the pool of potential candidates who fit given search parameters reflects a certain proportion of women, the platform will re-rank candidates so that every page of search results reflects that proportion. The company also plans to offer employers reports that track the gender breakdown of their candidates across several stages of the recruitment process, as well as comparisons to the gender makeup of peer companies.
. . .
Headhunting tools present some of the same fundamental concerns as matching tools. Rather than predicting more direct signals of “job success,” they often end up predicting recruiter or jobseeker actions, which can amplify biased social behaviors. This can happen especially quickly when predictive models are updated dynamically, as in recommender systems. For example, if an employer tends to click on the profiles of male software engineers, not only might she be shown more male software engineers, but other recruiters seeking candidates for similar roles may also see more male software engineers.
Moreover, male software engineers may start seeing these web developer jobs at a greater rate than women, whose profiles are not being clicked on at the same rate. Without intervention, these effects could be amplified over time, since people can only act on profiles and jobs that they are shown. These tools don’t completely block recruiters from seeing certain types of candidates, or certain types of candidates from seeing certain jobs. But the cumulative effect of being buried several pages deep in search results could have similar effects.
There are also familiar legal ambiguities. Regulators lack clear guidelines to assess disparate impact. Nor is it clear whether the candidates considered by these tools are “applicants” for recordkeeping and assessment purposes.
Headhunting tools appear prone to explicitly prioritize measures of “company fit” or “likelihood of being hired” at that company. To some extent, these measures resemble analog assessments of “culture fit,” which might disadvantage applicants who have not had the opportunity to work in similar companies, despite their abilities.
There are some encouraging new practices in this class of technology. Entelo’s diversity-aware reporting tool could help employers identify their recruiting activities that may be biased against women and candidates of color. LinkedIn’s gender-aware candidate search results feature is another step in the right direction. Vendors should carefully consider expanding such an approach beyond gender, to ensure that other kinds of underrepresented candidates are surfaced more proportionally to the makeup of the underlying candidate pool. In addition, Entelo’s “peer-based skills” feature, which augments the skills on a candidate’s profile, claims to lift up qualified female candidates. In theory, such a function could do so, but the company’s public statements about the feature are not detailed enough for us to confidently say that the tool works as described.
Screening
In the screening stage, employers formally begin reviewing applications, rejecting unqualified or relatively weak applicants and prioritizing the remainder for closer consideration. Here, predictive technologies assess, score, and rank applicants according to their qualifications, soft skills, and other capabilities to help hiring managers decide who should move on to the next stage. These tools help employers quickly whittle down their applicant pool so they can spend more time considering the applicants deemed to be strongest. A substantial number of job applicants are automatically or summarily rejected during this stage.
Qualifications
Many employers will consider applicants’ existing qualifications, such as prior experience in a given role, certifications, or proficiency with particular software systems. In some contexts—like retail and service sectors—nearly all minimally qualified candidates may be offered employment. For lower-volume recruitment, meeting hard qualification requirements is a prerequisite for more in-depth consideration.
Many simple applicant tracking systems offer features to screen out applicants who don’t appear to have the minimum requirements or skills, based on lists of predefined questions or keywords, often called “knockout questions.” However, more advanced tools, such as interactive online tests or software tools that automatically analyze written answers, aim to improve the traditional screening process using more sophisticated analysis.
One example is Mya, a chatbot that allows employers to engage with jobseekers in an interactive manner. Chatbots like Mya are gaining popularity as tools to automate the screening process, particularly for employers trying to fill high-volume, high-turnover jobs. Like traditional job application software, Mya asks jobseekers basic screening questions. The tool does not appear to make nuanced predictions about candidates, but rather interprets written answers to predefined questions and responds in a conversational manner.
Mya can begin interacting with jobseekers before they submit formal applications, answering initial questions by chat, text message, and email. The bot extracts key details from text-based conversations using natural language processing (NLP), and then uses basic decision trees to determine the appropriate response and action.
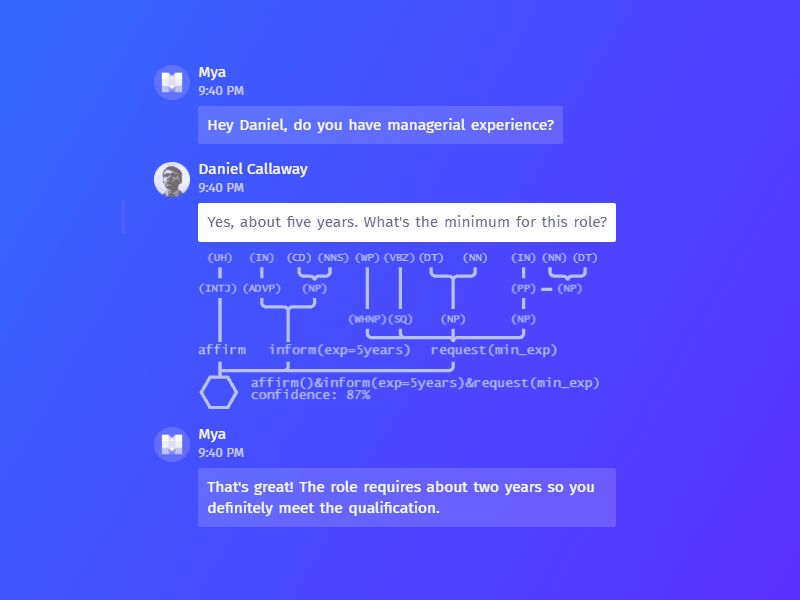
The Mya chatbot can pre-screen candidates
When Mya determines that candidates meet an employer’s predefined requirements, it automatically passes them directly to the next stage of the process or puts them in touch with a human recruiter. If the bot detects candidates that are a “poor-fit,” it can be configured to preemptively discourage them from applying for a job, “reject[ing] candidates gently, suggesting other job openings they may be qualified for and/or inviting them to register in the talent pool.”
Other screening tools help recruiters look beyond keywords and pre-set questions, such as reviewing applicants’ resumes automatically using machine learning techniques.
One such tool, Ideal, predicts how closely an applicant’s resume matches the employer’s minimum and preferred qualifications. Ideal extracts and interprets the text of an applicant’s resume and, based on that employer’s past screening and hiring decisions, assigns the applicant a letter grade, from A to D.
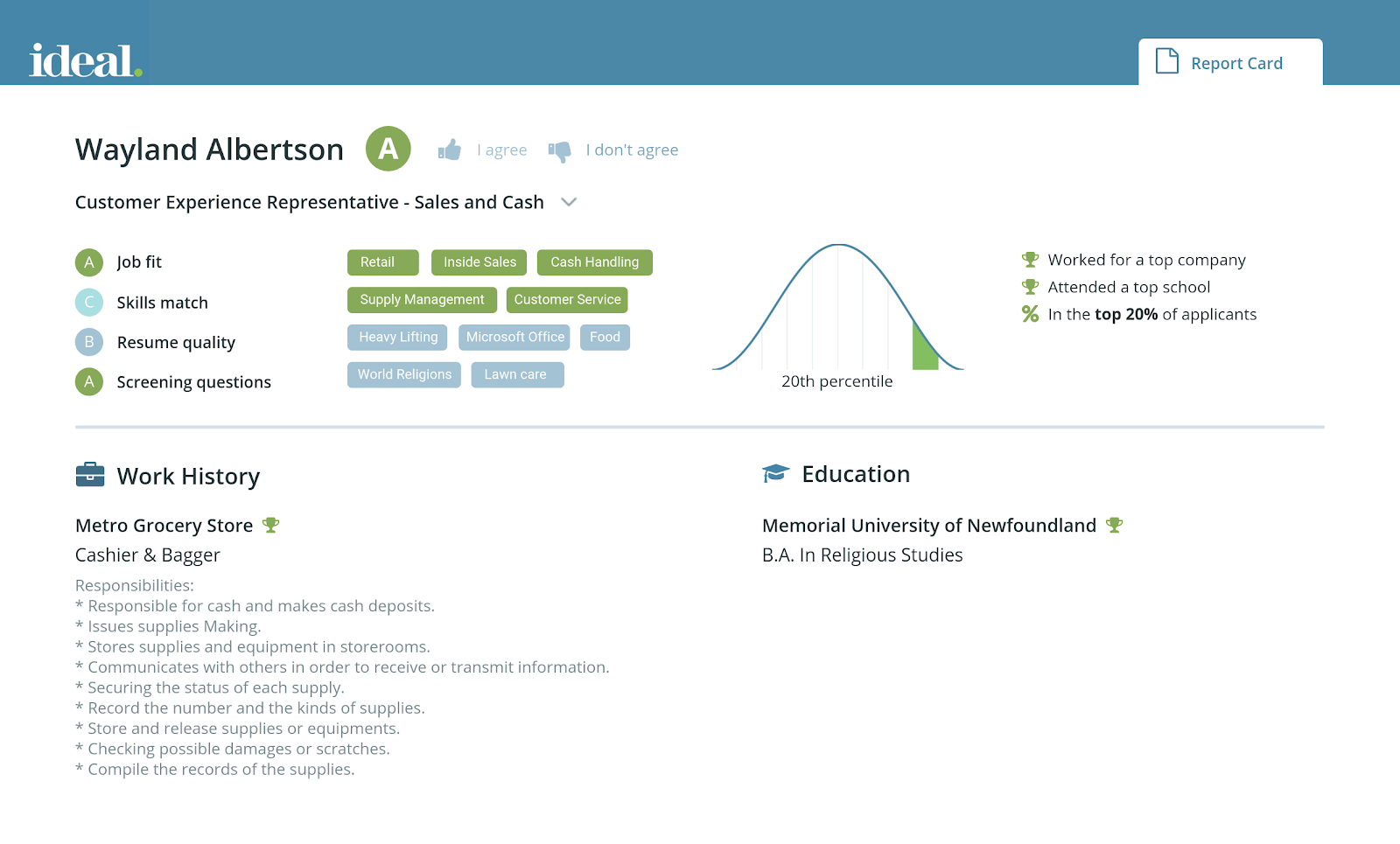
Ideal’s dashboard displays letter grades and other predicted details
Ideal allows hiring managers to give feedback to its screening algorithm, by indicating whether they “agree” or “don’t agree” with the assessment of a particular applicant.
. . .
Tools like Mya and Ideal offer employers ways to more efficiently screen large applicant pools with relatively standardized procedures. In theory, such processes could benefit qualified candidates who might have been accidentally ignored or screened out by strict knockout questions, or due to resource limitations or interpersonal biases. Unsurprisingly, both companies highlight the fact that their software does not explicitly consider factors like race, gender, or socioeconomic status.
When screening systems aim to replicate an employer’s prior hiring decisions, the resulting model will very likely reflect prior interpersonal, institutional, and systemic biases.
When screening systems aim to replicate an employer’s prior hiring decisions, as Ideal does, the resulting model will likely reflect prior interpersonal, institutional, and systemic social biases. Although it might seem natural for screening tools to consider previous hiring decisions, those decisions often reflect the very patterns many employers are actively trying to change through diversity and inclusion initiatives. Workplace performance data, while itself at risk of reflecting similar biases, may at least surface nontraditional signals of likely success an employer has not previously considered.
Moreover, although natural language processing techniques have advanced in recent years, researchers have found that NLP systems trained on real-world data can quickly absorb society’s racial and gender biases. One study found, for example, that NLP tools learned to associate African-American names with negative sentiments, while female names were more likely to be associated with domestic work than professional or technical occupations. Limitations in the diversity of NLP training data mean they may perform poorly with candidates who have regional or cultural dialects, or for whom English is a second language. Tools that rely on NLP could therefore reflect “expected” linguistic patterns and, as such, could misunderstand, penalize, or even unfairly screen out minority candidates. Some researchers are seeking to develop more inclusive models, but such research is still in its infancy.
Finally, while chatbots used in hiring today appear to be relatively simple—following a pre-approved script—future hiring chatbots might be given more flexibility. If vendors begin to experiment with chatbots that learn from social interactions with users, they will need to take care that they don’t autonomously parrot user-generated misbehavior and prejudices.
Assessments
Many employers, particularly larger employers, use pre-employment assessments to measure aptitude, skills, and personality traits to differentiate potential top performers from other applicants. Today’s assessment tools, which often build on these traditional tests, are appealing for employers who want to spot the strongest candidates among a large pool of qualified candidates.
Predictive assessment tools are just emerging, but they are quickly gaining popularity. Some vendors offer “off-the-shelf” assessments for a variety of job functions (like customer service, sales, and project management) and competencies (like “problem solving” and “interpersonal skills”). For example, job board website Indeed offers a library of such tests that employers can include in their online job applications. Applicants take the tests during the online application process, which Indeed automatically scores “with the help of machine learning.” These ready-made assessments are intended to predict generic job performance and aren’t specific to a given employer or applicant pool.
Other vendors offer custom-built assessments for particular employers, and for specific roles. These bespoke assessments use the employer’s workforce and performance data to predict how new applicants may compare to current “successful” employees.
One vendor, Koru, offers an assessment tool that infers candidates’ personality traits to predict future job performance. The tool poses questions to candidates through a self-assessment survey, and based on their answers, scores candidates on personal attributes like “grit,” “rigor,” and “teamwork,” as well as their predicted alignment with an employer’s desired traits.
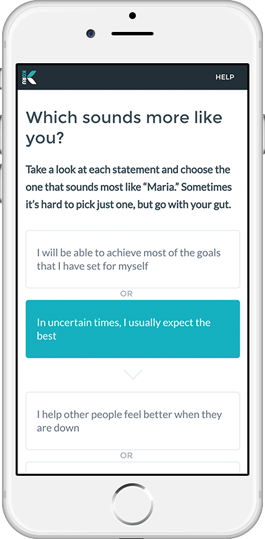
Koru’s self-assessment interface
To determine the desired trait profile for a specific employer, Koru has a group of existing employees complete its assessment, collecting several hundred data points per employee. It cross-references that information with the employer’s own performance indicators for those employees (like employee reviews, promotions, or sales numbers) to identify the personality traits that most differentiate a company’s high performers from its low performers. The result is a “fingerprint” for a specific position—that is, the particular mix of personality traits that Koru finds to be most correlated with success on the job, against which future applicants are evaluated.
For each new applicant, the employer receives an overall percentage “fit” score, as well as individual scores for specific characteristics and priority skills.
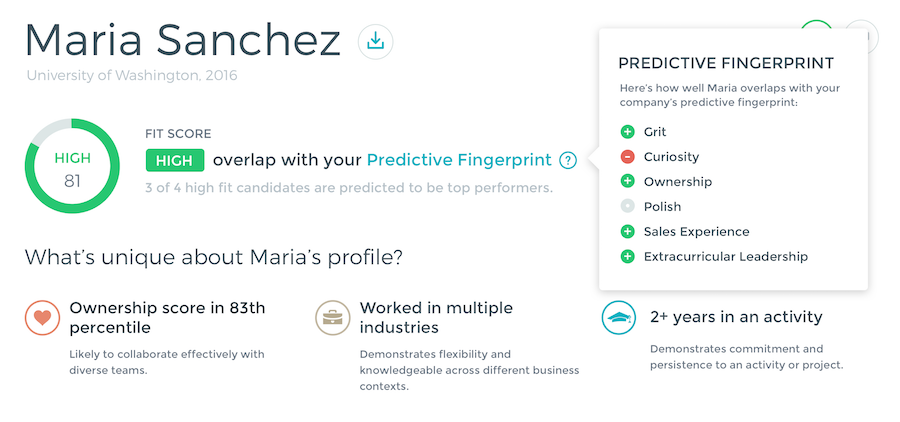
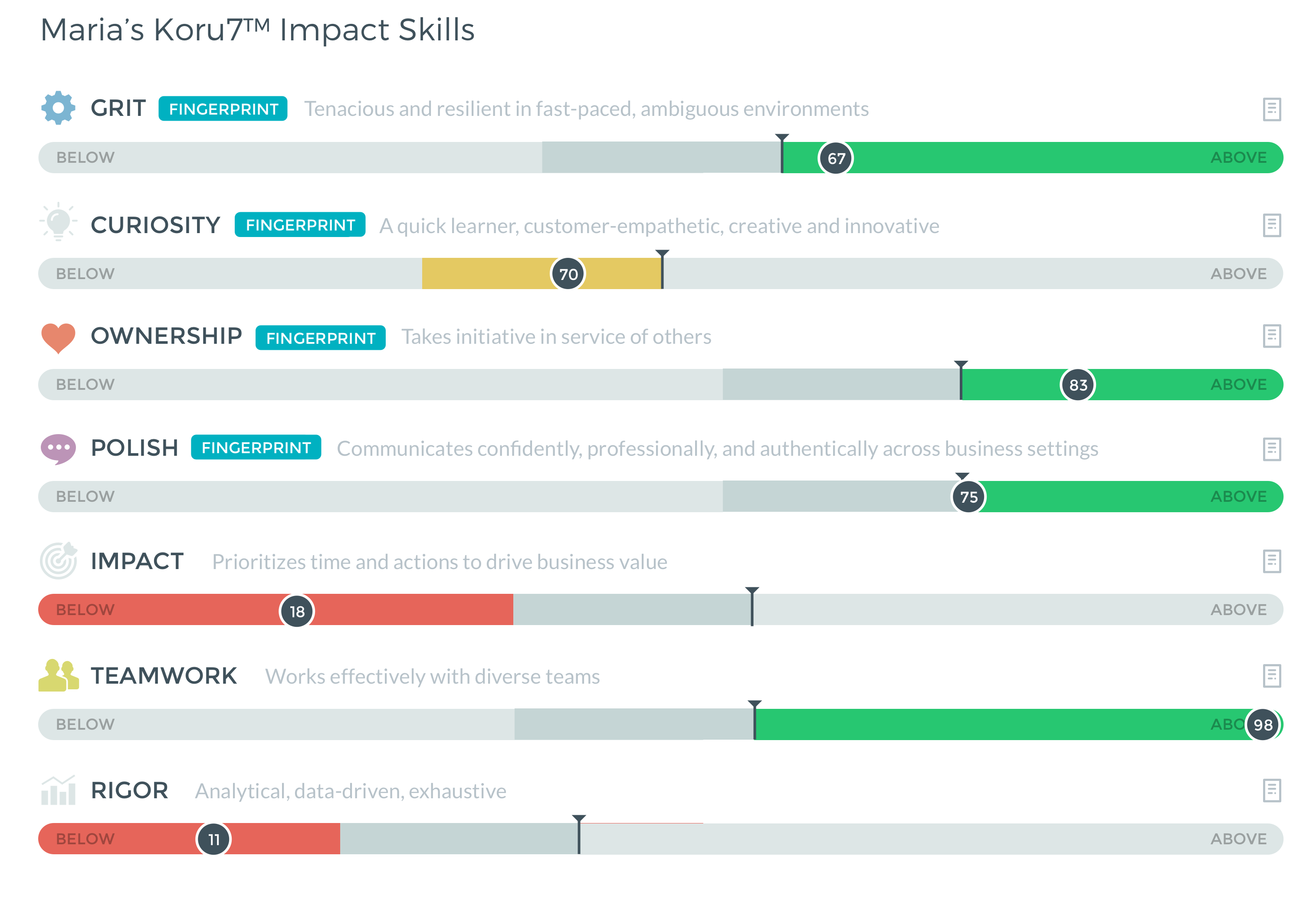
Koru’s assessment results, including a “predictive fingerprint”
Based on candidates’ predicted fit scores, Koru sorts them for review, and the employer can filter the list of candidates by “low,” “medium,” and “high” fit, by specific strengths, and by standard resume information like college, major, and prior work experience.
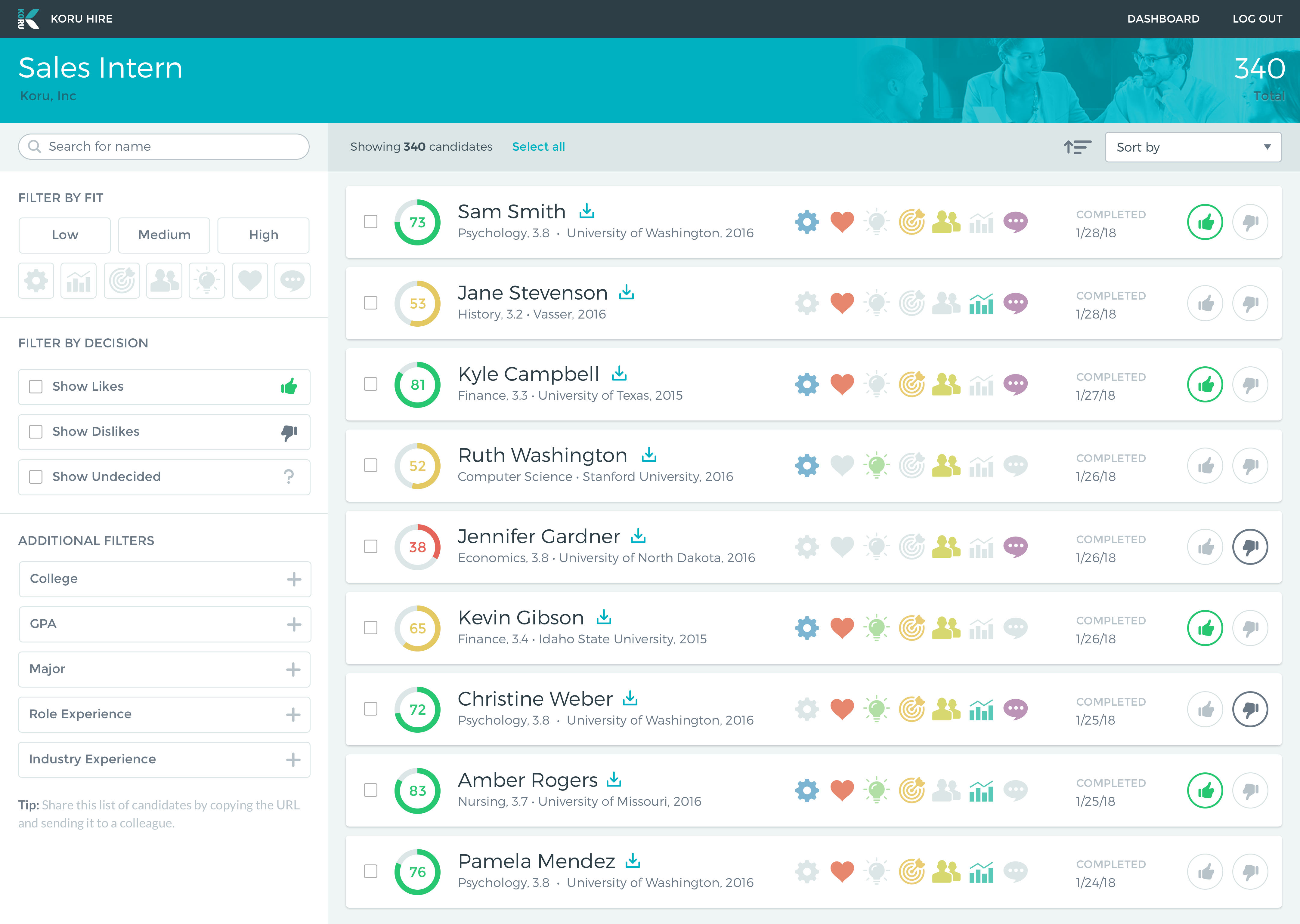
Like many other predictive hiring tools, Koru scores and ranks candidates
The company has mentioned on several occasions that their tests have been validated and evaluated for adverse impact on women and minority candidates, but it does not disclose its methods nor the results of its analysis.
Like Koru, other vendors seek to assess candidates’ personality traits, but rather than asking candidates to fill out a survey—which candidates could fill out inaccurately—they offer games and interactive activities that purport to measure candidates’ behaviors more directly.
Pymetrics is one prominent vendor that offers “neuroscience” web and mobile games to measure cognitive, social, and emotional traits of candidates, such as processing speed, memory, and perseverance. For instance, one of their games flashes red and green dots on the screen and asks players to click when they see a red dot. The game appears to measure candidates’ reaction times, but in fact is used to assess candidates’ impulsivity, attention span, and ability to learn from mistakes.
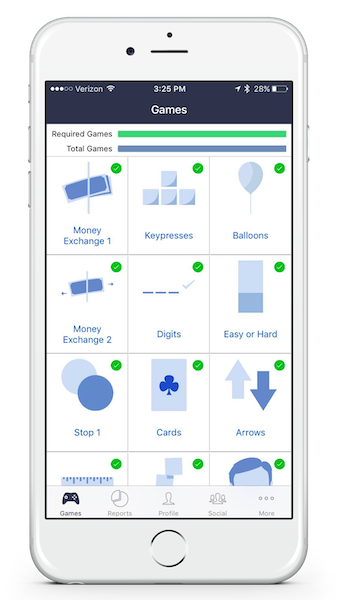
Pymetrics’ interactive games
Like Koru, Pymetrics builds custom predictive models for each employer and for specific positions. Before doing so, the company starts by gathering data from tens of thousands of people (not specific to the employer) in order to distill baseline “trait profiles” for different types of game players. The employer then asks current employees to play many of Pymetrics’ stock games. To build a predictive model, Pymetrics applies machine learning techniques to determine which traits—as measured by its games—best differentiate the employer’s top performers from its other employees. Of course, for this to work, the employer needs to tell Pymetrics who it considers to be its top performers, based on whatever metrics the employer is already using to assess its employees.
When the Pymetrics model is ready, the employer asks each new job candidate to play the games. Based on their game play, Pymetrics calculates a percentage score for each candidate, indicating how well that candidate matches with the employer’s desired suite of traits for the job.
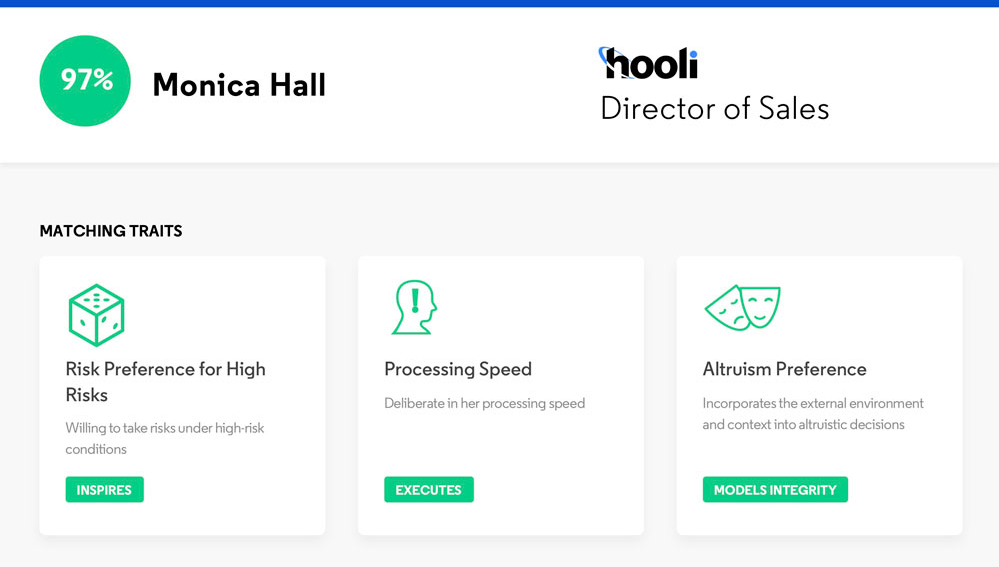
Pymetrics’ assessment results
Candidates whose scores fail to meet the employer’s predefined threshold are automatically rejected for the specific role. Interestingly, if the employer is hiring for multiple roles, Pymetrics offers a “common application”-style service, redirecting candidates to other open roles with the same employer, or elsewhere, for which their inferred traits appear to be a better match.
Pymetrics is adamant that its assessments comply with U.S. legal requirements. The company appears to be aware that how employers currently assess “top” performers is very likely to be biased along gender and racial lines, and that such biases could easily be reflected in their resulting models.
Pymetrics does offer some public explanation regarding the steps it takes to “de-bias,” or mitigate observed disparities in, its models. The company explains that they use statistical techniques to remove obvious demographic biases when evaluating behavioral traits. It also tests its models for differential impact along gender and racial lines. When statistical disparities are detected, Pymetrics apparently further adjusts its models in an attempt to compensate, though they do not describe the details of this stage of the process. In May 2018, Pymetrics publicly released the source code of an internal tool it developed to identify biases in its own models. While this is a worthwhile step, it does not make the models that it develops for employers available for external, independent auditing.
. . .
Pre-employment tests have a deeply troubled history, and have long been decried as being inherently discriminatory against both people of color and people with disabilities. The newest assessment offerings raise similar questions and concerns about validation, structural biases, and their influence on human decision-making.
Tools like Koru and Pymetrics exemplify some of the most fundamental concerns about predictive technology used in hiring. The very act of differentiating high performers from low performers often reflects subjective evaluations, which is a notorious source of discrimination. Models based on these practices can mirror undesirable social patterns. Even when these tools accurately infer traits that current, successful employees share, they could easily turn away equally talented candidates who don’t happen to share those characteristics. Inferred traits may not actually have any causal relationship with performance, and at worst, could be entirely circumstantial. Tools with “common application” features could rely on such traits to unfairly redirect certain candidates to lower status jobs.
It is not clear that existing legal best practices apply to, or provide an effective check on, predictive assessment tools.
It is not clear that existing legal best practices apply to, or provide an effective check on, these tools. The EEOC’s guidelines for “tests and other selection procedures” say that these tests and procedures should be “validated”—that is, shown to be sufficiently related to or predictive of job performance. Perhaps because of this guidance, most bespoke assessment tools we observed, including Koru and Pymetrics, are not built to incorporate feedback in real time, updating themselves as more candidates are considered and hired. Rather, the models appear to be created more deliberately, with distinct models built for each position and each employer. Moreover, because machine learning tools enable employers to correlate nearly any test to some aspect of job performance, existing validation guidelines may be ill-equipped to prevent discriminatory outcomes.
Validation notwithstanding, such tools (and most personality tests) are built on fundamental psychological theories of human behavior that reflect particular historical and social patterns. Applicants of different genders or from different cultural backgrounds could describe themselves or act differently, for instance, even if they have similar competencies. Many psychology and behavioral research studies have relied on college students as subjects, and researchers have questioned whether those studies can truly be generalized to wider populations. New social science research methods, like those that use online crowdsourcing techniques, allow researchers to access a wider diversity of subjects, but such methods present their own unique experimental validity and ethical challenges. Either way, such tests could penalize jobseekers who don’t fit a traditional mold, especially those with disabilities.
Also concerning is the fact that many assessment systems assign candidates specific, numerical “fit” scores, and then rank and display candidates to recruiters according to those scores. This can create the perception of substantial difference between candidates where there may be little, if any. The problem is especially stark when (as is common) predictive models are based on employee performance data, which employers often admit, at least in casual settings, are of poor quality. Even for candidates who pass an initial screening round, these numbers and rankings create an illusion of statistical accuracy and specificity that could color how recruiters view candidates during the remainder of the hiring process.
Finally, the information that’s displayed to employers by a tool’s user interface can have subtle but powerful effects on hiring outcomes. For instance, recruiters will likely focus first on candidates with the very highest scores. But if black and white candidates pass an assessment at equivalent rates, and if black candidates on average tend to receive marginally lower passing scores than white candidates, black candidates will likely fare worse over time. One vendor, Applied, demonstrates a promising approach by randomizing the order in which candidate materials are shown to human reviewers.
Interviewing
In the interview stage, employers interact directly with individual applicants, and hiring decisions often crystalize at this stage. Emerging tools at this stage claim to measure applicants’ performance in video interviews, by automatically analyzing verbal responses, tone, and even facial expressions. Employers might use these tools to save interviewers time, relieve scheduling burdens, and standardize what is often seen as an inescapably subjective part of the hiring process.
One prominent video interviewing company, HireVue, lets employers solicit recorded interview answers from applicants, and then “grades” these responses against interview answers provided by current, successful employees.
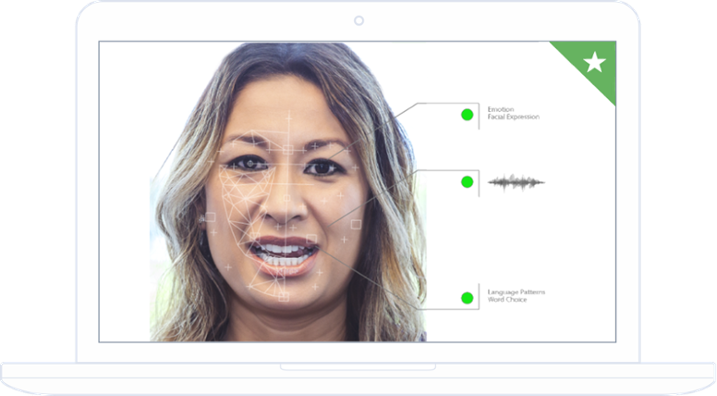
HireVue analyzes facial expressions, language patterns, and audio cues
More specifically, HireVue’s tool parses videos using machine learning, extracting signals like facial expression and eye contact, vocal indications of enthusiasm, word choice, word complexity, topics discussed, and word groupings. It uses these signals to create a model that claims to capture relationships between interview responses and workplace performance, based on the employer’s preexisting metrics.
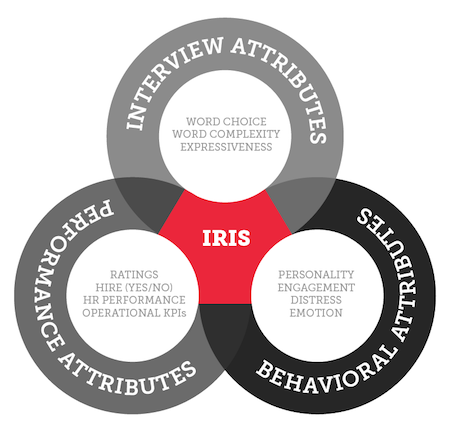
HireVue’s description of the data included in its models
As new candidates submit responses for an open role, HireVue uses these models to produce an “insight score” of 0-100 for each candidate. Employers can choose to automatically pass high-scoring candidates along for further review. Inversely, candidates who score below a certain threshold can be automatically rejected.
HireVue says it tests the models it creates for certain kinds of bias. For example, HireVue claims to test each model on different demographic subgroups in order to detect adverse impact on the basis of gender, race, and age. If such bias within the model is detected, the company explains that it identifies the specific factors in the model that contribute to those differences and removes them before retraining, validating, and deploying the new model. Once an employer begins accepting applications, the model is periodically checked for both accuracy and adverse impact.
. . .
There is significant public concern about video interviewing systems like HireVue, and for good reasons. Speech recognition software can perform poorly, especially for people with regional and nonnative accents. Facial analysis systems can struggle to read the faces of women with darker skin. Both kinds of systems are likely to improve over time, as new and more inclusive data sets become available.
But the critiques go deeper than accuracy. Some skeptics question the legitimacy of using physical features and facial expressions that have no credible, causal link with workplace success, to make or inform hiring decisions. Tests that have the effect of considering someone’s immutable characteristics—even if they do so in a facially legal way—may violate expectations of dignity and justice, and prevent candidates from making a good-faith effort to demonstrate their suitability for a job. Moreover, some worry that interviewees might be rewarded for irrelevant or unfair factors, like exaggerated facial expressions, and penalized for visible disability or speech impediments.
Even if affirmative selection decisions are made by humans, automated rejections are still concerning.
In response to these critiques, HireVue, like many other vendors, points out that it does not make any decisions about whom to hire, but merely helps to inform human recruiters. But even if affirmative selection decisions are made by humans, automated rejections are still concerning. On the bright side, HireVue’s software at least appears to allow employers to hide its automatically generated “insight score” from subsequent reviewers, potentially mitigating overreliance on its measurements further along in the hiring process.
While HireVue seems to take some steps to remove bias from the models it creates, the company hasn’t shared many details about how it does so. Absent further transparency, advocates and regulators cannot fully assess the efficacy of their efforts.
What About De-Biasing?
In recent years, academic and industry researchers have been working to develop techniques to “de-bias” predictive models. These techniques often involve testing for disparate outcomes (using collected or inferred protected characteristics) and then adjusting the model’s behavior accordingly.
However, best practices have yet to crystallize. Many techniques maintain a narrow focus on individual protected characteristics like gender or race, and rarely address intersectional concerns, where multiple protected traits produce compounding disparate effects. The issue itself is only starting to emerge as a research focus in the computer science community.
Some hiring technology vendors seem to have embraced de-biasing methods to address racial and gender discrimination, which should be encouraged and celebrated. However, there is still more work to do: We did not identify any vendor that appeared to assess adverse impact based on other sensitive features, like religion, national origin, disability, or sexual orientation, which could just as easily emerge when predictive tools are used.
Bias testing in hiring tools today is almost always opaque to the public, performed internally by companies, and lacking independent validation, making the results of internal tests and vendor claims difficult to verify or challenge.
In sum, the development and deployment of de-biasing techniques are promising and will likely play an important role in the future of predictive hiring technology. But there are limits: Some predictors of “success” may be so entwined with protected attributes that de-biasing will be insufficient. In these cases, other kinds of equity-promoting interventions will be needed.
Selection
In the selection stage, employers make final hiring decisions, which might include background checks and negotiation of offer terms. Here, hiring tools aim to predict whether candidates might violate workplace policies, or to estimate what mix of salary and other benefits to offer. Employers who use these tools often seek to increase their “yield” of new hires from extended offers, on terms favorable to the employer. For applicants, this is a critical moment of negotiation.
Background Checks
Employers commonly run pre-employment background checks, most often to determine if an applicant has a criminal history or if they are authorized to work. Automated background checks have long concerned civil rights advocates, who highlight the fact these systems tend to have a disproportionate negative impact on workers of color, immigrants, and women. Today, few employers use predictive technology in a way that changes the nature of background checks—but a few companies are trying to change that.
One background check vendor, Fama, offers employers a service to flag candidates at risk of engaging in sexual harassment, workplace violence, and other “toxic behavior.” Fama says it makes these assessments based on public online content, like social media posts, using automated content analysis tools.
Another vendor, Predictim, offers a similar background check service for potential childcare providers. Until recently, Predictim used Facebook, Twitter, and other social media data, to generate reports claiming to assess potential caregivers’ likelihood to engage in “bullying/harassment, disrespectfulness/bad attitude, explicit content, and drug abuse,” and assigning applicants scores from 1 (low risk) to 5 (high risk) based on that assessment.
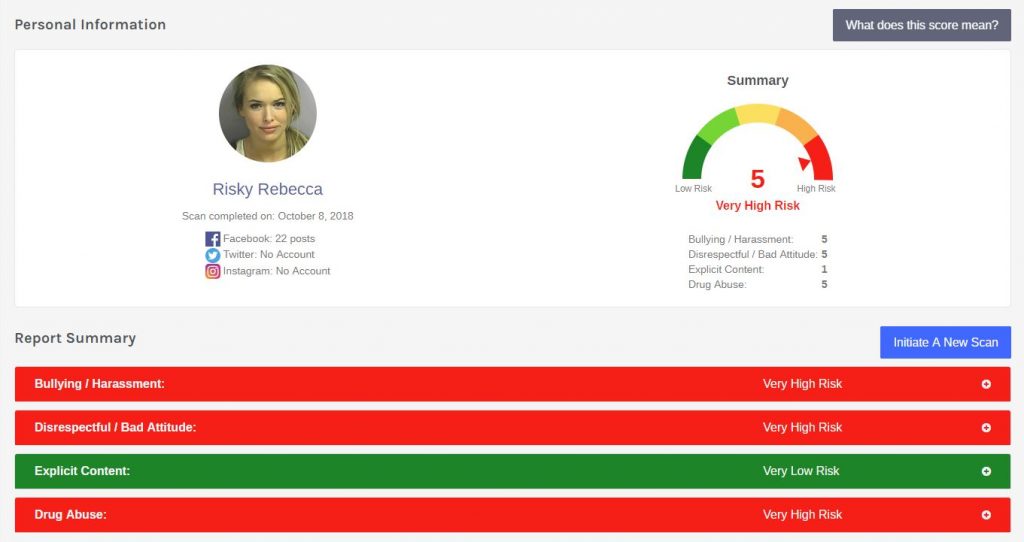
A sample Predictim report
Following critical press coverage of the service, both Facebook and Twitter revoked the vendor’s access to user posts, determining that the tool had violated the platforms’ policies. For Facebook, the platform’s developer policy prohibits the use of Facebook data to inform “eligibility decisions,” such as hiring decisions, while Twitter prohibits using its data for “surveillance purposes,” including background checks. Predictim responded that it will continue operating its service, but using other data sources like blog posts and Reddit.
. . .
Social media background checks are fraught for several reasons. First, they presume that a person’s online behaviors, like some use of foul language, are relevant to their professional activities. Second, such tools “have limited ability to parse the nuanced meaning of human communication, or to detect the intent or motivation of the speaker.” Even the most advanced technology companies struggle to define and automatically identify “toxic” content. Finally, background checks could surface details about an applicant’s race, sexual identity, disability, pregnancy, or health status, which employers should not consider during the hiring process.
Social media background checks are constrained by a range of laws and corporate policies. In the United States, the Fair Credit Reporting Act often applies, imposing accuracy requirements and other consumer protections. State laws also govern background checks, with some states barring employers from demanding access to applicants’ social media accounts. Social media companies are also increasingly barring background check vendors from accessing their users’ data. For all the reasons above, we do not expect significant growth in this space.
Offer
Employers make offers to applicants who make it through the hiring process, which typically include details about salary, benefits, start date, and other details. Hiring tools at this stage often help employers plan for onboarding activities and payroll changes. But a few of these tools are also offering individualized predictions about what specific offer candidates are likely to accept.
For example, enterprise software company Oracle, through its omnibus Recruiting Cloud product, provides employers with predictions about the likelihood a candidate will accept a job offer, and what the employer can do to increase the candidate’s chance of acceptance. The employer can adjust salary, bonus, stock options, and other benefits to see in real time how the prediction changes. The tool can update itself with employers’ data about the outcome of previous offers and acceptances over time.
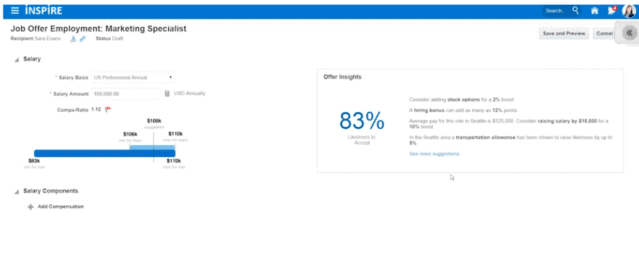
Oracle’s prediction of a candidate’s likelihood of accepting an offer updates in real time as the employer adjusts offer parameters
. . .
We worry that tools like this might amplify pay gaps for women and workers of color. Human resource data commonly include ample proxies for a worker’s socioeconomic and racial status, which could be reflected in salary requirement predictions. In any case, offering employers highly specific insight into a candidate’s salary requirements increases information asymmetry between employers and candidates at a critical moment of negotiation.
Offering employers highly specific insight into a candidate’s salary requirements increases information asymmetry between employers and candidates at a critical moment of negotiation.
These tools might also undermine—or even conflict with—laws that bar employers from considering candidates’ salary histories when making compensation decisions. Such laws are being enacted across the country precisely to address entrenched pay disparities. But if employers can predict someone’s past salary to a degree of relative accuracy, they no longer need to ask.
On the brighter side, these same types of tools can provide employers with a chance to reflect on their own pay practices. Enterprise human resource technology companies like ADP and Workday, as well as several vendors that primarily focus on diversity and inclusion, now offer features to assess pay gaps. However, it is unclear whether these analyses are available to hiring managers at the time offers are made, or whether the tools simply offer aggregate, after-the-fact analysis. Nevertheless, this type of reflective analysis presents a promising direction for advanced technology used at this critical stage of hiring.
Performance Evaluation: Shaping Future Recruitment Decisions
After the hiring process, employers continuously evaluate the performance of their employees, judging their productivity and quality of work to inform pay, promotion, and termination decisions. The outcomes of these evaluations—even absent direct involvement by technology—play a major role in shaping predictive models used to judge future job applicants. It’s important for employers to understand the inherent limitations of performance data before relying on them to guide future hiring decisions.
Recruiters are understandably interested in using insights about successful employees to help hire new ones. But according to McKinsey, only 14 percent of executives believe they can actually identify high and low performers at their companies.
Scholars of business operations point out that even seemingly robust performance data can be deeply flawed. Performance reviews and ratings have been shown on multiple occasions to reflect bias on the basis of race and gender, and promotion and pay practices suffer from the same problem. Employers could also introduce new opportunities for interpersonal bias, if they solicit feedback from customers about their interaction with employees. Even basic signals of success, like tenure at a company, can reflect enduring effects of workplace discrimination, including racial and gender-related discrimination and sexual harassment.
Institutional practices can taint the performance and promotion data that is commonly the wellspring for predictive hiring tools.
Institutional practices can taint the performance and promotion data that is commonly the wellspring for predictive hiring tools. Take for example Google, which hires employees into a system of hierarchical team and supervision structures (“ladders”) that determine promotion opportunities and compensation levels. Roles on technical ladders pay higher salaries and are more prestigious internally than roles on non-technical ladders. But lawsuits have alleged that, as recently as 2017, the company systematically discriminated against women in salary and promotion decisions by placing them on less prestigious ladders and lower salary bands than men with similar duties and experience, while promoting women more slowly and at lower rates than their male peers. Such practices are not unique to Google. When predictive tools are based on such flawed data, it raises fundamental questions about their utility in the first place.
Some employers are attempting to improve the quality of their performance data by measuring worker behavior and productivity more directly, but such techniques raise their own unique concerns about worker surveillance, privacy, and other unevenly distributed harms.
Reflections and Recommendations
Guiding Questions
During the course of our research, a number of common questions emerged about the nature of the predictive hiring tools we analyzed. We found ourselves needing to answer these questions before we could even begin to think about the equity implications of a given tool.
What is the tool predicting, and about whom? Hiring tools aim to predict very different things. For example, some tools try to predict an applicant’s likely performance in a given job, while others predict recruiters’ preferences or an internet user’s likelihood of clicking on an ad. Different kinds of bias can emerge depending on the specific predictive goal.
What data does the tool use to make predictions? Hiring tools are only as good as the data they are built from. As described above, the nature and quality of training data for predictive tools can vary, ranging from click patterns, to historical application data, to past hiring decisions, to performance evaluations and productivity measures. Each data source can present unique and challenging bias issues. The models built upon these data are used to evaluate a range of inputs and can be applied to anything from resume text, to game play, to facial expressions. Some of these inputs can violate social norms, reflect immutable characteristics, or lack apparent causal relationship with job performance.
Does the tool’s behavior change dynamically in response to user interactions? Some hiring tools are infrequently updated, while others are more dynamic, relying on real-time feedback to update underlying models. This distinction matters because static tools can offer more opportunity for reflection, auditing, and review before deployment. More dynamic tools, such as those powering advertising and matching platforms, are more likely to absorb bias arising through human behaviors and can be more difficult to study and monitor.
How does a tool communicate its predictions, and how are its users likely to understand them? Predictive hiring tools can produce numerical scores, rank candidates, and display a range of other results. Because hiring tools are typically billed as aids for human decisionmakers, it is important to carefully consider how people—whether recruiters or applicants—might understand and be influenced by these outputs.
What specific steps is a vendor taking to detect and address different kinds of bias in its tools? Hiring technology vendors frequently claim that they audit and address bias within the tools they create. But they seldom offer details or make available the results of independent evaluations, at least publicly. Given the absence of formal best practices in this area, and the different kinds of biases to be addressed, vendors should be expected to provide details about their procedures. What method is the vendor using to measure for “bias” and for what categories of people? How does the vendor go about “removing” these effects? Is the vendor’s process transparent, public, and externally audited?
Will this tool help an organization discover patterns of bias in its hiring practices? Sometimes, predictive hiring tools can be used to help reveal and measure biases that exist within an existing workforce or applicant flow, rather than imposing predictions on candidates. Employers should be encouraged to use analytical and predictive tools for reflection and analysis before deploying, or at least alongside deployments of, tools used to facilitate the hiring process itself, so that steps can be taken to address existing disparities.
Reflections
Too often, the precise role of predictive technologies in hiring is oversimplified by vendors and popular commentators. Hiring technologies play dramatically different roles at different stages of the hiring process, and present different kinds of risks and benefits. More specifically:
Hiring is rarely a single decision point, but rather a cumulative series of small decisions. Predictive technologies can play very different roles throughout the hiring funnel, from determining who sees job advertisements, to estimating an applicant’s performance, to forecasting a candidate’s salary requirements. Understanding how these technologies work, and their specific roles within the hiring process, is critical to addressing their potential impacts on equity.
While new hiring tools rarely make affirmative hiring decisions, they often automate rejections. Much of this activity happens early in the hiring process, when job opportunities are automatically surfaced to some people and withheld from others, or when candidates are deemed by a predictive system not to meet the minimum or desired qualifications needed to move further in the application process.
Predictive hiring tools can reflect institutional and systemic biases, and removing sensitive characteristics is not a solution. Predictions based on past hiring decisions and evaluations can both reveal and reproduce patterns of inequity at all stages of the hiring process, even when tools explicitly ignore race, gender, age, and other protected attributes.
Nevertheless, vendors’ claim that technology can reduce interpersonal bias should not be ignored. Bias against people of color, women, and other underrepresented groups has long plagued hiring, but with sufficient deliberation, transparency, and oversight, some new hiring technologies might be poised to help improve on this poor baseline.
Even before people apply for jobs, predictive technology plays a powerful role in determining who learns of open positions. Employers and vendors are sourcing tools, like digital advertising and personalized job boards, to proactively shape their applicant pools. These technologies are outpacing regulatory guidance, and are exceedingly difficult to study from the outside.
Hiring tools that assess, score, and rank jobseekers can overstate marginal or unimportant distinctions between similarly qualified candidates. In particular, rank-ordered lists and numerical scores may influence recruiters more than we realize, and not enough is known about how human recruiters act on predictive tools’ guidance.
Recommendations
A lack of transparency and outdated legal and regulatory guidance have made effective enforcement of antidiscrimination laws difficult in the age of predictive technology. At the same time, the growing popularity and collateral risk of these technologies demands attention. We offer the following preliminary recommendations:
Vendors and employers must be dramatically more transparent about the predictive tools they build and use, and must allow independent auditing of those tools. Employers should disclose information about the vendors and predictive features that play a role in their hiring process. Vendors should take active steps to detect and remove bias in their tools. They should also provide detailed explanations about these steps, and allow for independent evaluation. Without this level of transparency, regulators and other watchdogs have no practical way to protect jobseekers or hold responsible parties accountable.
The EEOC should begin to consider new regulations that interpret Title VII in light of predictive hiring tools. At bare minimum, the agency should issue a report that further explores these issues, including a candid reflection on the capacity of the Uniform Guidelines to account for modern hiring technology, and make recommendations for further action. (The Commission held one public meeting on the subject in 2016, but there has been little public action since.)
Regulators, researchers, and industrial-organizational psychologists should revisit the meaning of “validation” in light of predictive hiring tools. In particular, the value of correlation as a signal of “validity” for antidiscrimination purposes should be vigorously debated. These deliberations could help inform future regulatory guidance and corporate best practices.
Digital sourcing platforms must recognize their growing influence on the hiring process and actively seek to mitigate bias. Ad platforms and job boards that rely on dynamic, automated systems should be further scrutinized—both by the companies themselves, and by outside stakeholders. These systems tend to be more dynamic and complex than models used for assessment, and lag behind in efforts to measure and address bias. This stage of the hiring process is often overlooked and requires substantially more study and consideration.
Conclusion
Legal scholars have aptly noted that “although algorithms offer the potential for avoiding or minimizing bias, the real question is how the biases they may introduce compare with the human biases they avoid." Our research did not convince us that sufficient safeguards yet exist to ensure this balance will tip in favor of equity.
Because of the inherent weaknesses in nearly all workforce data, predictive hiring tools are prone to be biased by default. Legal and regulatory protections from technology-enabled discriminatory recruitment practices remain largely untested, and in the worst case, they are unsuited to contend with the sort of predictive tools described in this report. Stakeholders are flying blind when it comes to assessing fairness and equity. Jobseekers have little visibility into the tools that are being used to assess them. Employers can have little insight into how their vendors’ proprietary tools actually work. Regulators lack the legal authority, resources, and expertise needed to oversee the growing landscape of predictive hiring technologies. Moreover, modern predictive tools do not fit neatly into established understandings of employment law concepts.
But the picture is not entirely grim: Vendors have rolled out some promising features that reflect at least some awareness of the deep and systemic inequalities that continue to distort hiring dynamics. Measures like these could ultimately help pull hiring technologies in a more constructive direction, but much more work is needed.
Vendors are rapidly releasing new features, retiring old ones, and addressing flaws. Our hope is that by using detailed and specific examples to examine the equities and biases of predictive hiring products, we have highlighted common issues that remain unaddressed and unresolved—despite others’ calls for care and caution. We urge advocates, lawmakers, employers, and other stakeholders to confront the emerging questions posed by predictive hiring technologies, articulate principles for their responsible use, and take concrete steps to update regulatory frameworks accordingly.
About This Report
About the Authors
Miranda Bogen is a Senior Policy Analyst at Upturn. She holds a Master’s degree in Law and Diplomacy with a focus on international technology policy from The Fletcher School of Law and Diplomacy at Tufts, and bachelor’s degrees in Political Science and Middle Eastern & North African Studies from UCLA.
Aaron Rieke is a Managing Director at Upturn. He holds a JD from Berkeley Law, with a Certificate of Law and Technology, and a BA in Philosophy from Pacific Lutheran University.
Upturn is a 501(c)(3) nonprofit organization based in Washington, DC that promotes equity and justice in the design, governance, and use of digital technology.
Corrections
2019-02-15: Clarified that Textio’s job description software relies on models specific to particular contexts, such as the industry and location of jobs.
Acknowledgements
Many thanks to Ifeoma Ajunwa, Solon Barocas, Rumman Chowdhury, Fiona Dale, Natasha Duarte, Kate Glazebrook, Tanya Goldman, Rachel Goodman, Angela Hanks, Jennifer Kim, Jon Kleinberg, Logan Koepke, Karen Levy, Hannah Masuga, Hanna McCloskey, Michelle Miller, Aiha Nguyen, David Robinson, Dariely Rodriguez, Galen Sherwin, Emma Weil, Harlan Yu, Jenny Yang, the Cornell AI Policy and Practice group and others for their helpful input on the structure and content of this report.
1
A November 2016 survey from the Society for Human Resource Management found that 53 percent of HR departments use “big data” to make decisions, most often for recruitment and selection purposes. Jobs of the Future: Data Analysis Skills, SHRM Survey Findings, November 2018, https://www.shrm.org/hr-today/trends-and-forecasting/research-and-surveys/Documents/Data-Analysis-Skills.pdf. This number has almost certainly increased since then.
1
A 2018 survey of 9000 recruiters found that 64 percent “use data at least ‘sometimes’” in the course of their recruitment activity; 79 percent are likely to do so in the next two years, and 76 percent believe artificial intelligence will have a significant impact on recruiting. LinkedIn Global Recruiting Trends 2018, https://business.linkedin.com/content/dam/me/business/en-us/talent-solutions/resources/pdfs/linkedin-global-recruiting-trends-2018-en-us.pdf. Sixty percent of technology companies plan to invest in AI-powered recruiting software in 2018. Entelo 2018 Recruiting Trends Report, December 14, 2018, available at http://resources.entelo.com/download-2018-entelo-recruiting-trends-report.
1
Alex P. Miller, Want Less-Biased Decisions? Use Algorithms., Harvard Business Review, July 26, 2018, https://hbr.org/2018/07/want-less-biased-decisions-use-algorithms.
1
Gideon Mann and Cathy O’Neil, Hiring Algorithms Are Not Neutral, Harvard Business Review, December 9, 2016, https://hbr.org/2016/12/hiring-algorithms-are-not-neutral; Joy Buolamwini, When the Robot Doesn’t See Dark Skin, The New York Times, June 21, 2018, https://www.nytimes.com/2018/06/21/opinion/facial-analysis-technology-bias.html.
1
For a more detailed early history and sociology of hiring technology platforms, see Ifeoma Ajunwa and Daniel Green, Platforms at Work: Automated Hiring Platforms and Other New Intermediaries in the Organization of Work, Research in the Sociology of Work (forthcoming), 2018 at 21-27.
1
Erin Olsen, Online Labor Exchanges and Advanced Job Matching: An Evaluation of Vendors and Opportunities, RealTime Talent, August 2016, http://www.realtimetalent.org/wp-content/uploads/2016/09/RTT_2016_August_MN_OnlineLaborExchange_Special.pdf at 6.
1
These are also often called “job aggregators.” See Job Aggregator, Hiring Success Glossary, SmartRecruiters, https://www.smartrecruiters.com/resources/glossary/job-aggregator.
1
Loren Baker, Indeed.com Launches Job Search PPC Advertising Network, Search Engine Journal, May 15, 2006, https://www.searchenginejournal.com/indeedcom-launches-job-search-ppc-advertising-network/3420/; History of Job Advertising: From Window Signs to Programmatic Recruitment, Perengo, March 21, 2017, http://blog.perengo.com/programmatic/history-of-job-advertising-intro.
1
Testimony of Dr. Eric Dunleavy, Equal Employment Opportunity Commission Meeting on Big Data in the Workplace, October 13, 2016, available at https://www.eeoc.gov/eeoc/meetings/10-13-16/transcript.cfm (describing how “[a]pplicants are easier to reach today, and can apply to many jobs from anywhere. In part because of this situation, automated steps at the front end of a hiring process may be particularly useful, given the large size of internet-based applicant pools and the human capital effort required to evaluate those applicants on eligibility and qualifications.”). For instance, Google receives roughly two million applications per year for several thousand open positions. Richard Feloni, Google’s former HR boss shared the company’s 4 rules for hiring the best employees, Business Insider, March 8, 2018, http://www.businessinsider.com/how-google-hires-exceptional-employees-2016-2. It is estimated that applications from online job boards receive a 1-4 percent average response rate. Olsen, supra note 6. Some early vendors also offered kiosks to facilitate, process, and screen job applications, especially for retail and other hourly positions. Ajunwa and Green, supra note 5 at 23.
1
Linda Barber, e-Recruitment Developments (2006), Institute for Employment Studies, https://www.employment-studies.co.uk/system/files/resources/files/mp63.pdf at 11.
1
This class of technology is commonly referred to by the acronym “ATS.”
1
By 2010, LinkedIn had 90 million users; by 2013 it passed 225 million, and it hit 500 million in 2017. A Brief History of LinkedIn, https://ourstory.linkedin.com (accessed October 7, 2018); Barb Darrow, LinkedIn Claims Half a Billion Users, Fortune, April 24, 2017, http://fortune.com/2017/04/24/linkedin-users.
1
Michael Overell, The History of Innovation in Recruitment Technology and Services, TechCrunch, October 29, 2016, https://techcrunch.com/2016/10/29/the-history-of-innovation-in-recruitment-technology-and-services.
1
One such sourcing platform boasts that it offers access to more than 450 million profiles. Gaurav Kataria, Introducing Entelo Insights, Entelo Blog, May 1, 2018, https://blog.entelo.com/introducing-entelo-insights.
1
Psychometric intelligence tests, rooted in the same cognitive theories that motivated the eugenics movement, gained traction during World War I as a tool to assess drafted soldiers and were repurposed after the war as tools for “industrial psychology.” See generally Craig Haney, Employment Tests and Employment Discrimination: A Dissenting Psychological Opinion, Berkeley Journal of Employment & Labor Law 5(1), June 1982, https://scholarship.law.berkeley.edu/cgi/viewcontent.cgi?referer=https://www.google.com/&httpsredir=1&article=1071&context=bjell. Intelligence testing also played a significant role as a justification to restrict immigration to the U.S. Haney, id. at 8 (“Intelligence tests administered at the Ellis Island receiving station in New York in 1912 had already ‘documented’ the fact that fully four-fifths or more of the Jews, Hungarians, Italians, and Russians entering this country were ‘feeble-minded.’”). But the idea of assessing potential workers is far older: China began using tests to identify talent since well before 500 BC. John Rust and Susan Golombok, Modern Psychometrics, Third Edition: The Science of Psychological Assessment, 2014 at 4.
1
While these terms of art are popular among employers and others to describe practices intended to increase the representation of minority and marginalized groups in workplaces, we will mostly refrain from using them in the remainder of this report in order to emphasize that equitable hiring should not be a goal held separate from companies’ core hiring process. See Anna Holmes, Has ‘Diversity’ Lost Its Meaning?, The New York Times Magazine, October 27, 2015, https://www.nytimes.com/2015/11/01/magazine/has-diversity-lost-its-meaning.html.
1
For a detailed discussion on diversity and inclusion technology, see Stacia Sherman Garr and Carole Jackson, Diversity and Inclusion Technology: Could this be the Missing Link?, RedThread Research and Mercer, September 11, 2018.
1
Rewriting the rules for the digital age: 2017 Deloitte Global Human Capital Trends, https://www2.deloitte.com/content/dam/Deloitte/global/Documents/About-Deloitte/central-europe/ce-global-human-capital-trends.pdf at 40-41.
1
Britain’s Royal Society, “Machine learning: the power and promise of computers that learn by example,” April 2017, https://royalsociety.org/~/media/policy/projects/machine-learning/publications/machine-learning-report.pdf.
1
Hiring technology has closely followed trends from commercial marketing and sales contexts. Applicant management systems mirrored customer relationship management systems that companies often used to manage sales. Employers mimicked brands in embracing social networking sites as a channel for engagement with potential applicants. And recruitment advertising tools adopted the payment structures and programmatic ad services honed for consumer marketing. Deloitte, supra note 18.
1
LinkedIn Global Recruiting Trends 2017, https://www.slideshare.net/pedrooolito/linkedin-global-recruiting-trends-report-2017 (finding that time to hire is the second most important way recruiters measure success).
1
2016 Human Capital Benchmarking Report, Society for Human Resource Management, November 2016, https://www.shrm.org/hr-today/trends-and-forecasting/research-and-surveys/Documents/2016-Human-Capital-Report.pdf.
1
Positions that require specialized education, skills or training—particularly STEM positions—take longer to fill than other roles. Jonathan Rothwell, Still Searching: Job Vacancies and STEM Skills, Metropolitan Policy Program at Brookings, July 2014, https://www.brookings.edu/wp-content/uploads/2014/07/Job-Vacancies-and-STEM-Skills.pdf.
1
According to LinkedIn, 57 percent of companies see competition for talent as a top challenge for their hiring teams. LinkedIn (2017), supra note 21.
1
For example, retailer Sears adds more than 150,000 workers during the holidays, so the company pushed hard to reduce the duration of its hiring process from three months to 35 days. Glenn Rifkin, Big Data, Predictive Analytics, and Hiring, Korn Ferry Institute Briefings Magazine, May 12, 2014, https://www.kornferry.com/institute/big-data-predictive-analytics-and-hiring.
1
2016 Human Capital Benchmarking Report, supra note 22.
1
LinkedIn (2017), supra note 21.
1
The precise composition of this metric varies by both company and industry. For example, Teach for America, which gets tens of thousands of applications each year, looks carefully at which applicants make it through the hiring process as well as their assessments during the course of the program. The organization uses that data to inform which candidates are invited to phone or on-site interviews. Marykate Zukiewicz, Melissa A. Clark, and Libby Makowsky, Implementation of the Teach For America Investing in Innovation Scale-Up, Mathematica Policy Research, March 2015 (describing how “a mathematical selection model helps guide decisions about whether applicants will progress to the next stage. This model, which TFA updates annually, uses recruitment, selection, and student achievement data from previous cohorts of corps members to determine the factors associated with corps member effectiveness and then uses these factors to predict the effectiveness of each new applicant.”).
1
Christine Porath, How to Avoid Hiring a Toxic Employee, Harvard Business Review, February 3, 2016, https://hbr.org/2016/02/how-to-avoid-hiring-a-toxic-employee; Melody Wilding, How to spot toxic employees before you hire them, Quartz, January 5, 2018, https://qz.com/work/1172945/how-to-spot-toxic-employees-before-you-hire-them.
1
See, e.g., Kiera Abbamonte, How to Put Together a Loss Prevention Plan for Your Store, Shopify Blogs, April 19, 2018, https://www.shopify.com/retail/retail-loss-prevention (urging retailers to “tak[e] loss prevention into account during the hiring and training processes” by “screening for conscientious candidates who conduct themselves with integrity” because “[e]mployees who excel in those areas are partners in the loss prevention fight. They’re less likely to abuse their power as employees and more invested in a retailer’s success. They’re more dedicated to helping you reduce retail shrinkage.”).
1
See, e.g., John Sullivan, A Recruiting Strategy to Counter the Threat of Unions and the EFCA, ERE, January 26, 2009, https://www.ere.net/a-recruiting-strategy-to-counter-the-threat-of-unions-and-the-efca. It is technically illegal to refuse to hire union “salts,” or labor organizers who apply for jobs in nonunion companies with the intent to form a union. NLRB v. Town & Country Electric, 516 US 85 (1995); see also Erik Forman, Let’s Get to Work, Jacobin, February 7, 2017, https://www.jacobinmag.com/2017/02/labor-unions-workers-salts-students-organizing. However, legal protections for such organizers have been eroded in subsequent rulings. Nick Johnson, No Salt Added, Jacobin, March 22, 2017, https://jacobinmag.com/2017/03/salts-union-organizing-nlrb-obama-trump-labor.
1
See, e.g., LinkedIn (2017), supra note 21 (finding that “the length of time new hires stay at a company” is the top way recruiters measure success); Use of Workforce Analytics for Competitive Advantage, Society for Human Resource Management Foundation, May 2016, https://www.shrm.org/foundation/ourwork/initiatives/preparing-for-future-hr-trends/Documents/Workforce%20Analytics%20Report.pdf at 24 (explaining that Nielson uses first-year retention as a key metric to judge whether a hire was successful); Mitchell Hoffman, Lisa B. Kahn, and Danielle Li, Discretion in Hiring, NBER Working Paper 21709, September 2017, http://www.nber.org/papers/w21709.pdf (in which the researchers used job tenure as a signal to determine whether firms that used job testing and minimized human discretion in the hiring process ended up with “better” hires).
1
And a profitable one: Credit Suisse estimated that a 1 percent increase in retention could save $75-100 million per year. Rachel Emma Silverman and Nikki Waller, The Algorithm That Tells the Boss Who Might Quit, The Wall Street Journal, March 13, 2015, https://www.wsj.com/articles/the-algorithm-that-tells-the-boss-who-might-quit-1426287935.
1
The cost of replacing employees is estimated to be roughly 20 percent of departing employees’ annual salary. For highly paid and executive level positions, the cost can exceed 200 percent of the annual salary. Heather Boushey and Sarah Jane Glynn, There Are Significant Business Costs to Replacing Employees, Center for American Progress, November 16, 2012, https://www.americanprogress.org/wp-content/uploads/2012/11/CostofTurnover.pdf.
1
According to LinkedIn, a significant majority of talent acquisition professionals around the world see diversity affecting how they hire. LinkedIn Global Recruiting Trends 2018, available at https://business.linkedin.com/talent-solutions/recruiting-tips/2018-global-recruiting-trends. Employers may also be attentive to the improved financial performance, enhanced innovation, and stronger organizational culture that come with more diverse and inclusive teams. See, e.g., Sylvia Ann Hewlett, Melinda Marshall, and Laura Sherbin, How Diversity Can Drive Innovation, Harvard Business Review, December 2013, https://hbr.org/2013/12/how-diversity-can-drive-innovation; Vivian Hunt, Dennis Layton, and Sara Prince, Why Diversity Matters, McKinsey, January 2015, https://www.mckinsey.com/business-functions/organization/our-insights/why-diversity-matters. Employers, particularly in the technology industry, have recently found themselves subject to scorching public and government pressure over poor progress on workforce diversity. Letter from the Congressional Black Caucus to NCTA - The Internet & Television Association and US Telecom - The Broadband Association, February 22, 2018, available at https://www.scribd.com/document/373547119/CBC-Diversity-Letter-2-22-18-1 (accessed October 7, 2018). Some companies set explicit diversity goals and publish results, relying heavily on recruiters and managers to drive improvement in diversity metrics over time. See, e.g., Walmart 2015 Diversity & Inclusion Report, https://cdn.corporate.walmart.com/01/8b/4e0af18a45f3a043fc85196c2cbe/2015-diversity-and-inclusion-report.pdf; @janetvh, We’re committing to a more diverse Twitter, Twitter Blog, August 28, 2015, https://blog.twitter.com/official/en_us/a/2015/we-re-committing-to-a-more-diverse-twitter.html. A few go even further, tapping recruiters and hiring teams to focus specifically on finding diverse candidates, or linking manager compensation to the company’s performance against its goals. See, e.g., Heather Clancy, How LinkedIn embeds diversity goals into day-to-day management, Fortune, October 20, 2015, http://fortune.com/2015/10/20/linkedin-compensation-diversity; Sodexo—Making Every Day Count: Driving Business Success Through The Employee Experience, Catalyst Knowledge Center, January 1, 2012, http://www.catalyst.org/knowledge/sodexo-making-every-day-count-driving-business-success-through-employee-experience.
1
However, without strong commitments, the long-term benefits of a diverse workforce can come into tension with short term time and monetary costs. The Business Case and Challenges of Workforce Diversity, Allegis Group, March 28, 2018, https://www.allegisgroup.com/en/insights/blog/2018/march/business-case-challenges-diversity (finding that despite compelling evidence of economic, productivity, and innovation benefit, “[a] significant portion of hiring managers were either somewhat or strongly concerned about issues related to attracting quality talent (23 percent), filling positions quickly (39 percent), and optimizing costs (27 percent).”).
1
A How-To Guide for Using A Recruitment Chatbot, Ideal, https://ideal.com/recruitment-chatbot/ (accessed October 7, 2018) (“It’s estimated that 65% of resumes received for a role are ignored. By interacting with this ignored 65% of candidates, a chatbot is doing the tasks that already time-strapped human recruiters don’t have the time nor capacity to do in the first place.”).
1
For an overview of the variety of cognitive biases that play a role in the hiring process, see Theo Fellgett, Cognitive biases in hiring — a cheat sheet, Finding Needles in Haystacks, July 25, 2017, https://medium.com/finding-needles-in-haystacks/cognitive-biases-in-hiring-a-cheat-sheet-689bd2571b04.
1
See, e.g., Marianne Bertrand and Sendhil Mullainathan, Are Emily and Greg more employable than Lakisha and Jamal? A field experiment on labor market discrimination, American Economic Review 94, 2004 (finding that racial discrimination is still a prominent feature of the labor market); Devah Pager and Bruce Western, Identifying Discrimination at Work: The Use of Field Experiments, The Journal of Social Issues, 68(2) (finding that Blacks are less than half as likely to receive consideration by employers relative to equally qualified Whites across a wide range of low-wage jobs, and also noting that in the field, audit experiments offer a clean experimental method design with which to assess causal effects); Katherine B. Coffman, Christine L. Exley, and Muriel Niederle, When gender discrimination is not about gender, Harvard Business School Working Paper No. 18-054, August 1, 2018, (finding ample evidence discrimination against women in hiring, but finds that this discrimination is “not driven by gender-specific animus”); Claudia Goldin and Cecilia Rouse, Orchestrating Impartiality: The Impact of ‘Blind’ Auditions on Female Musicians, American Economic Review 90(4), September 2000 (observing in 2000 that “few researchers have been able to address directly the issue of bias in hiring practices,” but demonstrating that “the switch to blind auditions can explain 30 percent of the increase in the proportion female among new hires and possibly 25 percent of the increase in the percentage female in the orchestras from 1970 to 1996”); Shelley J. Correll, Stephen Benard, and In Paik, Getting a Job: Is There a Motherhood Penalty?, American Journal of Sociology 112(5), March 2007 (finding that female job applicants are penalized for being mothers, while otherwise identical male job applicants are rewarded for being fathers); Monica Biernat and Diane D. Kobrynowicz, Gender and race‐based standards of competence: Lower minimum standards but higher ability standards for devalued groups, Journal of Personal and Social Psychology 72, 1997 (finding that that African‐American job applicants were held to even stricter standards of “competence” than white applicants).
1
Lincoln Quillian, Devah Pager, Ole Hexel, and Arnfinn H. Midtbøen, Meta-analysis of field experiments shows no change in racial discrimination in hiring over time, PNAS 114(41), October 10, 2017 (a meta-analysis which looked at every available field experiment on hiring discrimination from 1989 through 2015, found that “white applicants receive 36% more callbacks than equally qualified African Americans” while “[w]hite applicants receive on average 24% more callbacks than Latinos.” Studies included both resume audits—where fictitious resumes with distinctly racial names are submitted—as well as in-person audits—where racially dissimilar but otherwise similar pairs of trained testers apply for jobs. Notably, comparing audit study results from 1975 to 2015, the authors find no evidence of change over time in rates of hiring discrimination with respect to African Americans.).
1
E.g. Joseph Zappa, Structural bias poses obstacles to faculty of color, The Brown Daily Herald, December 5, 2014, http://www.browndailyherald.com/2014/12/05/structural-bias-poses-obstacles-faculty-color/ (describing how “[b]ecause fewer people of color—particularly underrepresented minorities—complete doctoral studies than whites, there are fewer candidates of color for assistant professorships and even fewer for more advanced academic positions,” which, compounded with unconscious bias and competing priorities during the search process leads to fewer mentors and champions for younger faculty of color.)
1
While others have used “institutional” discrimination to also refer to societal forces of inequity, we distinguish the two here to highlight the role of individual organizations. See, e.g., P.J. Henry, Institutional Bias, in John F. Dovidio, Victoria M. Esses and Miles Hewstone, The Sage Handbook of Prejudice, Stereotyping and Discrimination (2010), available at https://pdfs.semanticscholar.org/6e97/22aded84469a394b60c63ce2ff7acd0af881.pdf. Others have described the three levels of discrimination as individual, organizational, and societal. See, e.g., Devah Pager and Hana Shepherd, The Sociology of Discrimination: Racial Discrimination in Employment, Housing, Credit, and Consumer Markets, Annual Review of Sociology (2009).
1
E.g. Kathleen Davis, The One Word Men Never See In Their Performance Reviews, FastCompany, August 27, 2014, https://www.fastcompany.com/3034895/the-one-word-men-never-see-in-their-performance-reviews.
1
See, e.g., Ariel Jao and Associated Press, Segregation, school funding inequalities still punishing Black, Latino students, NBC News, January 12, 2018, https://www.nbcnews.com/news/latino/segregation-school-funding-inequalities-still-punishing-black-latino-students-n837186; Laura A. Rivera, Ivies, extracurriculars, and exclusion: Elite employers’ use of educational credentials, Research in Social Stratification and Mobility 29(1), January 2011, https://www.sciencedirect.com/science/article/pii/S027656241000065X (“Contrary to common sociological measures of institutional prestige, employers privileged candidates who possessed a super-elite (e.g., top four) rather than selective university affiliation…firms performed a strong secondary screen on candidates’ extracurricular accomplishments, favoring high status, resource-intensive activities that resonated with white, upper-middle class culture.”).
1
Jeremy Ashkenas, Haeyoun Park, and Adam Pearce, Even With Affirmative Action, Blacks and Hispanics Are More Underrepresented at Top Colleges Than 35 Years Ago, The New York Times, August 24, 2017, https://www.nytimes.com/interactive/2017/08/24/us/affirmative-action.html.
1
See, e.g., Schuette v. Coalition to Defend Affirmative Action, Integration and Immigrant Rights, and Right for Equality by Any Means Necessary 572 U.S. ___ (2014) (6-2) (Sotomayor, S., dissenting) (explaining that “[t]he way to stop discrimination on the basis of race is to speak openly and candidly on the subject of race, and to apply the Constitution with eyes open to the unfortunate effects of centuries of racial discrimination.”).
1
E.g. Donna K. Bivens, What Is Internalized Racism?, in Flipping the Script: White Privilege and Community Building (Maggie Potapchuck 2005), http://www.racialequitytools.org/resourcefiles/What_is_Internalized_Racism.pdf.
1
In fact, Kimberlé Crenshaw coined the term “intersectionality” in a law review article critiquing single-dimensional analysis used in a number of employment discrimination cases brought under Title VII. Kimberlé Williams Crenshaw, Demarginalizing the Intersection of Race and Sex: A Black Feminist Critique of Antidiscrimination Doctrine, Feminist Theory, and Antiracist Politics, 1989 University of Chicago Legal Forum 1 (1989), https://chicagounbound.uchicago.edu/cgi/viewcontent.cgi?article=1052&context=uclf.
1
See, e.g., Anne B. Shaver, Intersectionality in Title VII Litigation: Plaintiffs’ Class Action Perspective, ABA Section of Labor and Employment Law Conference, November 10, 2017, https://www.americanbar.org/content/dam/aba/events/labor_law/2017/11/conference/papers/Shaver%20-%20Intersectionality.pdf.
1
See, e.g., Joy Buolamwini and Timnit Gebru, Gender Shades: Intersectional Accuracy Disparities in Commercial Gender Classification, Proceedings of the 1st Conference on Fairness, Accountability and Transparency, PMLR (2018).
1
Friedman and Nissenbaum define three main types of bias: preexisting bias, technical bias, and emergent bias. Batya Friedman and Helen Nissenbaum, Bias in computer systems, ACM Transactions on Information Systems (TOIS) TOIS Homepage archive, Volume 14 Issue 3, July 1996.
1
Dave Gershgorn, Companies are on the hook if their hiring algorithms are biased, Quartz, October 22, 2018, https://qz.com/1427621/companies-are-on-the-hook-if-their-hiring-algorithms-are-biased.
1
See generally Solon Barocas and Andrew Selbst, Big Data’s Disparate Impact, 104 Calif. L. Rev. 671 (2016), http://www.californialawreview.org/wp-content/uploads/2016/06/2Barocas-Selbst.pdf.
1
The company Xerox (working with third party vendor Evolv, which has since been acquired by enterprise HR software provider Cornerstone OnDemand) ended up removing the variable from its model. Joseph Walker, Meet the New Boss: Big Data, Wall Street Journal, September 20, 2012, https://www.wsj.com/articles/SB10000872396390443890304578006252019616768.
1
For a seminal discussion on the potential issues of relying on disparate impact when machine learning is used in the context of hiring, see Barocas and Selbst, supra note 53.
1
See Raja Parasuraman & Victor Riley, Humans and Automation: Use, Misuse, Disuse, Abuse, 39 Hum. Factors 230 (1997). For example, one study found that recruiters with access to a decision aid tool tended to make decisions similar to those recommended. Alexander Thomas Jackson, Examining Factors Influencing Use of A Decision Aid in Personnel Selection, Dissertation, Department of Psychological Sciences College of Arts and Sciences, Kansas State University, May 2016 (finding that “when people are provided with the decision aid, their predictions were significantly more similar to (but not the same as) the predictions made by the aid than people who were not provided with the decision aid….This research also shows that when provided with a decision aid that has high validity, people will increase their reliance on the decision aid over multiple decisions.”).
1
For instance, recruiters’ behavior may be influenced by position bias, and end up focusing disproportionately on candidates presented at the top of a list. For early work on position bias, see Thorsten Joachims, Laura Granka, Bing Pan, Helene Hembrooke, and Geri Gay, Accurately interpreting clickthrough data as implicit feedback, In SIGIR ‘05, pages 154–161, ACM, 2005.
1
Shari Trewin, AI Fairness for People with Disabilities: Point of View, IBM Accessibility Research, November 26, 2018, https://arxiv.org/pdf/1811.10670.pdf (“For example, if five of our job applicants use assistive technologies such as a screen reader or magnifier, and the online test itself is not fully accessible, then long response times could lead to systematic exclusion of these five applicants using assistive technologies, even though their disability is not known.”)
1
E.g. Os Keyes, The Misgendering Machines: Trans/HCI Implications of Automatic Gender Recognition, Proceedings of the ACM on Human-Computer Interaction, Vol. 2, No. CSCW, Article 88, November 2018, https://dl.acm.org/citation.cfm?id=3274357. For similar critiques about the assignment of racial categories, see Sebastian Benthall and BruceD. Haynes, Racial categories in machine learning, in FAT* ’19: Conference on Fairness, Accountability, and Transparency (FAT* ’19), January 29–31, 2019, https://arxiv.org/pdf/1811.11668.pdf.
1
See, e.g., Pauline T. Kim, Data-Driven Discrimination at Work, William & Mary Law Review 58, 2017 at 865; Iris Bohnet, What Works: Gender Equality by Design, Harvard University Press, March 2016; Using technology to combat bias in hiring, MIT News, March 23, 2018, http://news.mit.edu/2018/mit-alumna-stephanie-lampkin-using-technology-to-combat-hiring-bias-blendoor-0323 (describing how hiring technology vendor Blendoor “tracks how candidates move through the interview process — noting when a candidate is eliminated or gets hired. The app then uses this information to better match candidates in the future and identify at what stage bias may have come into play.”). For instance, a Fortune investigation found that even after retail company Walmart tool steps to overhaul its career website to promote diversity, more than half of the 4,400 job postings used language that was more likely to attract male candidate, while 84 percent of director-level jobs used male-biased language. Stacey Jones and Grace Donnelly, Walmart’s New Jobs Approach Could Be Undermined by Gender Bias, April 4, 2017, http://fortune.com/2017/04/04/walmart-jobs-gender-bias.
1
Civil Rights Act of 1984 § 702, 42 U.S.C. § 2000e-2(a) (2012).
1
401 U.S.C 424, 429-39.
1
42 USC § 2000e.
1
The Age Discrimination in Employment Act of 1967 (ADEA), 29 U.S. Code § 623; Americans With Disabilities Act of 1990, 42 U.S.C, § 12112.
1
Under federal law, an employment agency is “any person regularly undertaking with or without compensation to procure employees for an employer or to procure for employees opportunities to work for an employer and includes an agent of such a person.” Civil Rights Act, 42 U.S.C. § 2000(e)(701)(c) (1964).
1
42 U.S. Code § 2000e-2(a)(2).
1
See, e.g., Employment Tests and Selection Procedures, The U.S. Equal Employment Opportunity Commission, September 23, 2010, https://www.eeoc.gov/policy/docs/factemployment_procedures.html (describing the prohibition of disparate treatment and disparate impact discrimination).
1
Equal Employment Opportunity Commission, https://www.eeoc.gov.
1
While the EEOC is empowered to investigate systemic discrimination, the majority of charges the EEOC files are individual complaints. In FY2017, the agency received 84,254 discrimination charges, and resolved 99,109 charges. Of the 184 lawsuits the agency filed, only 30 involved charges of systemic discrimination. EEOC Releases Fiscal Year 2017 Enforcement And Litigation Data, U.S. Equal Opportunity Employment Commission, January 25, 2018, https://www.eeoc.gov/eeoc/newsroom/release/1-25-18.cfm; see generally Pauline T. Kim, Addressing Systemic Discrimination: Public Enforcement and the Role of the EEOC, 95 Boston University Law Review 3, 2015, https://papers.ssrn.com/sol3/papers.cfm?abstract_id=2611761.
1
42 USC § 2000e.
1
Enforcement Guidance and Related Documents, Equal Employment Opportunity Commission, https://www.eeoc.gov/laws/guidance/enforcement_guidance.cfm.
1
Office of Federal Contract Compliance Programs, U.S. Department of Labor, https://www.dol.gov/ofccp.
1
Executive Order 11246 — Equal Employment Opportunity, Office of Federal Contract Compliance Programs (OFCCP), https://www.dol.gov/ofccp/regs/compliance/ca_11246.htm.
1
Frequently Asked Questions, Internet Applicant Recordkeeping Rule, Office of Federal Contract Compliance Programs, https://www.dol.gov/ofccp/regs/compliance/faqs/iappfaqs.htm.
1
State Laws on Employment-Related Discrimination, National Conference of State Legislatures, http://www.ncsl.org/research/labor-and-employment/discrimination-employment.aspx (accessed November 8, 2018); also see, e.g., Cities and Counties with Non-Discrimination Ordinances that Include Gender Identity, Human Rights Campaign, https://www.hrc.org/resources/cities-and-counties-with-non-discrimination-ordinances-that-include-gender (accessed November 8, 2018). On the other hand, some state laws have constrained affirmative action: California Proposition 209 amended the state constitution to prohibit public institutions from “discriminat[ing] against, or grant[ing] preferential treatment to, any individual or group on the basis of race, sex, color, ethnicity, or national origin in the operation of public employment, public education, or public contracting.” California Constitution Article I §31.
1
State Equal Pay Laws, National Conference of State Legislatures, August 23, 2016, http://www.ncsl.org/research/labor-and-employment/equal-pay-laws.aspx; Salary history bans, HRDive, August 24, 2018, https://www.hrdive.com/news/salary-history-ban-states-list/516662/; Salary history bans, HRDive, August 24, 2018, https://www.hrdive.com/news/salary-history-ban-states-list/516662/.
1
See, e.g., Nikoletta Bika, A recruiter’s guide to GDPR compliance, Workable, https://resources.workable.com/tutorial/gdpr-compliance-guide-recruiting.
1
Kim, supra note 60 at 916.
1
However, a number of vendors, especially larger and more established ones, are very aware of employers’ compliance needs, and build features to accommodate them. See, e.g., Compliance, Pymetrics: Using Neuroscience and Data Science to Revolutionize Talent Management (“Pymetrics”); Compliance In Recruiting: How Ideal’s Technology Prioritizes Compliance, Ideal, https://ideal.com/compliance/ (accessed November 10, 2018); Indeed Assessments - EEOC Statement, Indeed, https://www.indeed.com/assessments/eeoc (accessed November 10, 2018).
1
Pauline Kim and Sharion Scott, Discrimination in Online Employment Recruiting, St. Louis University Law Journal 63(1), 2019, https://papers.ssrn.com/sol3/papers.cfm?abstract_id=3214898 at 12.
1
U.S. v. City of Warren, Mich. 138 F.3f 1083 (6th Cir. 1998); U.S. v. Brennan, 650 F.3d 65 (2d Cir. 2011).
1
Uniform Guidelines on Employee Selection Procedures, http://www.uniformguidelines.com.
1
Id., §5(B)
1
Barocas and Selbst, supra note 53 and Kim, supra note 60, respectively. Additionally, many vendors claim their tools are proprietary, or create tools too complex to be clearly interpreted. Dallas Card, The “black box” metaphor in machine learning, July 5, 2017, https://towardsdatascience.com/the-black-box-metaphor-in-machine-learning-4e57a3a1d2b0.
1
See Kim, supra note 60 at 920 (“In order for claimants to diagnose whether statistical bias has infected an algorithm, they would need access to the training data and the underlying model. The claimants would have to trace how the data miners collected the data, determine what populations were sampled, and audit the records for errors. Conducting these types of checks for a dataset created by aggregating multiple, unrelated data sources containing hundreds of thousands of bits of information would be a daunting task for even the best-resourced plaintiffs. In addition, the algorithm’s creators are likely to claim that both the training data and the algorithm itself are proprietary information. Thus, if the law required complainants to prove the source of bias, they would face insurmountable obstacles. […] Because the harms are more diffuse, individuals will find it extremely difficult to detect when a biased algorithm has produced an adverse outcome and to understand what caused the model to be biased. Even if these obstacles are overcome, the appropriate remedy would be structural in nature—namely, an injunction to revise or eliminate use of a biased model.”)
1
At the EEOC, harassment cases can languish for years, The Associated Press, April 9, 2018, https://wtop.com/national/2018/04/at-the-eeoc-harassment-cases-can-languish-for-years.
1
Rebecca Wexler, Life, Liberty, and Trade Secrets: Intellectual Property in the Criminal Justice System, 70 Stanford Law Review 1343, 2018, https://papers.ssrn.com/sol3/papers.cfm?abstract_id=2920883. Employers may also claim that the personnel records used to construct the tools are confidential. Kim, supra note 60 at 863. Platforms often claim that they are not liable for the conduct of their users. See, e.g., Onuoha v. Facebook, Inc., Case No. 5:16-cv-06440-EJD, Defendant’s Motion to Dismiss (N.D. Cal. Apr. 3, 2017), (arguing that “[p]laintiffs have failed to allege any facts plausibly suggesting that Facebook, as opposed to certain unnamed third-party advertisers, engaged in any unlawful or discriminatory conduct. […] even if some advertisers violated Facebook’s policies and engaged in unlawful discrimination, Plaintiffs cannot show that Facebook may be held liable for their actions.”).
1
Some machine learning researchers also refer to hiring as a compound decision that takes the form of decision-making pipelines. Amanda Bower et al, Fair Pipelines, FAT/ML 2017, August 2017, https://arxiv.org/pdf/1707.00391.pdf.
1
These phases are not universally defined, but reflect common usage and practice within the talent acquisition industry and common perceptions of the hiring process among jobseekers. While these categories vary slightly from industry descriptions, they most clearly capture the purpose and particulars of decision-making that happens during the course of most hiring funnels.
1
We also do not deeply explore the myriad technologies employers are turning to in order to track and measure their workforces. Those interested in discussions of such technologies, their histories, and their social, policy, and legal implications can reference, among other resources, Ifeoma Ajunwa, Algorithms at Work: Productivity Monitoring Platforms and Wearable Technology as the New Data-Centric Research Agenda for Employment and Labor Law, 63 St. Louis University Law Journal ___ (2019, Forthcoming), https://papers.ssrn.com/sol3/papers.cfm?abstract_id=3247286; Karen E.C. Levy and Solon Barocas, Refractive Surveillance: Monitoring Customers to Manage Workers, International Journal of Communication 12, 2018, http://ijoc.org/index.php/ijoc/article/view/7041; Karen E.C. Levy, The Contexts of Control: Information, Power, and Truck-Driving Work, The Information Society, 31:160–174, 2015, http://www.karen-levy.net/wp-content/uploads/2016/08/The-Contexts-of-Control-Information-Power-and-Truck-Driving-Work.pdf.
1
Seventy percent of these technologies are provided by third party vendors. Deloitte, supra note 18 at 40. When asked by a speaker at a conference on recruitment automation in San Francisco in June 2018, a substantial number of recruiters admitted to getting more than five unsolicited pitches per week for new technology solutions.
1
Ajunwa and Green, supra note 5 at 22.
1
Employers use an average of 24 different recruiting technologies during the course of recruitment. Meaghan Kacsmar, Top Recruiting Statistics for 2018, iCims Hiring Insights, November 25, 2017, https://www.icims.com/hiring-insights/for-employers/article-top-recruiting-statistics-for-2018. That’s likely because older talent acquisition and human capital management software behemoths that many large companies use, like Oracle or Workday, can be notoriously slow to incorporate and release updates. This means even employers whose job application process is embedded primarily on those larger platforms may also turn to multiple new technology platforms to facilitate various recruitment activities. Deloitte, supra note 18 at 46. Most digital hiring tools offer employers the ability to integrate new tools with legacy, enterprise software systems, usually using APIs. For example, AI recruiting vendor ENGAGE offers “[o]ver 100 integrations supported out of the box including complex multi-step workflows.” Engage, https://www.engagetalent.com/solution (accessed October 7, 2018).
1
During the course of drafting this report alone, applicant tracking system Greenhouse introduced a predictive tool, Greenhouse Predict, to forecast candidate offer acceptance and new hire start dates and announced a partnership with IMB to integrate Watson AI and predictive analytics, Greenhouse competitor Smashfly launched a recruitment chatbot, Indeed.com launched a digital assessment tool, and AI video interviewing company HireVue acquired game-based assessment vendor MindX. Introducing Greenhouse Predicts: Machine-Learning Feature Live for All Customers, Greenhouse Blog, May 9, 2018, https://www.greenhouse.io/blog/introducing-greenhouse-predicts-machine-learning; Peter Weddle, TATech Global News Bulletin: HealthJobsNationwide launches a new site, Smashfly launches new career site management system & TalentInc. acquires ResumeRabbit, TATech, September 23, 2018, https://www.tatech.org/tatech-global-news-bulletin-healthjobsnationwide-launches-a-new-site-smashfly-launches-new-career-site-management-system-talentinc-acquires-resumerabbit; Elyse Mayer, Recruiting With Smashfly’s Emerson: A Dynamic Experience for Talent and TA Teams, August 13, 2018, http://blog.smashfly.com/2018/08/13/smashfly-launches-ai-recruiting-assistant-emerson/; New Skills-Based Screening Platform Aims to Democratize Hiring, Indeed Press Room, May 14, 2018, http://press.indeed.com/press/new-skills-based-screening-platform-aims-to-democratize-hiring/; HireVue Acquires MindX to Create a Robust AI-Based Talent Assessment Suite, HR Technologist, May 14, 2018, https://www.hrtechnologist.com/news/recruitment-onboarding/hirevue-acquires-mindx-to-create-a-robust-aibased-talent-assessment-suite.
1
Recognizing, of course, that not all employers want to attract new or external candidates; some employers may well have a favored candidate or type of candidate in mind and so share jobs in an intentionally obscure fashion.
1
As the labor market tightens, employers may try to tap candidate pools like former prisoners, the long-term unemployed, and women who have voluntarily left the workforce. See Don Lee, Tight job market is good for felons, people with disabilities and others who are hard to employ. But can it last?, Los Angeles Times, June 26, 2017, http://www.latimes.com/business/la-fi-hardcore-jobless-20170626-story.html; Sylvia Ann Hewlett and Carolyn Buck Luce, Off-Ramps and On-Ramps: Keeping Talented Women on the Road to Success, March 2005, https://hbr.org/2005/03/off-ramps-and-on-ramps-keeping-talented-women-on-the-road-to-success.
1
Danielle Gaucher, Justin Friesen, and Aaron C. Kay, Evidence That Gendered Wording in Job Advertisements Exists and Sustains Gender Inequality, Journal of Personality and Social Psychology, July 2011, https://www.hw.ac.uk/services/docs/gendered-wording-in-job-ads.pdf; Danielle Colliers and Charlotte Zhang, Can We Reduce Bias in the Recruiting Process and Diversify Pools of Candidates by Using Different Types of Words in Job Descriptions?, Cornell University ILR Collection, Fall 2016, https://digitalcommons.ilr.cornell.edu/cgi/viewcontent.cgi?article=1132&context=student. Job board company ZipRecruiter also found that job ads that used gendered keywords saw fewer applicants. Removing These Gendered Keywords Gets You More Applicants, ZipRecruiter Blog, September 19, 2016, https://www.ziprecruiter.com/blog/removing-gendered-keywords-gets-you-more-applicants.
1
The company estimates that high-scoring job posts are filled 17 percent faster that other postings, and also that they attract 25 percent more applicants who will make it through a company’s screening process, and 23 percent more female applicants. Tim Halloran, Better hiring starts with smarter writing, Textio Word Nerd, June 16, 2017, https://textio.ai/better-hiring-starts-with-smarter-writing-7ecd9a38ec64.
1
Textio, https://textio.com. Textio notes several examples of its purported impact on gender diversity: Johnson & Johnson saw a 9 percent increase (or 90,000) per year in the number of women applicants to science and technology roles. Tim Halloran, How Johnson & Johnson is adding 90,000 more women to their hiring pipeline, Textio Word Nerd, January 3, 2018, https://textio.ai/johnson-and-johnson-textio-video-d95c1480c601; Zillow saw a 12 percent increase in women who applied. Marissa Coughlin, Zillow Group drives inclusion with augmented writing, Textio Word Nerd, June 27, 2018, https://textio.ai/zillow-group-drives-inclusion-with-augmented-writing-a9fda3f0324f; Atlassian went from 10 percent female hires to 57 percent in the company’s technical graduate program. Tim Halloran, How Atlassian went from 10% female technical graduates to 57% in two years, Textio Word Nerd, December 12, 2017, https://textio.ai/atlassian-textio-81792ad3bfbf. Other vendors offering similar tools include TalVista, Applied, and Uncommon.co. The National Center for Women & Information Technology takes a more analog approach with a checklist. NCWIT Checklist for Reducing Unconscious Bias in Job Descriptions/Advertisements, https://www.ncwit.org/sites/default/files/resources/ncwitchecklist_reducingunconsciousbiasjobdescriptions.pdf; Entelo offers a similar service to ensure inclusive language is used in recruitment outreach emails. Elena Sigacheva, Implementing Your Diversity & Inclusion Initiatives at the Top of the Funnel, Entelo Blog, October 24, 2018, https://blog.entelo.com/diversity-and-inclusion-from-day-1.
1
Textio initially built its product using 240 million job posts, cross referencing them with hiring outcomes. As the co-founder explained to a Quartz columnist, “[a]s customers use Textio to write their content, they contribute their anonymized data—published job posts, applicant stats, and hiring outcomes—to the collective dataset.” Just a few words can increase female and minority job applicants by more than 20%, Quartz Ideas, July 11, 2017, https://qz.com/1023518/just-a-few-words-can-increase-female-and-minority-job-applicants-by-over-20. The company notes that it ingests an additional 10 million job posts and outcomes each month. Andy Johnson, Why the best job posts come from team effort, Textio Word Nerd, https://textio.ai/why-the-best-job-posts-come-from-team-effort-5f856524ea43.
1
Allie Hall, What is the Textio Score? Taking the subjectivity out of writing job descriptions, Textio Word Nerd, May 10, 2017, https://textio.ai/writing-a-great-job-description-textio-score-83b0eed0b8e8.
1
Like every tool we observed, Textio relies on binary notions of gender. For a discussion on the social implications of such a design choice, see Foad Hamidi, Morgan Klaus Scheuerman, and Stacy M. Branham, Gender Recognition or Gender Reductionism?: The Social Implications of Embedded Gender Recognition Systems, Proceedings of the 2018 CHI Conference on Human Factors in Computing Systems (April 2018), https://dl.acm.org/citation.cfm?doid=3173574.3173582.
1
Tim Halloran, Watch your (gender) tone, Textio Word Nerd, July 8, 2017, https://textio.ai/watch-your-gender-tone-2728016066ec.
1
Hall, supra note 101; Matt Abbot, “Textio adds new guidance for accounting, executive, hospitality, manufacturing and sales jobs,” Textio Word Nerd, June 5, 2017, https://textio.ai/textio-adds-new-guidance-for-accounting-executive-hospitality-manufacturing-and-sales-roles-945cc78be2d5, Tim Halloran, “The power of location in job posts,” Textio Word Nerd, July 31, 2017, https://textio.ai/the-power-of-location-in-job-posts-3129d185b8a4, Julie Yue, “The cultural power of words at F5,” Textio Word Nerd, October 24, 2018, https://textio.ai/the-cultural-power-of-words-at-f5-7deeb4381885.
1
Gaucher et al, supra note 97; Danielle Colliers and Charlotte Zhang, Can We Reduce Bias in the Recruiting Process and Diversify Pools of Candidates by Using Different Types of Words in Job Descriptions?, Cornell University ILR Collection, Fall 2016, https://digitalcommons.ilr.cornell.edu/cgi/viewcontent.cgi?article=1132&context=student.
1
See, e.g., Steve Bates, ‘Post and Pray’ Has Had Its Day, Society for Human Resource Management, December 18, 2015, https://www.shrm.org/resourcesandtools/hr-topics/talent-acquisition/pages/recruitment-advertising.aspx; Don’t Post and Pray—Control Your Job Posting Results, Recruiting.com, https://www.recruiting.com/blog/dont-post-pray-control-job-posting-results; Tim Sackett, “Post and Pray” Actually Takes More Effort Than Recruitment Marketing, November 7, 2017, http://fistfuloftalent.com/2017/11/post-pray-actually-takes-work-recruitment-marketing.html (accessed October 7, 2018); Dustin Robinson, The end of Post and Pray Recruiting, Hello Talent Blog, March 8, 2016, https://www.hellotalent.com/blog/moving-away-from-post-and-pray-recruiting.
1
See, e.g., Nikoletta Bika, How to advertise a job opening on pay-per-click job boards, Workable, https://resources.workable.com/tutorial/how-to-advertise-a-job-opening-on-pay-per-click-job-boards (accessed October 16, 2018); see also Premium Job Ads, Monster (accessed October 28, 2018) https://hiring.monster.com/recruitment/premium-job-ad.aspx?intcid=CTA_HP_PJA-LK (“With Monster Premium Job Ads each of your jobs gets automatically distributed to 500+ sites. We’ll also pull data from over 100 social sources and use it to target both active and passive candidates on Facebook, Instagram and Twitter who have the skill sets you’re looking for.”); Indeed Target Ads, https://www.indeed.com/hire/ita-apply (“Select who will see your ads based on their location, searches, and experience.”).
1
See, e.g., Google, AdWords Help, How keywords work, available at https://support.google.com/adwords/answer/1704371?hl=en; see also Google AdWords Help, “Understanding bidding basics,” https://support.google.com/adwords/answer/2459326?hl=en; Microsoft, Bing Ads, “Choosing keywords,” http://advertise.bingads.microsoft.com/en-us/cl/241/training/choosing-keywords.
1
For more detail about Facebook’s targeting options, see Aaron Rieke and Miranda Bogen, Leveling the Platform: Real Transparency for Paid Messages on Facebook, May 2018, https://www.upturn.org/reports/2018/facebook-ads. While Facebook bars advertisers from discriminating “against people based on personal attributes such as race, ethnicity, color, national origin, religion, age, sex, sexual orientation, gender identity, family status, disability, medical or genetic condition,” hundreds of companies have been accused of improperly targeting job ads by age on Facebook, and Facebook has been accused of facilitating and being complicit in such discrimination. Communications Workers of America v. T-Mobile US Inc, Case No. 5:17-cv-07232-BLF, available at https://www.onlineagediscrimination.com/sites/default/files/documents/og-cwa-complaint.pdf; National Fair Housing Alliance v. Facebook, Case No. 1:18-cv-02689, available at http://nationalfairhousing.org/wp-content/uploads/2018/03/NFHA-v.-Facebook.-Complaint-w-Exhibits-March-27-Final-pdf.pdf (in which the plaintiffs contend that “[…] Facebook has encouraged, endorsed, aided and abetted, and executed discriminatory age-restricted advertisements and recruiting on behalf of employers and other employment agencies, both in the past and in the present […] Upon information and belief, currently when employers want to recruit applicants for employment, Facebook performs nearly all of the necessary functions of an employment agency and marketing firm: Facebook helps the employer to create the ad; collects, develops and provides databases of information on Facebook users to employers so that such employers can know which individuals are looking for employment, know various types of information about those applicants, such as their age and gender, and exclude certain groups of people from their ad campaigns; coordinates with the employer to develop the recruitment, marketing and/or advertising strategy to determine which people will and will not receive the ads; delivers the ads to prospective applicants; collects payments for these services from the employer; informs the employer of the performance of the ad campaign with numerous data analytics; and retains copies of the ads and data related to them.”); see also Kim and Scott, supra note 80 at 5-6, 13, and 25.
1
See, e.g., Chris Forman, Programmatic Media: The Future of Recruitment Advertising, Recruiter.com, January 25, 2016, https://www.recruiter.com/i/programmatic-media-the-future-of-recruitment-advertising/.
1
See Rieke and Bogen, supra note 109; Unleashing LinkedIn’s Targeting Capabilities, 2017, [https://business.linkedin.com/content/dam/me/business/en-us/marketing-solutions/cx/2017/pdfs/linkedin-targeting-guide.pdf]. On job site Indeed, employers can target their ads by the type of job role and location, and the platform uses candidates’ search history and resume information to determine which users should be targeted for a given target job title or keywords. Taylor Meadows, Target, Reach and Engage with Job Candidates Using Indeed Targeted Ads | Brand, Indeed Blog, October 31, 2018, http://blog.indeed.com/2018/10/31/how-to-use-indeed-targeted-ads-brand. |
1
On Facebook, this is called “custom audiences.” On LinkedIn, advertisers can use the “Matched Audience” feature. AJ Wilcox, LinkedIn’s new Matched Audiences feature just blew Facebook Custom Audiences out of the water for B2B, Marketing Land, April 24, 2017, [https://marketingland.com/linkedins-new-matched-audiences-feature-just-blew-facebook-custom-audiences-water-b2b-212213].
1
Audience Expansion - Overview, LinkedIn Marketing Solutions Help, [https://www.linkedin.com/help/lms/topics/8169/8179/51626] (accessed November 9, 2018) (“Audience Expansion allows you, as an advertiser, to increase the reach of your campaign by showing your ads to audiences with similar attributes to your target audience. For example, if your campaign targets members with the skill ‘Online Advertising,’ your campaign might also be shown to members who list the skill ‘Interactive Marketing’ on their profile if Audience Expansion is enabled. This means you can discover new quality prospects and automatically drive them into your marketing funnel.”).
1
For a technical discussion of the implications of this situation, see Cynthia Dwork and Christina Ilvento, Fairness Under Composition, CoRR, 2018, [https://arxiv.org/pdf/1806.06122.pdf].
1
As advertising delivery algorithms autonomously learn which type of users tend to take an advertiser’s target action, they often automatically adjust to show that ad to similar users who fall within the bounds of the initial target audience. Onuoha v. Facebook, Inc., Case No. 5:16-cv-06440-EJD, Amicus Brief in Support of Plaintiffs filed by Upturn, Inc., November 16, 2018, [https://www.upturn.org/static/files/2018-11-16_Upturn_Facebook_Amicus.pdf]; Standard events best practices, Facebook Advertiser Help, https://www.facebook.com/business/help/402791146561655?helpref=faq_content (accessed November 7, 2018); What are custom conversions and how do I use them?, Facebook Advertiser Help, https://www.facebook.com/business/help/780705975381000?helpref=faq_content, (accessed November 7, 2018).
1
Kim and Scott, supra note 80.
1
See id. at 26; Rieke and Bogen, supra note 109.
1
We have argued that platforms that play a meaningful role in determining which users receive which advertisements should not necessarily be immune from unlawful, discriminatory outcomes. Onuoha v. Facebook, Inc., Case No. 5:16-cv-06440-EJD, Amicus Brief in Support of Plaintiffs filed by Upturn, Inc., November 16, 2018, https://www.upturn.org/static/files/2018-11-16_Upturn_Facebook_Amicus.pdf (in which we assert that “Facebook, through the operation of its ad delivery system, independently directs housing ads based on its users’ protected class characteristics. Facebook’s users, in the normal course of using Facebook’s services, cannot help but reveal to Facebook preferences and personal characteristics that enable this discrimination to occur. As a result, Facebook develops content that contributes materially to unlawfulness under the Fair Housing Act.”).
1
Thomas v. Washington County School Board, 915 F.2d 922, 925 (4th Cir. 1990) (limiting the posting of job openings in favor of word-of-mouth hiring when there is a predominantly white workforce violates Title VII because “[these policies] serve to freeze the effects of past discrimination.”).
1
Kim and Scott, supra note 80.
1
Supra note 73.
1
Executive Order 11246 §202(1), available at https://www.dol.gov/ofccp/regs/statutes/eo11246.htm (requiring government contracting agencies to “take affirmative action to ensure that applicants are employed, and that employees are treated during employment, without regard to their race, color, religion, sex, sexual orientation, gender identity, or national origin. Such action shall include, but not be limited to the following: employment, upgrading, demotion, or transfer; recruitment or recruitment advertising […]”).
1
See Kim and Scott, supra note 80; Amit Datta, Anupam Datta, Jael Makagon, Deirdre K. Mulligan, and Michael Carl Tschantz, Discrimination in Online Advertising: A Multidisciplinary Inquiry, Proceedings of the 1st Conference on Fairness, Accountability and Transparency, 2-18, http://proceedings.mlr.press/v81/datta18a/datta18a.pdf.
1
ZipRecruiter, https://www.ziprecruiter.com.
1
Sirui Yao and Bert Huang, Beyond Parity: Fairness Objectives for Collaborative Filtering, 31st Conference on Neural Information Processing Systems (NIPS 2017), https://papers.nips.cc/paper/6885-beyond-parity-fairness-objectives-for-collaborative-filtering.pdf (“[a] frequently practiced approach for recommendation called collaborative filtering…makes recommendations based on the ratings or behavior of other users in the system. The fundamental assumption behind collaborative filtering is that other users’ opinions can be selected and aggregated in such a way as to provide a reasonable prediction of the active user’s preference.”).
1
Id.
1
Elizabeth MacBride, How AI Aids Small Business Hiring: An Interview With ZipRecruiter’s CEO, Forbes, October 31, 2017, https://www.forbes.com/sites/elizabethmacbride/2017/10/31/meet-the-jobs-startup-with-leverage-to-bring-google-and-facebook-to-the-table/#1e60659033fb (“ZipRecruiter has found that when job seekers just apply to any and all jobs they find at random, employers (on average) will give one six of those candidates a ‘thumbs up’ on the platform. […] When ZipRecruiter’s machine-learning algorithm drives certain candidates to apply to certain jobs, employers give one in four of those candidates a ‘thumbs up.’ Once employers give someone a ‘thumbs up,’ ZipRecruiter looks for jobseekers similar to that candidate – with 1 in 3 employers giving those candidates a “thumbs up.’”).
1
This is a challenging problem, since users’ behavior may indeed accurately reflect their beliefs and preferences, but still reflect internal and subconscious biases that continue to drive systemic racial, gender, and other disparities.
1
Researchers have shown a similar effect in online advertising. Amit Datta, Michael Carl Tschantz, and Anupam Datta, Automated Experiments on Ad Privacy Settings, Proceedings on Privacy Enhancing Technologies 2015; 2015 (1):92–112, http://www.andrew.cmu.edu/user/danupam/dtd-pets15.pdf (finding that “simulated males were more often shown ads encouraging the user to seek coaching for high paying jobs than simulated females” on Google).
1
Alexandra Chouldechova and Aaron Roth, The Frontiers of Fairness in Machine Learning, arXiv, October 20, 2018, https://arxiv.org/pdf/1810.08810.pdf (“The vast majority of work in computer science on algorithmic fairness has focused on one-shot classification tasks. But real algorithmic systems consist of many different components that are combined together, and operate in complex environments that are dynamically changing, sometimes because of the actions of the learning algorithm itself.” The authors also note that while several papers have considered the issue, “the high level message from these works is that our current notions of fairness compose poorly.”). Researchers of recommender systems have also noted that notions and metrics of fairness commonly used to assess simpler predictive tools like those used in the criminal justice context are insufficient to describe and remedy unfair effects within these more complex recommendation algorithms. Yao and Huang, supra note 125.
1
See Moritz Hardt, How big data is unfair, Medium, September 26, 2014, https://medium.com/@mrtz/how-big-data-is-unfair-9aa544d739de (“If the training data reflect existing social biases against a minority, the algorithm is likely to incorporate these biases. This can lead to less advantageous decisions for members of these minority groups. Some might object that the classifier couldn’t possibly be biased if nothing in the feature space speaks of the protected attributed, e.g., race. This argument is invalid. After all, the whole appeal of machine learning is that we can infer absent attributes from those that are present. Race and gender, for example, are typically redundantly encoded in any sufficiently rich feature space whether they are explicitly present or not. They are latent in the observed attributes and nothing prevents the learning algorithm from discovering these encodings. In fact, when the protected attribute is correlated with a particular classification outcome, this is precisely what we should expect.”); Yao and Huang, id. (“When aiming to protect algorithms from treating people differently for prejudicial reasons, removing sensitive features (e.g., gender, race, or age) can help alleviate unfairness but is often insufficient. Features are often correlated, so other unprotected attributes can be related to the sensitive features and therefore still cause the model to be biased. Moreover, in problems such as collaborative filtering, algorithms do not directly consider measured features and instead infer latent user attributes from their behavior.” (internal citations omitted)).
1
Arvind Narayanan, @random_walker, “If you personalize based on viewing history, targeting by race/gender/ethnicity is a natural emergent effect. But a narrowly worded denial allows companies to deflect concerns. Journalists: when dealing with machine learning systems, you need to up your game.” October 23, 2018, 10:25 AM, https://twitter.com/random_walker/status/1054786014072528897; Nitasha Tiku, Why Netflix Features Black Actors In Promos to Black Users, Wired, October 24, 2018, https://www.wired.com/story/why-netflix-features-black-actors-promos-to-black-users.
1
Charge of Discrimination , Communications Workers of America against Facebook, Equal Employment Opportunity Commission (September 18, 2018), *available at* (https://www.aclu.org/sites/default/files/field_document/facebook_eeoc_complaint-_cwa.pdf) (alleging that “Facebook targeted all of these discriminatory advertisements, as both an employment agency and an agent of the other companies, and received money for doing so.”); See also Onuoha v. Facebook, Inc., Case No. 5:16-cv-06440-EJD, Plaintiffs’ First Amended Complaint at 27 (arguing that Facebook is an employment agency because the company “regularly receives compensation from employers to place advertisements for employers—and provide related marketing, recruitment, sourcing, advertising, branding, information, and/or hiring services to and on behalf of employers—in order to recruit applicants for employment and encourage them to apply for employment with such employers.”).
1
E.g. Onuoha v. Facebook, Inc., Defendant’s Motion to Dismiss, Case No. 5:16-cv-06440-EJD at 29 (responding that “[p]roviding a platform for third parties to publish their ads does not transform Facebook into an employment agency.”).
1
For example, the OFCCP requires federal contractors to keep detailed records on “Internet applicants.” According to the rule, “[a]n ‘Internet applicant” is an individual who satisfies all four of the following criteria: The individual submitted an expression of interest in employment through the Internet or related electronic data technologies; The contractor considered the individual for employment in a particular position; The individual’s expression of interest indicated that the individual possesses the basic qualifications for the position; and The individual, at no point in the contractor’s selection process prior to receiving an offer of employment from the contractor, removed himself or herself from further consideration or otherwise indicated that he/she was no longer interested in the position.” 41 C.F.R. § 60-1.12. It is not immediately clear how matching platforms, which allow employers and jobseekers to assess one another without formal expressions of intent and via largely automated consideration square with this rule. At the same time, the regulator has clarified, for example, that “[a] job seeker is ‘considered’ for employment in a particular position if the contractor assesses the substantive information provided in the resume with respect to any qualification involved with the particular position. The software reviews job seekers’ qualifications and ranks job seekers based not merely on whether they possess the basic qualifications but on an assessment of the extent to which they possess those qualifications vis–à–vis other candidates. Consequently, the resumes of job seekers reviewed by the software have been considered for a particular position under the Internet Applicant rule.” Internet Applicant Recordkeeping Rule, Office of Federal Contract Compliance Programs, https://www.dol.gov/ofccp/regs/compliance/faqs/iappfaqs.htm#Q4JS (accessed November 8, 2018).
1
However, in a tight job market this sort of activity may become more popular as employers struggle to fill open positions.
1
See, e.g., Entelo Smart Profiles With Candidate Insights, Entelo Help, https://entelo.zendesk.com/hc/en-us/articles/360003166832-Entelo-Smart-Profiles-with-Candidate-Insights (accessed October 7, 2018).
1
The platform flags those people predicted to have at least a 30 percent chance of changing jobs in the next 90 days as being “more likely to move.” What Makes A Candidate “More Likely To Move”?, Entelo Help, https://entelo.zendesk.com/hc/en-us/articles/204690129-What-makes-a-candidate-More-Likely-to-Move- (accessed October 7, 2018).
1
John Bischke, Entelo Study Shows When Employees are Likely to Leave Their Jobs, Entelo Blog, October 6, 2014, https://blog.entelo.com/new-entelo-study-shows-when-employees-are-likely-to-leave-their-jobs.
1
The tool also presents candidates’ predicted salary range based on job title and third-party information. Entelo relies on a company called Paysa, which itself uses machine learning techniques to calculate salary averages. Entelo Smart Profiles With Candidate Insights, supra note 137. Notably, Paysa also makes its data available to jobseekers. See more at Paysa, https://www.paysa.com (accessed October 7, 2018). Salary predictions are an important component of equity in hiring systems which will be addressed in a later section.
1
Can an Algorithm Get More Women Hired?, The Wall Street Journal, May 29, 2014, https://www.wsj.com/video/can-an-algorithm-get-more-women-hired/935B1EB4-7734-439B-B6B1-7193FBD5212D.html. An Indian vendor called Belong, which serves companies recruiting primarily in southeast Asia, nearly mirrors Entelo’s capabilities: it crawls the web for passive candidates, predicts passive candidates’ likelihood of changing jobs, and allows employers to search for “diverse”—in this case, female—candidates. https://belong.co (accessed October 7, 2018).
1
Can an Algorithm Get More Women Hired?, id.
1
Photos (sourced from elsewhere on the web) are displayed on candidate profiles, though Unbiased Sourcing Mode will “anonymize names and hide photos, school names, employment gaps, years of experience, graduation dates and replace gender-specific pronouns.” Entelo Encourages Diversity and Inclusion in Hiring Practices, Adding New Unbiased Sourcing Mode to Its Platform, Entelo, August 8, 2018, https://www.entelo.com/press-releases/entelo-encourages-diversity-and-inclusion-in-hiring-practices-adding-new-unbiased-sourcing-mode-to-its-platform/.
1
Entelo calls this predictive feature “peer-based skills.” Sigacheva, supra note 99. LinkedIn does something similar for their ad targeting options, using “look-alike modeling to infer skills from a member’s job title and job description,” but it is not clear if these inferred skills are also used to surface candidates in manual search results. Unleashing LinkedIn’s Targeting Capabilities, 2017, https://business.linkedin.com/content/dam/me/business/en-us/marketing-solutions/cx/2017/pdfs/linkedin-targeting-guide.pdf at 14.
1
Sigacheva, id.
1
As part of this process, the platform considers signals including users’ profile data and past behavior against job attributes including “explicit/implicit skills,” job title, industry, and company size, and recency of the job posting to predict the probability the user will click on a given job. Jobs that score below a certain threshold of relevance to individual users are not shown. LinkedIn also uses matching functions described in the preceding section. Sankar Venkatraman, Candidate Matching Algorithms Explained: How LinkedIn Matches Job Seekers With Employers and Vice Versa, A Comprehensive Outlook on Matching Technology, TalentTech Labs, February 2018, https://talenttechlabs.com/wp-content/uploads/2018/02/Trends-Report-A-Comprehensive-Outlook-on-Matching-Technology.pdf (“Before relevant jobs are presented to members, they pass through multiple matching, filtering and ranking stages, each of which is driven by our Machine Learning algorithms. During each stage, the relevant jobs for a member are narrowed down starting from an index of several million jobs on the platform down to a couple hundred of relevant jobs that are eventually ranked and recommended to the seeker.”)
1
This is likely inferred in part based on how actively the candidate is browsing LinkedIn for job openings.
1
Venkatraman, supra note 146 (“Other aspects that also go into the matching algorithms include query features such as the frequency of appearance of the search parameters (for instance a keyword) in a candidate’s profile or recruiter-candidate features like the relationship between recruiter and the target candidate (for e.g. does the recruiter tend to prefer candidate from a particular industry or a company or a region etc.). LinkedIn’s solution takes into account over 100 such signals to build relevance models and rank candidates.”).
1
LinkedIn calls this feature “Representative Results.” Rosalie Chan, LinkedIn is using AI to make recruiting diverse candidates a no-brainer, Business Insider, October 10, 2018, https://www.businessinsider.com/linkedin-new-ai-feature-increase-diversity-hiring-2018-10.
1
Chan, id.
1
Kim and Scott, supra note 80 at 26-27.
1
For a technical discussion of the proportion-based approach LinkedIn seems to have built on, see Van Dang and W. Bruce Croft, Diversity by Proportionality: An Election-based Approach to Search Result Diversification, SIGIR’12, August 12-16, 2012, https://ciir-publications.cs.umass.edu/getpdf.php?id=1050. Some researchers call the sort of intervention LinkedIn implemented “fairness-aware re-ranking.” See, e.g., Weiwen Liu and Robin Burke, Personalizing Fairness-aware Re-ranking, FATREC’18, October 2018, https://arxiv.org/pdf/1809.02921.pdf. For a discussion on fairness metrics in rankings, see Ke Yang and Julia Stoyanovich, Measuring Fairness in Ranked Outputs, SSDBM ‘17 Proceedings of the 29th International Conference on Scientific and Statistical Database Management, June 2017, https://dl.acm.org/citation.cfm?id=3085526.
1
We do not refer here to basic eligibility screening tools that do not pertain to specific roles, like employment verification, drug tests, or basic criminal background checks.
1
These systems look for information about candidate’s education (e.g. listed institutions, degrees, and majors), self-reported skills, and work experience (e.g. former employers and job titles). Melanie Pinola, How Can I Make Sure My Resume Gets Past Resume Robots and into a Human’s Hand?, LifeHacker, December 9, 2011, https://lifehacker.com/5866630/how-can-i-make-sure-my-resume-gets-past-resume-robots-and-into-a-humans-hand; Bo Cowgill, Bias and Productivity in Humans and Algorithms: Theory and Evidence from Resume Screening, presented at IZA Workshop: Labor Productivity and the Digital Economy, October 2017, http://conference.iza.org/conference_files/MacroEcon_2017/cowgill_b8981.pdf (describing how common keywords systems behave: “The keywords on the resumes were first merged together based on common linguistic stems (for example ‘swimmer’ and ‘swimming’ were counted toward the ‘swim’ stem). Then, resume covariates were created to represent how many times each stem was used on each resume.”). Some estimate that these systems screen out up to 75 percent of applicants, in part due to rigid keyword matching rules and poor font recognition. Terena Bell, The secrets to beating an applicant tracking system (ATS), CIO, April 17, 2018, https://www.cio.com/article/2398753/careers-staffing/careers-staffing-5-insider-secrets-for-beating-applicant-tracking-systems.html.
1
For example, while older resume systems would be restricted to looking for words like “retail” on resumes for retail positions, newer technologies can recognize that past work at retailers like Walmart can also signal qualification. Artificial Intelligence for High-Volume Retail Recruiting, Ideal, January 2017, https://ideal.com/wp-content/uploads/2017/01/Ideal-AI-For-Retail-Recruiting-eBook-2.3.pdf.
1
Leslie Hook, Prepare to Meet the Robot Recruiters, Financial Times, October 15, 2017, https://www.ft.com/content/ac97e806-a520-11e7-8d56-98a09be71849. For example, one employer used Mya to screen 140,000 applicants for 20,000 seasonal warehouse jobs. Allan Schweyer, Robots in Recruiting: The Implications of AI on Talent Acquisition, Appcast, May 26, 2017, https://www.slideshare.net/appcast_io/whitepaper-robots-in-recruiting-the-implications-of-ai-on-talent-acquisition. In 2017, the company entered into a 3-year partnership with Adecco, the largest temporary staffing company in the world. AI-Recruiting Company, Mya Systems, Inks 3-Year Global Partnership With World’s Leading Workforce Solutions Provider, The Adecco Group, to Automate Its Recruiting Operations, Business Wire, August 10, 2017, https://www.businesswire.com/news/home/20170810005371/en/AI-Recruiting-Company-Mya-Systems-Inks-3-Year-Global. Popular recruitment marketing company Smashfly offers an analogous tool called Emerson. Elyse Mayer, Recruiting With Smashfly’s Emerson: A Dynamic Experience for Talent and TA Teams, Smashfly Blog, August 13, 2018, http://blog.smashfly.com/2018/08/13/smashfly-launches-ai-recruiting-assistant-emerson; a Russian analog, Robot Vera, performs similar tasks through a speech-based interface and can also conduct basic interviews. Robot Vera, https://ai.robotvera.com (accessed October 7, 2018).
1
Traditional applicant tracking systems often allow employers to manually define and weight the importance of screening questions, and to transform candidates’ answers into behind-the-scenes scores based on those answers.
1
Peng Lai “Perry” Li, Natural Language Processing, 1 Georgetown Law Technology Review 98, 2016, https://www.georgetownlawtechreview.org/natural-language-processing/GLTR-11-2016.
1
Schweyer, supra note 156.
1
Artificial Intelligence for High-Volume Retail Recruiting, supra note 155.
1
Ideal, https://ideal.com.
1
Artificial Intelligence for High-Volume Retail Recruiting, supra note 155.
1
Ideal does appear to offer—but does not guarantee it will perform—testing and monitoring for adverse impact in its candidate grading system. Workplace Diversity Through Recruitment: A Step-By-Step Guide, Ideal, https://ideal.com/product/reduce-bias (accessed October 7, 2018). For customers who collect demographic data during the course of their hiring process, Ideal explains that it can instruct its algorithms to both ignore those demographics and test for and removed adverse impact based on the EEOC’s 4/5th rule, the U.S. Department of Labor’s affirmative action program, Canada’s equity programs for designated groups, and the European Union’s hiring discrimination laws. Compliance In Recruiting: How Ideal’s Technology Prioritizes Compliance, https://ideal.com/compliance (accessed October 7, 2018). Mya has not appeared to made public statements about whether it attempts to monitor its system for disparate impact.
1
See, e.g., Julia Hirschberg and Christopher D. Manning, Advances in natural language processing, Science, Vol. 34 Iss. 6245, July 17, 2015, https://cs224d.stanford.edu/papers/advances.pdf.
1
Adam Sutton, Thomas Lansdall-Welfare, and Nello Cristianini, Biased Embeddings from Wild Data: Measuring, Understanding and Removing, arXiv, June 16, 2018, https://arxiv.org/pdf/1806.06301.pdf.
1
Natural language processing algorithms have been shown to perform poorly on phrases written with African American English syntax. Su Lin Blodgett, Lisa Green, and Brendan O’Connor, Demographic Dialectal Variation in Social Media: A Case Study of African-American English, Proceedings of the 2016 Conference on Empirical Methods in Natural Language Processing, November 2016, https://aclweb.org/anthology/D16-1120.
1
This is particularly concerning when employers rely on chatbots to screen candidates for jobs where writing is not a central job requirement. One solution might be redirecting to a human recruiter those candidates with whom the chatbot struggles—but this diminishes the benefit of blindness. Either way, such systems still require active monitoring to ensure the chatbots are not unduly screening out qualified candidates.
1
For instance, Google researchers found that two consequential, publicly available image data sets that are frequently used to train image recognition algorithms lacked geographic diversity, making machine learned models more likely to fail when presented with pictures from the developing world. To address this challenge, the company launched an “Inclusive Images” competition to encourage the development of more inclusive—and more accurate—models. Shreya Shankar, Yoni Halpern, Eric Breck, James Atwood, Jimbo Wilson, and D. Sculley, No Classification without Representation: Assessing Geodiversity Issues in Open Data Sets for the Developing World, NIPS 2017 workshop: Machine Learning for the Developing World, https://ai.google/research/pubs/pub46553; Tulsee Doshi, Introducing the Inclusive Images Competition, Google AI Blog, September 6, 2018, https://ai.googleblog.com/2018/09/introducing-inclusive-images-competition.html.
1
Simon Chandler, The AI Chatbot Will Hire You Now, Wired, September 13, 2017, https://www.wired.com/story/the-ai-chatbot-will-hire-you-now/ (“Grayevsky explains that Mya Systems “sets controls” over the kinds of data Mya uses to learn. That means that Mya’s behavior isn’t generated using raw, unprocessed recruitment and language data, but rather with data pre-approved by Mya Systems and is clients. This approach narrows Mya’s opportunity to learn prejudices in the manner of Tay—a chatbot that was released into the wilds by Microsoft last year and quickly became racist, thanks to trolls.”); cf. Peter Lee, Learning from Tay’s introduction, Official Microsoft Blog, March 25, 2016, https://blogs.microsoft.com/blog/2016/03/25/learning-tays-introduction/.
1
Nikoletta Bika, Pre-employment testing: a selection of popular tests, Workable, https://resources.workable.com/tutorial/pre-employment-tests (accessed November 8, 2018). An industry-sponsored survey found that 76 percent of employers use assessments as part of their hiring decision; 86 percent of companies with more than 1,000 employees did so. The State of Pre-Hire Assessments, HR.com, 2018. The field of industrial-organizational (I/O) psychology focuses in part on developing and validating techniques and testing instruments to assess job applicants. Thirty two percent of employers use behavioral assessments, with another 19 percent considering it; 4 percent use game- or scenario-based assessments, with another 16 percent considering it. Stacey Harris and Erin Spencer, Sierra-Cedar 2018–2019 HR Systems Survey, September 12, 2018, https://www.sierra-cedar.com/wp-content/uploads/sites/12/2018/09/Sierra-Cedar_2018-2019_HRSystemsSurvey_WhitePaper.pdf.
1
Eighty two percent of companies include some form of pre-employment assessment in their hiring process. Dave Zielinski, Predictive Assessments Give Companies Insight into Candidates’ Potential, Society for Human Resource Management, January 22, 2018, https://www.shrm.org/resourcesandtools/hr-topics/talent-acquisition/pages/predictive-assessments-insight-candidates-potential.aspx.
1
An industry-sponsored survey found that roughly one third of employers use psychometric assessments, while roughly 15 percent use assessments enhanced by more advanced artificial intelligence and machine learning technology. The State of Pre-Hire Assessments, HR.com, 2018.
1
IBM Kenexa, for instance, describes its off-the-shelf assessments as “us[ing] behavioral science techniques to measure traits, skills, and fit of candidates and/or employees,” built by “a team of I/O psychologists and 30+ years of behavioral science expertise […].” Hire with confidence: IBM Kenexa Employee Assessments Full product catalog (accessed November 2, 2018), https://public.dhe.ibm.com/common/ssi/ecm/lo/en/los14071usen/los14071-usen-02_LOS14071USEN.pdf.
1
Module Library, Indeed Assessments, https://www.indeed.com/assessments/module-library (accessed November 8, 2018); IBM Kenexa Employee Assessments, https://www.ibm.com/us-en/marketplace/employee-assessments/details (accessed November 8, 2018).
1
Stephanie Condon, Indeed rolls out platform to remove bias from hiring, ZDNet, https://www.zdnet.com/article/indeed-rolls-out-platform-to-remove-bias-from-hiring/.
1
Many thanks to Cornell professor Ifeoma Ajunwa for her astute articulation of the difference between off-the-shelf and bespoke assessments.
1
The vendor also divides each attribute into more specific subcategories: Grit (growth mindset, self efficiency), ownership (citizenship, integrity, conscientiousness), curiosity (creativity, empathy), polish (communications), teamwork (emotional intelligence, collaboration, positivity), rigor (evidence-based decision-making), and impact (real-world problem-solving, innovation). Koru also offers employers the option to add their own target competencies and develop measurements for them.
1
Since hiring assessments must be shown to be “valid” for any given employer in to pass legal muster, mature vendors in this space know to train their models using local data—that is, predictive models are trained using an employer’s own position-specific data.
1
Predictive Hiring: How Artificial Intelligence is Helping Recruiters w/ @Kristen_Hammy, Experian, May 17, 2018, http://www.experian.com/blogs/news/datatalk/predictive-hiring.
1
Josh Jarrett and Sarah Croft, The Science Behind Predictive Hiring for Fit, Koru, http://23nc3f3w6gde3a85ex2h0swb.wpengine.netdna-cdn.com/wp-content/uploads/2017/10/Koru7_ImpactSkills_WhitePaper.pdf; Ankush Gupta, Q & A with Kristen Hamilton, CEO, Koru, HR Technologist, May 24, 2017, https://www.hrtechnologist.com/interviews/recruitment-onboarding/q-a-with-kristen-hamilton-ceo-koru-3/.
1
Pymetrics, supra note 79.
1
These games and their related measurements are adapted from academic neuroscience research. Pymetrics Internal Demo Day Pitch, Microsoft for Startups, January 30, 2017, https://www.youtube.com/watch?v=hzSlmZZQZgQ.
1
These traits include memory span, processing speed, attention duration, willingness to take risks, ability to learn from feedback, altruism, planning speed, flexibility, reward responsiveness, and focus, among others. Pymetrics, supra note 79; Pymetrics: using science + technology to improve recruiting for all, SlideShare, August 21, 2014, https://www.slideshare.net/pymetrics/pymetrics-marketplace-38226636 at 12. Rather than determining whether or not candidates have a certain trait, Pymetrics places each trait on a spectrum: instead of being a “strong” or “weak” planner, an applicant might be deemed a more “deliberate planner” than an “efficient planner.” Matching People to Careers Bias-Free // Frida Polli, Pymetrics (FirstMark’s Data Driven), Data Driven NYC, February 3, 2017, https://www.youtube.com/watch?v=Yv6bqDZtoVs.
1
Pymetrics Internal Demo Day Pitch, supra note 182.
1
Id.
1
Job-Matching Platform Pymetrics Closes $40 Million Funding Round, Staffing Industry Analysts, September 28, 2018, https://www2.staffingindustry.com/site/Editorial/Daily-News/Job-matching-platform-pymetrics-closes-40-million-funding-round-47578?.
1
Pymetrics, supra note 79.
1
An industry analysis found Pymetrics to have “the most structured understanding of the regulatory climate. They design their process to meet the EEOC’s ⅘ rule of thumb. That is, a process is generally not discriminatory of (sic) the members of a minority class pass a given workflow hurdle at a rate of at least 80% of the majority class.” The Emergence of Intelligent Software: The 2018 Index of Predictive Tools in HRTech, HRExaminer, https://www.goscoutgo.com/wp-content/uploads/2017/11/HRX170917-Emergence-of-Intelligent-Software-v4.2s-1.pdf.
1
In other words, the company says it aims to not “overweight” traits that are more predictive of membership in a dominant demographic group than of a defined measure of job success. Khari Johnson, Pymetrics open-sources Audit AI, an algorithm bias detection tool, VentureBeat, May 31, 2018, https://venturebeat.com/2018/05/31/pymetrics-open-sources-audit-ai-an-algorithm-bias-detection-tool.
1
These include the EEOC’s 4/5 test, Fisher exact test, Z-test, Bayes factor test, and the chi squared test, all of which are used to test the likelihood the observed correlation happened by chance. audit-AI: Open Sourced Bias Testing for Generalized Machine Learning Applications, https://github.com/pymetrics/audit-ai.
1
Pymetrics, supra note 79.
1
audit-AI: Open Sourced Bias Testing for Generalized Machine Learning Applications, https://github.com/pymetrics/audit-ai.
1
Eric Rosenbaum, Silicon Valley is stumped: Even A.I. cannot always remove bias from hiring, CNBC, May 30, 2018, https://www.cnbc.com/2018/05/30/silicon-valley-is-stumped-even-a-i-cannot-remove-bias-from-hiring.html (noting that “pymetrics […] does not let third-party algorithm auditing firms, like [Cathy] O’Neil’s, review its actual job-hiring code for undetected bias.”).
1
See, e.g., Craig Haney, supra note 15 at 2, 9. (asserting that “[…] these tests represent a most formidable barrier to equal opportunity and racial justice in the workplace,” because “[t]esting was used as the instrument of a racist world view that held whole groups of people to be genetically inferior to others, while the early test enthusiasts proclaimed the neutrality of the instruments that supposedly documented racial inferiority.”).
1
See, e.g., Enforcement Guidance: Disability-Related Inquiries and Medical Examinations of Employers Under the Americans with Disabilities Act (ADA), U.S. Equal Employment Opportunity Commission, July 27, 2000, available at https://www.eeoc.gov/policy/docs/guidance-inquiries (articulating that “[h]istorically, many employers asked applicants and employees to provide information concerning their physical and/or mental condition. This information often was used to exclude and otherwise discriminate against individuals with disabilities – particularly nonvisible disabilities, such as diabetes, epilepsy, heart disease, cancer, and mental illness – despite their ability to perform the job.”).
1
This topic is discussed in detail in the “Performance Evaluation” section of this report. See also, e.g., Melissa Hart, Subjective Decisionmaking and Unconscious Discrimination, 56 Alabama Law Review 746, 2005, https://papers.ssrn.com/sol3/papers.cfm?abstract_id=788066; Rachel Geman, “Don’t I Think I Know You Already?”: Excessive Subjective Decision Making as an Improper Tool for Hiring and Promotion, Second Annual ABA Section of Labor and Employment Law Conference, 2008, https://www.americanbar.org/content/dam/aba/administrative/labor_law/meetings/2008/ac2008/030.pdf.
1
For instance, when Amazon tried to develop its own predictive tool, the tool ended up reflecting preference toward male candidates “because Amazon’s computer models were trained to vet applicants by observing patterns in resumes submitted to the company over a 10-year period. Most came from men, a reflection of male dominance across the tech industry.” Jeffrey Dastin, Amazon scraps secret AI recruiting tool that showed bias against women, Reuters, October 9, 2018, https://www.reuters.com/article/us-amazon-com-jobs-automation-insight/amazon-scraps-secret-ai-recruiting-tool-that-showed-bias-against-women-idUSKCN1MK08G.
1
Uniform Guidelines on Employee Selection Procedures §5 (General standards for validity studies). Indeed describes the steps it takes to establish validity for its off-the-shelf assessments, which include “us[ing] a standardized process in the determination and development of assessment content. This process attempts to link assessment content with job-relevant knowledge, skills, abilities, and other characteristics (KSAOs).” Indeed Assessments - EEOC Statement, https://www.indeed.com/assessments/eeoc (accessed November 10, 2018).
1
For example, a company white paper encourages employers to “[c]ollect data that could be predictive. Start with your hypotheses and cast a net from there. Don’t fall victim to the trap of ‘throw all the data in and the algorithms will find magical patterns.’ Rarely does that happen. Start with the data you already have that you believe carry signal, and/or signal-rich data that you can quickly capture.” Improving Candidate Quality: New Signals for Hiring in the Innovation Economy, Koru, https://www.joinkoru.com/wp-content/uploads/2018/05/New-Signals-for-Hiring_Koru.pdf.
1
This process is distinctly different than that used for off-the-shelf assessments, which tend to be geared toward broader categories of positions and pre-defined skills. Off-the-shelf tools may rely more heavily on different theories of validity than bespoke, machine learning driven tools do, namely content and construct validity. See Uniform Guidelines on Employee Selection Procedures §5(B) (“Evidence of the validity of a test or other selection procedure by a content validity study should consist of data showing that the content of the selection procedure is representative of important aspects of performance on the job for which the candidates are to be evaluated. […] Evidence of the validity of a test or other selection procedure through a construct validity study should consist of data showing that the procedure measures the degree to which candidates have identifiable characteristics which have been determined to be important in successful performance in the job for which the candidates are to be evaluated.”).
1
Kim, supra note 60 (assessing that “[u]nder disparate impact doctrine, if a plaintiff shows that an employer practice has a disproportionate impact on a protected group, the employer may defend by showing that the practice is “job related … and consistent with business necessity.’ If an employer could meet this burden simply by showing that an algorithm rests on a statistical correlation with some aspect of job performance, then the test is entirely tautological, because, by definition, data mining is about uncovering statistical correlations. Any reasonably constructed model will satisfy the test, and the law would provide no effective check on data-driven forms of bias.” (internal citations omitted)); see also Barocas and Selbst, supra note 53.
1
Nicolas Geeraert, How knowledge about different cultures is shaking the foundations of psychology, The Conversation, March 9, 2018, https://theconversation.com/how-knowledge-about-different-cultures-is-shaking-the-foundations-of-psychology-92696 (“Individuals in the western world are indeed more likely to view themselves as free, autonomous and unique individuals, possessing a set of fixed characteristics. But in many other parts of the world, people describe themselves primarily as a part of different social relationships and strongly connected with others. This is more prevalent in Asia, Africa and Latin America. These differences are pervasive, and have been linked to differences in social relationships, motivation and upbringing.”); Hazel R. Markus and Shinobu Kitayama, Culture and the Self: Implications for Cognition, Emotion, and Motivation, Psychological Review 98(2), April 1991, https://www.researchgate.net/publication/232558390_Culture_and_the_Self_Implications_for_Cognition_Emotion_and_Motivation.
1
See David O. Sears, College sophomores in the laboratory: Influences of a narrow data base on social psychology’s view of human nature, Journal of Personality and Social Psychology, 51(3), 1986, and Joe Henrich, Steven J. Heine, and Ara Norenzayan, The weirdest people in the world? Behavioral and Brain Sciences, 33 (2-3), 2010.
1
The LinkedIn profile of Koru’s Senior Director of Assessment and Instructional Design indicates that the company “continuously test[s] and iterate[s] our assessment with the help of our Amazon Turk (mTurk) workers.” (accessed October 17, 2018).
1
Matthew Salganik, Bit by Bit: Social Research in the Digital Age, Princeton University Press, December 5, 2017; John Bohannon, Psychologists grow increasingly dependent on online research subjects, Science, June 7, 2016, http://www.sciencemag.org/news/2016/06/psychologists-grow-increasingly-dependent-online-research-subjects; Joel Ross, Lilly Irani, M. Six Silberman, Andrew Zaldivar, and Bill Tomlinson, B. (2010). Who are the Crowdworkers?: Shifting Demographics in Amazon Mechanical Turk, CHI EA 2010 (2863-2872).
1
Shari Trewin, AI Fairness for People with Disabilities: Point of View, IBM Accessibility Research, November 26, 2018, https://arxiv.org/pdf/1811.10670.pdf (“For example, if five of our job applicants use assistive technologies such as a screen reader or magnifier, and the online test itself is not fully accessible, then long response times could lead to systematic exclusion of these five applicants using assistive technologies, even though their disability is not known.”). As early as 2007, the EEOC has investigated whether personality tests “shut out people suffering from mental illnesses such as depression or bipolar disorder.” Lauren Weber and Elizabeth Dwoskin, Are Workplace Personality Tests Fair?, The Wall Street Journal, September 29, 2014, https://www.wsj.com/articles/are-workplace-personality-tests-fair-1412044257. In confidential settlements with the EEOC, Best Buy and CVS recently dropped personality tests from their recruitment process when the practice “came under increasing scrutiny for their potential to weed out people with mental illness or certain racial groups.” Best Buy, CVS Drop Personality Tests in Recruiting to Address EEOC Concerns, Talent Daily, June 12, 2018, https://www.cebglobal.com/talentdaily/best-buy-cvs-drop-personality-tests-in-recruiting-to-address-eeoc-concerns/.
1
Preliminary research has found that being reviewed first has a measurably positive impact on recruiters’ scores of applicants, while those reviewed later saw diminishing scores. Kate Glazebrook and Janna Ter Meer, Hiring, honeybees and human decision-making, June 29, 2017, https://medium.com/finding-needles-in-haystacks/hiring-honeybees-and-human-decision-making-33f3a9d76763.
1
Id.
1
At some firms, multiple people need to agree on whether to hire a person, which may reduce the influence of predictive decision aids at this stage. At Google, for instance, any hiring manager can say no about a candidate for any reason, but “cannot single-handedly give the “final yes” to extend a job offer. All suitable candidates must be passed along to a hiring committee for review.” Ruth Umoh, Top Google recruiter: Google uses this ‘shocking’ strategy to hire the best employees, CNBC, January 10, 2018, https://www.cnbc.com/2018/01/10/google-uses-this-shocking-strategy-to-hire-the-best-employees.html.
1
According to a prominent HR technology expert, “[a]lmost every major platform provider now has tools for video interviewing, and quickly growing vendors such as HireVue and others are now offering sophisticated I/O psychology assessments to screen and assess candidates.” Josh Bersin, HR Technology Disruptions for 2018, Bersin, http://marketing.bersin.com/rs/976-LMP-699/images/HRTechDisruptions2018-Report-100517.pdf.
1
Iris Bohnet, How to Take the Bias Out of Interviews, Harvard Business Review, April 18, 2016, https://hbr.org/2016/04/how-to-take-the-bias-out-of-interviews. Not only that, unstructured interviews were found in a meta-analysis to be significantly less predictive of performance than structured interviews. Frank L. Schmidt, The Validity and Utility of Selection Methods in Personnel Psychology: Practical and Theoretical Implications of 85 Years of Research Findings, 124 Psychological Bulletin 2 (1998) at 265.
1
In the company’s early days, they sent webcams to interviewees. RecTechFest - Hire Vue “Reimagining Hiring”, November 2, 2017, https://www.youtube.com/watch?v=dflPqaJFEy4.
1
Industry analysts estimate that as of 2018, 250 of HireVue’s 650 customers use the company’s predictive tools. The Emergence of Intelligent Software: The 2018 Index of Predictive Tools in HRTech, supra note 188. While HireVue does not currently focus heavily on judging personality type, in May 2018 the company acquired MindX, a game-based psychometric assessment company that purports to measure “problem-solving, mental flexibility, learning agility, attention, creativity, and quantitative aptitude.” HireVue Acquires MindX to Create a Robust AI-Based Talent Assessment Suite, HR Technologist, supra note 94.
1
We tried the AI software companies like Goldman Sachs and Unilever use to analyze job applicants, Business Insider, September 3, 2017, https://www.youtube.com/watch?v=QfuGRCmXmCs.
1
The company explains that because it understands such factors are prone to cultural variation, it trains model using people from the same culture(s) as the anticipated applicant pool. Loren Larson, Train, Validate, Re-train: How We Build HireVue Assessments Models, June 21, 2018, https://www.hirevue.com/blog/train-validate-re-train-how-we-build-hirevue-assessments-models; see also HireVue Launches Localized Japanese Version of AI-driven HireVue Assessments Product with Channel Partner Talent, March 27, 2018, https://cdn2.hubspot.net/hubfs/464889/HireVue%20Launches%20Localized%20Japanese%20Version%20of%20%20AI-driven%20HireVue%20Assessments%20Product%20with%20Channel%20Partner%20TalentA%20.pdf?t=1531413217995 (“For many jobs we localize results as a top performer in a customer service job in Australia may display different characteristics than a top performer in Japan.”).
1
RecTechFest, supra note 212 (describing how the system breaks down videos in three components: word choice, using natural language processing and voice-to-text transcriptions; the audio file, using spectrum analysis of volume, intonation, and speed; and facial analysis, comparing video frames to detect microexpressions). The tool does not use facial recognition in the traditional sense, in that it does not attempt to detect the identity of the speaker. Loren Larson, HireVue Assessments and Preventing Algorithmic Bias, June 22, 2018, https://www.hirevue.com/blog/hirevue-assessments-and-preventing-algorithmic-bias. Nevertheless, concerns about differential performance on people with different skin tones, uncommon facial characteristics, and certain disabilities remain salient.
1
RecTechFest, supra note 212.
1
Larson, supra note 215.
1
Id.
1
Rachael Tatman, Gender and Dialect Bias in YouTube’s Automatic Captions, Proceedings of the First Workshop on Ethics in Natural Language Processing, April 4, 2017, http://www.aclweb.org/anthology/W17-1606 (finding “robust differences in accuracy across both gender and dialect, with lower accuracy for 1) women and 2) speakers from Scotland”); Delip Rao, When Men and Women talk to Siri, March 9, 2018, http://deliprao.com/archives/276; Sonia Paul, Voice is the next big platform, unless you have an accent, Wired, March 20, 2017, https://www.wired.com/2017/03/voice-is-the-next-big-platform-unless-you-have-an-accent/; Rachel Tatman, Google’s speech recognition has a gender bias, July 12, 2016, https://makingnoiseandhearingthings.com/2016/07/12/googles-speech-recognition-has-a-gender-bias/ (finding a 70 percent chance transcriptions will be more accurate for men); Drew Harwell, The Accent Gap, The Washington Post, July 19, 2018, https://www.washingtonpost.com/graphics/2018/business/alexa-does-not-understand-your-accent (“People with nonnative accents, however, faced the biggest setbacks. In one study that compared what Alexa thought it heard versus what the test group actually said, the system showed that speech from that group showed about 30 percent more inaccuracies.”).
1
Buolamwini and Gebru, supra note 50.
1
For example, Mozilla launched an initiative to make training data for speech recognition software more inclusive. Michael Henretty, More Common Voices, Mozilla Open Innovation, June 7, 2018, https://medium.com/mozilla-open-innovation/more-common-voices-24a80c879944. Facial recognition researcher Joy Buolamwini’s research has prompted several leading software vendors to improve the accuracy of their models on people with darker skin. James Vincent, IBM hopes to fight bias in facial recognition with new diverse dataset, The Verge, June 27, 2018, https://www.theverge.com/2018/6/27/17509400/facial-recognition-bias-ibm-data-training. See also, Hee Jung Ryu, Hartwig Adam, and Margaret Mitchell, InclusiveFaceNet: Improving Face Attribute Detection with Race and Gender Diversity, ICML’18 FATML Workshop, 2018, https://arxiv.org/pdf/1712.00193.pdf; Ayanna Howard, Cha Zhang, and Eric Horvitz, Addressing bias in machine learning algorithms: A pilot study on emotion recognition for intelligent systems, 2017 IEEE Workshop on Advanced Robotics and its Social Impacts (ARSO), March 8-10, 2017, https://ieeexplore.ieee.org/abstract/document/8025197.
1
By immutable we refer not only unchangeable characteristics, but more broadly to those characteristics that are “a core trait or condition that one cannot or should not be required to abandon” and “traits that are so central to a person’s identity that it would be abhorrent … to penalize a person for refusing to change them, regardless of how easy that change might be physically.” Watkins v. U.S. Army, 875 F.2d 699, 726 (9th Cir. 1988) (Norris, J., concurring). See Jessica A. Clark, Against Immutability, 125 Yale Law Journal 1 (October 2015), https://www.yalelawjournal.org/article/against-immutability n.3-4 (citing Obergefell v. Wymyslo and DeBoer v. Snyder); Sharona Hoffman, The Importance of Immutability in Employment Discrimination Law, Case Western Reserve Faculty Publications (2011), https://scholarlycommons.law.case.edu/cgi/viewcontent.cgi?article=1010&context=faculty_publications.
1
For example, relying on an immutable characteristic that is not related to legally protected groups, or a characteristic not legally judged to be immutable but that is intrinsically associated with a person’s core identity or group membership.
1
For a discussion of the role of dignity in privacy invasive contexts, see Matt Reichel, Race, Class, and Privacy: A Critical Historical Review, International Journal of Communication 11 (2017).
1
Joy Buolamwini, When the Robot Doesn’t See Dark Skin, The New York Times, June 21, 2018, https://www.nytimes.com/2018/06/21/opinion/facial-analysis-technology-bias.html. The authors also appreciate Princeton professor Arvind Narayanan for his clear articulation of these concerns.
1
Tonya Riley, Get ready, this year your next job interview may be with an A.I. robot, CNBC, March 13, 2018, https://www.cnbc.com/2018/03/13/ai-job-recruiting-tools-offered-by-hirevue-mya-other-start-ups.html.
1
Loren Larson, supra note 216 (arguing “[f]irst of all, a HireVue Assessments model/algorithm is not a robot, but a form of AI/machine learning that has a single, specific, early-stage evaluation to perform. Its only focus is determining which subset of candidates within a given pool are most likely to be successful when compared to people already performing the job. That information is then provided to human recruiters as decision support. Those top candidates then move on from the screening stage to the person-to-person interviewing stages. Skilled recruiting professionals continue to decide which candidate gets the job after the completion of multiple stages in the hiring process.”).
1
RecTechFest, supra note 212.
1
An industry analysis identified HireVue as having one of “the most disciplined understanding[s] of bias and its management” of the 30 human resources technology companies that were interviewed. The Emergence of Intelligent Software: The 2018 Index of Predictive Tools in HRTech, supra note 188. The company’s director of data science has expressed, “[t]oday’s data scientists have a duty to test that their algorithms are not biased, ensuring their efforts do not unfairly impact certain demographic groups […] Since it is very difficult to know how bias is going to present itself once the algorithm is trained, post-training algorithm auditing is critical for identifying the implicit data that causes the greatest potential for bias.” How Recruiters Are Using Artificial Intelligence w/ @HireVue #DataTalk, Experian, April 3, 2018, https://www.youtube.com/watch?v=zvuJkPY2a2M.
1
See Tatsunori B. Hashimoto, Megha Srivastava, Hongseok Namkoong, and Percy Liang, Fairness Without Demographics in Repeated Loss Minimization, Proceedings of the 35th International Conference on Machine Learning, Stockholm, Sweden, PMLR 80, 2018, https://arxiv.org/pdf/1806.08010.pdf (“the substantial body of existing research into fairness for classification problems involving protected labels such as the use of race […] require the use of demographic labels, and are designed for classification tasks. This makes it difficult to apply such approaches to mitigate representation disparity in tasks such as speech recognition or natural language generation where full demographic information is often unavailable.”). Even before the use of predictive models, practitioners have come to the conclusion that in order to detect and correct for bias, they must have either direct or inferred access to data about protected group membership that can both reveal any bias and allow for measurable adjustment. For instance, in 1988, the British Commission for Racial Equity found a hospital that had introduced a computer screening tool to be guilty of racial and sexual discrimination, and recommended that “a question on racial origin be included in the UCCA [University Central Council for Admission] form,” finding that “[t]he percentage of non-European students in a medical school provides little information unless the proportion among applicants for places is known. At present this information is unobtainable, and it is ironic that protection of the interests of minority groups should necessitate their identification on application forms,” to prevent future discrimination. A Blot on the Profession, British Medical Journal (March 1988), http://europepmc.org/backend/ptpmcrender.fcgi?accid=PMC2545288&blobtype=pdf.
1
See, e.g., Michael Feldman, Sorelle A. Friedler, John Moeller, Carlos Scheidegger, and Suresh Venkatasubramanian, Certifying and Removing Disparate Impact, Proceedings of the 21st ACM SIGKDD International Conference on Knowledge Discovery and Data Mining, 2015; Cynthia Dwork, Moritz Hardt, Toniann Pitassi, Omer Reingold, and Richard Zemel, Fairness through awareness, Proceedings of Innovations in Theoretical Computer Science (2012); see generally research presented in proceedings of the ACM FAT* conferences. Much of this research focuses on the most basic demographic disparities, but recent work has shown more attention to intersectional analysis is needed. See, e.g., Buolamwini and Gebru, supra note 50; Sasha Costanza-Chock, Design Justice, A.I., and Escape from the Matrix of Domination, Journal of Design and Science (JoDS), July 26, 2018, https://cmsw.mit.edu/design-justice-ai-escape-matrix-of-domination/; Catherine D’Ignazio, A Primer on Non-Binary Gender and Big Data, MIT Center for Civic Media, June 3, 2016, https://civic.mit.edu/2016/06/03/a-primer-on-non-binary-gender-and-big-data/.
1
Few technical interventions, even within the context of academic research, are capable of handling complex sensitive attributes, and can only account for compound ones if they are transformed into an entirely new value. For example, a dataset with race and sex would need to affirmatively create a new data point for “race-sex.” Sorelle A. Friedler et al, A comparative study of fairness-enhancing interventions in machine learning, arXiv, February 13, 2018, https://arxiv.org/pdf/1802.04422.pdf (a meta-analysis of fairness aware algorithms, pointing out that some “require that the sensitive attributes be binary (e.g., “White” and “not White” instead of handling multiple racial categorizations) […]. While there are still very few fairness-aware algorithms that can formally handle multiple sensitive attributes directly in the algorithm all algorithms discussed can handle them if […] they are combined into a single sensitive attribute (e.g., race-sex). However, we might expect combining the attributes in this way to degrade performance under some metrics, especially in the case of algorithms that can only handle binary sensitive attributes, or when there are too many combinations for the size of the dataset to provide a large group of people with each new combined sensitive value.” (internal citations omitted)).
1
See, e.g., James Foulds and Shimei Pan, An Intersectional Definition of Fairness, 2018, https://arxiv.org/pdf/1807.08362.pdf.
1
We concede that these traits may be significantly more difficult to collect, observe, or infer than race, gender, or age due to their sensitivity, and that the collection and use of such data may be constrained under certain laws. Nevertheless, these features are relevant to the issue of discrimination in hiring. See András Tilcsik, Pride and prejudice: employment discrimination against openly gay men in the United States, American Journal of Sociology 117(2), September 2011, https://www.ncbi.nlm.nih.gov/pubmed/22268247; Google diversity annual report 2018, https://diversity.google/annual-report/ (describing in a footnote that “[w]e recognize that our current gender reporting is not inclusive of our non-binary population. We will consult on the best way forward, taking into account research such as Transgender-inclusive measures of sex/gender for population surveys.”).
1
Candidates harmed by an algorithm’s adverse impact might not be aware they were judged by such a system; even if they did, they would likely have trouble overcoming the high burden of proof necessary to prevail in a legal complaint. See Kim, supra note 85.
1
For instance, when Amazon tried to fix its recruitment tool that unfairly penalized women, “unqualified candidates were often recommended for all manner of jobs […] almost at random.” Jeffrey Dastin, Amazon scraps secret AI recruiting tool that showed bias against women, Reuters, October 9, 2018, https://www.reuters.com/article/us-amazon-com-jobs-automation-insight/amazon-scraps-secret-ai-recruiting-tool-that-showed-bias-against-women-idUSKCN1MK08G. Researchers often note the difficulty in balancing fairness and accuracy in predictive models. See John M. Kleinberg, Sendhil Mullainathan, and Manish Raghavan, Inherent Trade-Offs in the Fair Determination of Risk Scores, Proceedings of Innovations in Theoretical Computer Science (ITCS), 2017, https://arxiv.org/abs/1609.05807; Alexandra Chouldechova, Fair prediction with disparate impact: A study of bias in recidivism prediction instruments, FATML 2016, https://arxiv.org/pdf/1610.07524.pdf.
1
Technology to automate clerical minutiae—like generating offer letters, extending benefits, and creating access credentials—is common.
1
See, e.g., E-Verify, Civil Rights, Big Data, and Our Algorithmic Future, September 2014, https://bigdata.fairness.io/e-verify; E-Verify Errors: A Women’s Issue, National Immigration Law Center, March 2013, https://www.nilc.org/issues/workersrights/everifyimpactonwomen; Cynthia Gordy, When Background Checks Violate Civil Rights, The Root, April 25, 2012, https://www.theroot.com/when-background-checks-violate-civil-rights-1790884189.
1
Hire Learning, Fama, https://www.fama.io/hire-learning (accessed October 7, 2018).
1
In 2016, Fama announced a strategic partnership with a major background check firm First Advantage, putting te technology in front of a wide range of employers. Fama And First Advantage Announce Strategic Partnership, First Advantage Press Room, September 21, 2016, https://fadv.com/press-room/fama-fadv-announce-strategic-partnership.aspx.
1
The vendor initially offered analysis of Facebook, Twitter, and Instagram posts. Potential caregivers were asked to affirmatively provide Predictim permission to access these social media accounts. Predictim, https://www.predictim.com.
1
The company explains in a white paper what sort of behaviors might count within each category: “Bullying or Harassment: when an individual intentionally criticizes, insults, or denounces another individual, causing them to feel deeply hurt or upset. Drug Abuse: when an individual consumes a controlled substance recreationally. Examples include Heroin, Meth, Cocaine, Hydrocodone, Vicodin, Percocet, Morphine, Valium, Xanax, Marijuana, etc. Alcohol and Cigarettes are not considered drugs for this score. Disrespect and Antagonism: when an individual demonstrates a lack of respect, esteem, or courteous behavior. Explicit Content: when an individual posts sexual content.”
1
Predictim, https://www.predictim.com.
1
Dave Lee, Predictim babysitter app: Facebook and Twitter take action, BBC News, November 27, 2018, https://www.bbc.com/news/technology-46354276.
1
Facebook Platform Policy, https://developers.facebook.com/policy/ (accessed December 5, 2018) (“Don’t use data obtained from Facebook to make decisions about eligibility, including whether to approve or reject an application or how much interest to charge on a loan.”); Twitter Development Agreement and Policy VII(A)(3-4), https://developer.twitter.com/en/developer-terms/agreement-and-policy.html (accessed December 5, 2018) (“Twitter Content, and information derived from Twitter Content, may not be used by, or knowingly displayed, distributed, or otherwise made available to […] 3. any entity for the purposes of conducting or providing surveillance, analyses or research that isolates a group of individuals or any single individual for any unlawful or discriminatory purpose or in a manner that would be inconsistent with our users’ reasonable expectations of privacy; 4. any entity to target, segment, or profile individuals based on any entity to target, segment, or profile individuals based on health (including pregnancy), negative financial status or condition, political affiliation or beliefs, racial or ethnic origin, religious or philosophical affiliation or beliefs, sex life or sexual orientation, trade union membership, data relating to any alleged or actual commission of a crime, or any other sensitive categories of personal information prohibited by law […].”); Lee, id.
1
Drew Harwell, Facebook, Twitter crack down on AI babysitter-rating service, The Washington Post, November 27, 2018, https://www.washingtonpost.com/technology/2018/11/27/facebook-twitter-crack-down-ai-babysitter-rating-service.
1
For instance, Target recently agreed to review its screening criteria in response to criticism that “criminal records [] can include offenses too minor or old to affect their performance as employees.” Colin Moynihan, Target Agrees to Review Screening of Job Applicants Amid Claims of Bias, The New York Times, April 5, 2018, https://www.nytimes.com/2018/04/05/business/target-retail-hiring-bias.html.
1
Definitions of what constitutes toxic or concerning content are often vague and highly subjective. Natasha Duarte, Emma Llanso, and Anna Loup, Mixed Messages? The Limits of Automated Social Media Content Analysis, Center for Democracy & Technology, November 2017, https://cdt.org/files/2017/11/Mixed-Messages-Paper.pdf.
1
Implicit biases of initial content reviewers might color what type of content such tools end up judging to be problematic, in a way that could disproportionately flag as risky people from marginalized backgrounds. Google’s subsidiary Jigsaw suffered from this problem as it attempted to develop a tool to spot “toxic” online comments, inadvertently classifying phrases like “I am a man” as being less toxic, while phrases like “I am a gay black woman” was flagged as being highly toxic. Jessamyn West, August 24, 2017, https://twitter.com/jessamyn/status/900867154412699649. See also Caroline Sinders, Toxicity and Tone Are Not The Same Thing: analyzing the new Google API on toxicity, PerspectiveAPI, Medium, February 23, 2017, https://medium.com/@carolinesinders/toxicity-and-tone-are-not-the-same-thing-analyzing-the-new-google-api-on-toxicity-perspectiveapi-14abe4e728b3; Dave Gershgorn, Alphabet’s hate-fighting AI doesn’t understand hate yet, Quartz, February 27, 2017, https://qz.com/918640/alphabets-hate-fighting-ai-doesnt-understand-hate-yet/.
1
State Social Media Privacy Laws, National Conference of State Legislatures, November 6, 2018, http://www.ncsl.org/research/telecommunications-and-information-technology/state-laws-prohibiting-access-to-social-media-usernames-and-passwords.aspx.
1
Harwell, supra note 247.
1
Nagaraj Nadendla, Introducing Oracle Recruiting Cloud (Keynote), Oracle OpenWorld 2017, available at https://cloud.oracle.com/talent-management-cloud/videos.
1
For instance, Oracle includes in its list of predictive attributes used elsewhere in its tool data like workers’ home city. Predictive Attributes: Explained, Oracle Application Help, August 18, 2018, https://fga.fa.us1.oraclecloud.com/fscmUI/faces/AtkHelpPortalMain?TopicId=91F115C9860A9829E040D30A688147C6&_adf.ctrl-state=1c35rk138h_1&_afrLoop=554571474431440&_afrWindowMode=0&_afrWindowId=null&_afrFS=16&_afrMT=screen&_afrMFW=960&_afrMFH=819&_afrMFDW=1920&_afrMFDH=1080&_afrMFC=8&_afrMFCI=0&_afrMFM=0&_afrMFR=96&_afrMFG=0&_afrMFS=0&_afrMFO=0.
1
See, e.g., The Opportunity Atlas, https://www.opportunityatlas.org/ (accessed October 8, 2018); Sonya R. Porter and Maggie R. Jones, Where You Grow Up Can Affect Your Future, United States Census bureau, https://www.census.gov/library/stories/2018/10/opportunity-atlas.html.
1
Susan Mulligan, Salary History Bans Could Reshape Pay Negotiations, Society for Human Resource Management, February 16, 2018, https://www.shrm.org/hr-today/news/hr-magazine/0318/pages/salary-history-bans-could-reshape-pay-negotiations.aspx.
1
Some of these vendors include Pipeline, Pluto, SameWorks, Syndio Solutions and Visier. Stacia Sherman Garr and Carole Jackson, Diversity and Inclusion Technology: Could this be the Missing Link?, RedThread Research and Mercer, September 11, 2018.
1
See Christina Goldt, Supporting Workday Customers on Their Diversity Journeys, Workday Blog, October 11, 2017, https://blogs.workday.com/supporting-workday-customers-on-their-diversity-journeys/.
1
Scott Keller and Mary Meaney, Attracting and retaining the right talent, McKinsey, November 2017, https://www.mckinsey.com/business-functions/organization/our-insights/attracting-and-retaining-the-right-talent.
1
For example, supervisors tend to judge workers based on observable outcomes regardless of how much control workers had over the outcomes, in a phenomenon called outcome bias. See, e.g., Jonathan Baron and John C. Hershey, Outcome Bias in Decision Evaluation, Journal of Personality and Social Psychology, 54 (1988). This can happen at uneven rates across demographics: Women have been shown as being more likely to receive “critical subjective feedback” and their successes are more likely to be attributed to luck than skill or dedication than their male counterparts. Paola Cecchi-Dimeglio, How Gender Bias Corrupts Performance Reviews, and What to Do About It, Harvard Business Review, April 12, 2017, https://hbr.org/2017/04/how-gender-bias-corrupts-performance-reviews-and-what-to-do-about-it. Also, companies may not communicate clearly to workers what metrics will be considered as signals of success, and a company’s overall metrics may disproportionately benefit workers in certain roles. Employers, especially smaller ones, may also attempt to rely on data about current and past employees even when sample sizes are too small to reveal meaningful statistical insights. This phenomenon is often described as the “law of small numbers.” See, e.g., Matthew Rabin The Quarterly Journal of Economics Vol. 117, No. 3 (August 2002).
1
Kurt Kraiger and J. Kevin Ford, A Meta-Analysis of Ratee Race Effects in Performance Ratings, Journal of Applied Psychology 70(1), 1985, https://www.researchgate.net/profile/Kurt_Kraiger/publication/232527647_A_Meta-Analysis_of_Ratee_Race_Effects_in_Performance_Ratings/links/565311e208aeafc2aabadac0/A-Meta-Analysis-of-Ratee-Race-Effects-in-Performance-Ratings.pdf; JM Stauffer and MR Buckley, The existence and nature of racial bias in supervisory ratings, Journal of Applied Psychology 90(3), May 2005, https://www.ncbi.nlm.nih.gov/pubmed/15910152.
1
Alex Rosenblat, Karen E.C. Levy, Solon Barocas and Tim Hwang, Discriminating Tastes: Uber’s Customer Ratings as Vehicles for Workplace Discrimination, Policy & Internet Vol. 9, Iss. 3, June 28, 2017, https://onlinelibrary.wiley.com/doi/abs/10.1002/poi3.153; see also Caroline O’Donovan, That Four-Star Rating You Left Could Cost Your Uber Driver Her Job, Buzzfeed News, April 11, 2017, https://www.buzzfeednews.com/article/carolineodonovan/the-fault-in-five-stars#.yad03dx7O; Anya Kamenetz, Why Female Professors Get Lower Ratings, NPR, January 25, 2016, https://www.npr.org/sections/ed/2016/01/25/463846130/why-women-professors-get-lower-ratings.
1
For instance, reliance on tenure data could inadvertently pick up on sensitive signals like employees needing to leave to care for ailing family members or to accommodate spouses who needs to relocate for a new job (a sacrifice more often borne by women). See, e.g., Dina ElBoghdady, Why couples move for a man’s job, but not a woman’s, The Washington Post, November 28, 2014, https://www.washingtonpost.com/news/wonk/wp/2014/11/28/why-couples-move-for-a-mans-job-but-not-a-womans.
1
Ellis v. Google, available at http://altshulerberzon.com/wp-content/uploads/1.03.2018-First-Amended-Class-Action-Complaint.pdf (alleging that “[t]hroughout the Class Period, Google channeled women into lower paying job positions than men because of Google’s stereotypes about what men and women can or should do. For example, throughout the Class Period Google has channeled women (a) into lower paying Sales Brand Evangelist (aka Sales Solutions Senior Associate) jobs instead of higher paying Sales Representative jobs; (b) into lower paying Operations jobs instead of higher paying Engineer jobs; and (c) into lower paying Program Manager jobs instead of higher paying Technical Program Manager jobs on the basis of their gender. Google not only paid higher salaries to persons employed in jobs on Engineering ladders, but also paid more stock units and options to persons on Engineering ladders.”). Raises are often given when employees are promoted, and in the company’s early years, employees had to proactively apply for promotions. When the company realized that men requested to be promoted more frequently than women, it adjusted its practices, prompting more women to apply and a higher rate to be promoted. Cecilia Kang, Google data-mines its approach to promoting women, The Washington Post, April 2, 2014, https://www.washingtonpost.com/news/the-switch/wp/2014/04/02/google-data-mines-its-women-problem.
1
Ellis v. Google, id.
1
While women make up 48 percent of non-technical roles at Google, they make up only 21 percent of (often better-paying) technical roles. Nitasha Tiku, Bias Suit Could boost Pay, Open Promotions for Women at Google, Wired, September 14, 2017, https://www.wired.com/story/bias-suit-could-boost-pay-open-promotions-for-women-at-google/. A regulatory audit found “six to seven standard deviations between pay for men and women in nearly every job classification in 2015,” well exceeding evidence of statistical significance. Nitasha Tiku, Google Deliberately Confuses its Employees, Fed Says, July 25, 2017, https://www.wired.com/story/google-department-of-labor-gender-pay-lawsuit/. The cumulative effect of such practices is evident: while the company’s overall gender and racial diversity has improved somewhat since 2014, the company’s upper management remains overwhelmingly white and male. Google diversity annual report 2018, available at https://diversity.google/annual-report/.
1
For example, some companies are looking for patterns in employees’ communications in order to identify and predict top performers. Josh Bersin, What Emails Reveal About Your Performance At Work, October 12, 2018, https://joshbersin.com/2018/10/what-emails-reveal-about-your-performance-at-work/. Others are using “sociometric badges” and other sorts of physical tracking devices to monitor their employees. Thuy Ong, Amazon patents wristbands that track warehouse employees’ hands in real time, The Verge, February 1, 2018, https://www.theverge.com/2018/2/1/16958918/amazon-patents-trackable-wristband-warehouse-employees; There will be little privacy in the workplace of the future, The Economist, March 28, 2018, https://www.economist.com/special-report/2018/03/28/there-will-be-little-privacy-in-the-workplace-of-the-future. See also Ajunwa, supra note 90; Levy and Barocas, supra note 90.
1
For instance, “inclusive People Analytics” vendor Blendoor developed an index for employers to understand how various biases may be influencing their diversity and inclusion efforts. The index considers a variety of indicators, including whether companies track compensation by demographic, whether they have taken steps to reduce interpersonal bias in performance reviews, what sort of inclusive benefits (like maternity leave or flexible hours) the employer offers, and the proportion of promotions and managers who are diverse. A separate Blendoor Bias Index (BBI) also looks at indicators from earlier in the hiring process, like whether the employer tracks the progress of diverse applicants in their hiring pipeline, whether resume reviews are blind, and what percent of applicants, phone screens, interviewees, and hires are diverse. For all the indicators, see Blendoor, https://docsend.com/view/twcuxwz.
1
Equal Employment Opportunity Commission Meeting on Big Data in the Workplace, October 13, 2016, available at https://www.eeoc.gov/eeoc/meetings/10-13-16/transcript.cfm.
1
Kim, supra note 60.
Related Work
In this report, we investigate how large hourly employers are using technology to hire for low-wage hourly jobs. We scrutinize 15 online application processes, raise concerns with selection procedures like personality tests, and offer recommendations for employers and policymakers.
Labor and EmploymentWe urged the Subcommittee to ensure that hiring technologies are developed and used in ways that respect people’s civil rights, and offered recommendations concerning transparency and oversight.
Labor and EmploymentIn the Harvard Business Review, Miranda explains what we mean when we talk about “hiring algorithms” and why predictive hiring technology is far more likely to erode equity than it is to promote it.
Labor and EmploymentWe sent a memo on technology’s role in hiring discrimination to agency leaders within the Biden administration.
Labor and Employment